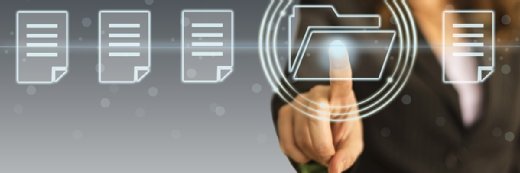
Getty Images/iStockphoto
Explore the evolving role of AI in the insurance industry
AI is transforming the insurance industry by automating processes and improving risk assessment, but it also poses challenges in data transparency and algorithmic decision-making.
AI's role in the insurance industry is growing. AI tools can ingest documents, assess risks, process claims, organize data and reduce fraud.
These applications of AI help insurance companies enhance efficiency and accuracy in evaluating risks and managing claims. However, AI technologies also introduce a variety of risks and challenges. Insurance companies must tread carefully to mitigate, detect and manage hallucinations and bias as they implement AI tools.
When issues like algorithmic decision-making and data transparency are properly addressed, AI can offer significant benefits to the insurance industry.
AI use cases in the insurance industry
AI is used across the insurance industry for tasks like underwriting, risk quantification and fraud detection. Here are some specific ways insurance providers are incorporating AI into their workflows.
Streamlining contact centers
Chris Raimondo, insurance technology leader at EY Americas, worked with a personal lines insurance carrier that integrated generative AI into its customer contact center. This AI tool automatically summarized call notes, improving accuracy and standardization in record-keeping. It also enhanced future customer support by providing agents with clear case histories, enabling them to offer more effective and individualized support.
Ingesting paper documents
Despite considerable digitization efforts, the insurance industry still processes many paper documents.
"Most insurers still deal with an insane amount of paper applications, payments and claims notes, and much of the content or data on those documents is handwritten," said Franklin Manchester, a global insurance strategic advisor at SAS. Manually reviewing documents takes time and effort, but AI can perform that analysis at scale much faster than a person can.
Predicting and quantifying risks
AI can also help insurance carriers predict and quantify risks with greater accuracy. Suhas Sethi, global business leader of the insurance practice at professional services firm Genpact, explained that AI can reduce property-casualty insurers' claims costs, which are rising due to inflation, supply chain issues and extreme weather events.
"By integrating AI powered by high-quality data from claims processes, insurers can more accurately predict the impact of natural catastrophes, enhancing exposure management and catastrophe modeling," Sethi said.
This enables insurers to choose risks and price policies more accurately. In the event of large claims, using generative AI tools can help insurers respond to claimants more quickly and ultimately manage the aftermath of catastrophes more effectively.
Muddu Sudhakar, cofounder and CEO of generative AI platform Aisera, said that insurance companies are also using generative AI to improve risk assessment. By analyzing demographic data and creating simulations, generative AI can identify potential claims and aid coders in developing statistical models. This approach can save insurers time and money in the long run.
Internal virtual assistants
Kjell Carlsson, head of AI strategy at Domino Data Labs, said the most common production use case among Domino customers in the insurance industry is internal virtual assistants. These AI-powered assistants can provide employees with tailored information on a customer's insurance policy, giving employees better context when answering customers' questions.
The internal aspect is key. Employees are better positioned to push back and question hallucinations than customers and can provide valuable feedback in the early stages.
"It is a particularly successful use case because it is low risk, delivers value quickly and plays to the strengths of LLMs," Carlsson said.
Data preparation
Gene Linetsky, CTO at business insurance service Embroker, has been exploring the potential for AI to improve extract, transform and load (ETL) processes using large language models (LLMs).
"Traditionally, ETL across multiple sources is expensive and time-consuming because of the needed custom-coded schema conversions," he said.
Recent advancements in LLMs promise to significantly improve ETL processes. LLMs excel in meaning-mapping, translating data fields among sets at a fraction of the cost and time required for configuring ETL adapters. Linetsky believes this will help insurers create fully autonomous data pipelines to process vast, highly diverse data streams; extract actionable insights; and integrate those insights into marketing, underwriting and client experience workflows.
