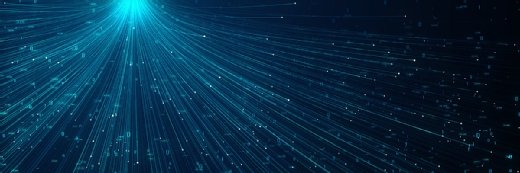
your123 - stock.adobe.com
What an automated data integration implementation means
Automated data integration can reduce time spent by data professionals on repetitive tasks. Learn about strategies to help implement automated data integration.
One of the core responsibilities for IT organizations is to partner with business units to fully leverage the potential of enterprise data, but achieving this goal is increasingly challenging. Instead of transforming data into actionable insights, many organizations are drowning in their own data.
Not only is the amount of data growing, but the number of application data stores creating the information is also expanding at a rapid pace. The more data and different types of data stores an IT shop must contend with, the greater these challenges become.
A 2020 IDC Global DataSphere forecast stated "the amount of data created over the next three years will be more than the data created over the past 30 years, and the world will create more than three times the data over the next five years than it did in the previous five."
When you add data generated by legacy systems, social media, mobile apps, IoT, database as a service (DBaaS) and IaaS, the challenge of combining and leveraging all this disparate data becomes painfully clear.
The ever-increasing growth of data and the wide array of applications generating it is compelling IT departments to find solutions that reduce the amount of time they spend collecting, storing, analyzing and presenting information to end-user communities.
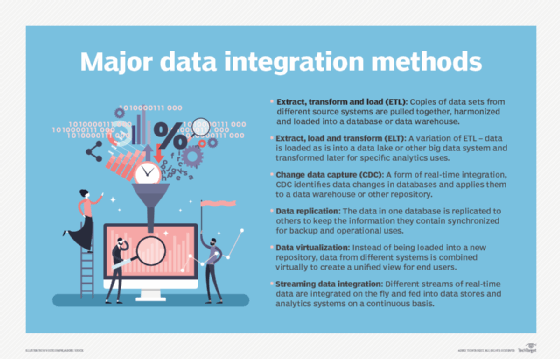
The benefits of automated data integration
To facilitate the analysis of information, many organizations turn to data marts, data warehouses and data lakes as their source platforms for business intelligence and enterprise reporting applications.
Data integration is the process of collecting the data from disparate source systems, then refining and formatting it before loading the information into the target platform. The industry acronym describing this process is ETL, for extract, transform and load.
A newer variation changes the sequence of the process to extract, load and transform, which refines and formats the data after it is loaded into the target data store.
Process automation has a wide range of applications -- but for data integration, the goal is to use automation and AI to reduce the human labor required to transfer data between the source and target systems.
Whenever there is a perceived need to automate a process, you'll find an enterprising set of vendors that provide solutions. From industry heavyweights such as Oracle, IBM, Informatica, SAS Institute Inc. and SAP, to smaller vendors that focus specifically on data integration, there is a wide and ever-growing array of offerings available.
Evaluating the automated data integration marketplace
Organizations interested in automated data integration platforms have a robust and somewhat bewildering set of products, technologies and features to evaluate. Below are several recommendations to help you jump-start your automated data integration product evaluation.
Follow a standardized product evaluation methodology to facilitate the selection process. Evaluation best practices include selecting the appropriate team, performing a thorough needs analysis and creating a robust set of weighted evaluation metrics. Use the evaluation metrics to create a vendor short list and execute a deep-dive comparison of the remaining vendors.
Visit the competing vendor websites to gain a better understanding of the features that are available. Add the additional features that are important to your evaluation matrix.
Understand and document your business needs by answering the following questions:
- Are you looking for a purpose-built platform that focuses specifically on data integration, or a general-purpose application suite that provides a much wider scope of features and functionality?
- Is the platform able to ingest a wide variety of data types from disparate source systems using industry-standard APIs?
- What data transformation capabilities does the product provide?
- What data governance, metadata management and data modeling capabilities does the product provide?
- Does the platform provide a robust UI for system and user administration and workload monitoring?
- Does the product support both batch and real-time processing? Real-time integrations are complex and require a detailed evaluation of how the system handles create, read, update and delete (CRUD) functions and data collisions.
- What workload management capabilities does it provide?
- Is the product able to conform to organizational, industry-specific or governmental regulatory requirements?
- How easy is it to scale the platform to accommodate increases in data volumes, source and target systems, and workloads?
Prevent budgetary surprises by thoroughly evaluating how the vendor will charge you. Cost models typically range from simple software purchases to cloud-based systems that charge by usage. To try to figure out costs, estimate initial and future workload volumes.
Also visit vendor, peer review and big data discussion forum websites. Gartner's Peer Reviews website is an excellent place to learn how the IT community rates various vendor products. Vendors often purchase distribution rights for Gartner's Magic Quadrants and make them available for the public to download.
Depending on your preference, you will need to identify if the product installation supports cloud, on-premises or both environments.