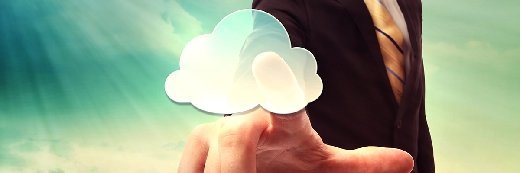
Melpomene - Fotolia
Machine learning approaches gain critical mass for data pros
Machine learning will bring change to analytics and data management, said data luminary Michael Stonebraker. Others agree managing such change will take special effort.
BOSTON -- It's not a futuristic technology anymore. Machine learning approaches are coming to mainstream data analytics and management.
The changes will come about as machine learning and related advanced analytics techniques surpass familiar BI approaches, and data professionals should prepare, according to noted database technologist Michael Stonebraker.
Speaking at Enterprise Data World 2019 here, Stonebraker advised data professionals to "start learning about machine learning packages -- because that is what you are going to be doing."
The effect of machine learning approaches will reach into many aspects of data management, he said in an EDW keynote presentation. The changes range from a full-scale effort to automate all aspects of database operations to new methods of data tagging based on patterns uncovered by machine learning programs.
Get out the algebra textbook
The machine learning charge is driven by greater data volume, velocity and variety, said Stonebraker, an adjunct professor at MIT who has played major roles in the development of such data management technologies and companies as Ingres, Illustra, Vertica, Tamr and Postgres, the predecessor to the PostgreSQL open source database.
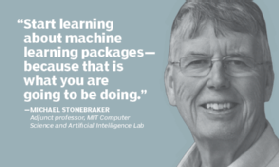
For data pros, learning about machine learning need not be entirely arduous, Stonebraker indicated. He advised them to "buy a linear algebra book," as it underlies much of what forms machine learning today.
In an interview after his presentation, Stonebraker expanded on that advice. He said much of the basic machine learning programming will be provided in packages created by software makers, but users will need to have some understanding of the principles behind machine learning predictions to successfully use the new technology.
Machine learning approaches at FINRA
The machine learning discussion continued at EDW in sessions such as one led by data analytics team leaders at FINRA, an independent financial industry regulatory authority based in Washington, D.C.
In a session entitled "Ushering in the Age of Machine Learning," Kristen Serafin, associate director of advanced analytics for market manipulation surveillance at FINRA, and Lizzie Westin, a lead data analyst there, described steps the organization has taken to bring machine learning to bear on what is big data by almost anyone's measure. FINRA, Serafin estimated, processes data on 135 billion financial market events per day.
She said FINRA has pursued machine learning within the context of research and development. Treating it experimentally was an important step, according to Serafin. While the authority abandoned some efforts, she said, other work turned into operational improvements at FINRA.
Serafin said some generally useful project practices apply with machine learning, too. Teams must understand the nature of the data in terms of such characteristics as volume and quality, and groups must gain skills to work with tools in the new technical environment, she told EDW attendees.
Meanwhile, getting users to accept the software, as always, is needed to achieve success. There, communication is key.
"You need to get buy-in first, and then turn that into sponsorship," Serafin said. "We did that through targeted training for business users."
The targeted training included stripping out a lot of technical language in presentations and focusing on real-world examples.
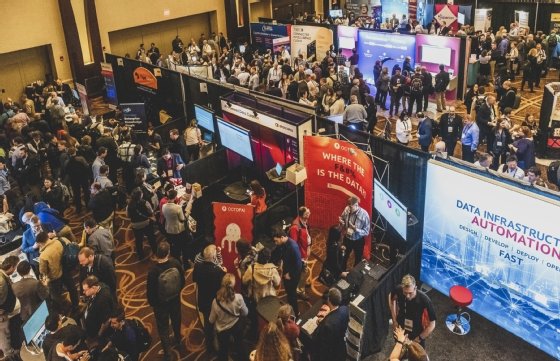
Unknown unknowns
Conference attendee Kumar Pillai marked machine learning as a primary area of interest just now. In his role as leader of global program management at job site Indeed.com, based in Austin, Texas, he deals with this with some caution.
In an interview, Pillai said traditional rule-based algorithms can still handle a large number of the use cases in organizations -- ones in which the patterns for analytics are already familiar.
Where machine learning approaches differ, he said, is in cases where "you don't know what you don't know." Such cases, Pillai continued, often are about discovering new patterns.
He said the kinds of tool packages that emerge for machine learning will ultimately dictate the strategies that companies pursue for the next generation of AI-oriented analytics.
"Machine learning, AI and big data -- this is all now pretty much at the height of the hype cycle, but many people don't know what the optimal uses are," Pillai said.