Usage-based insurance
Joel Pepera, director of telematics product development at transportation analytics platform Arity, previously played a key role in Geico's usage-based insurance practice. Usage-based insurance relies on AI and machine learning to develop insights into individual driving behaviors from sensor data generated by smartphones and other IoT devices.
Based on these insights, insurance carriers argue, they can price customers more accurately and fairly, focusing on their unique risk profiles rather than demographic factors. However, the practice has also led to pushback from drivers concerned about their data privacy.
Automating appraisals
Insurance companies are increasingly turning to AI to improve damage assessment based on claimants' submitted photos. Nicos Vekiarides, founder and CEO of deepfake detection platform Attestiv, said AI can help assess damages and settle claims through self-service processes for policyholders.
This, in turn, increases the volume of claims insurers can settle and lets claims experts focus on more complex scenarios that demand their attention. AI can train on millions of photos of settled claims to gain the equivalent of decades of experience much faster than a human adjuster.
"Of course, that does not negate the need for adjusters, as checks and balances are often necessary to ensure the AI models are not making errors," Vekiarides said. "But this type of hybrid verification can be much faster than settling all claims manually."
Automating fraud detection
AI can also be essential in managing insurance fraud, Vekiarides said. Traditionally, efforts to root out fraud have required considerable human inspection and analysis. Most insurance providers, however, do not have sufficient resources for such initiatives, given the relatively small size of the typical special investigations team. Consequently, although a good deal of fraud is caught, much can still slip through.
"It makes a lot of sense for insurers to analyze claims photos, videos, documents and circumstances using AI to spot anomalies for further review by investigation teams," Vekiarides said.
However, he also emphasized the importance of checks and balances. Human input is necessary to guard against false positives and to take proactive action, such as deploying adjusters or investigators to verify the results of AI analysis.
Improving claims processes
Sarah Bratschun, senior scientist at agricultural risk assessment platform Ceres Imaging, said AI can help reduce data fragmentation in claims processes. Her company addresses this problem in agriculture, where data used for risk management -- such as government databases, sensor readings, farm reports and satellite imaging -- is often fragmented and isolated.
AI helps integrate and standardize this data to better account for soil and crop health differences, including yield potential influenced by factors like soil type, climate and agricultural practices. Improved data enhances risk management and provides growers with reliable access to credit and fair, quick compensation for crop losses or failures. This empowers growers to make better-informed decisions, prioritizing their land's long-term health and productivity over short-term gains.
On the insurance side, reducing data fragmentation improves processes across the board. AI can extrapolate plant- and field-level data before and after harsh weather events to quickly and accurately determine, analyze and visualize the impacted area. It also reduces claim resolution turnaround time, enhancing customer experience.
Improving underwriting accuracy
AI also enables insurance carriers to create products and policies that better fit their customers' needs.
In agriculture, for example, AI plays an important role in modeling farmland data to accurately define productive and non-productive elements by classifying the land effectively, Bratschun said. Growers benefit from faster and more accurate claims determinations, and a correct understanding of their risk enables them to make informed decisions about crop selection and resource allocation.
At the portfolio level, lenders can better understand and rebalance risk or tailor loan terms and pricing to match the specific conditions of their policyholders. Land investors, meanwhile, can proactively manage the risk of their holdings through diversification.
EY's Raimondo also worked with a multi-line insurance carrier that used generative AI to consolidate diverse, unstructured data sources into a unified system for underwriters and service center resources. This AI implementation reduced time-consuming manual research, enabling teams to get comprehensive answers to underwriting and quoting questions more quickly.
Challenges of AI in the insurance industry
Insurance companies face the same AI challenges as many other industries, including trust, alignment, bias and hallucinations. In particular, the insurance industry is struggling with how to handle algorithmic decision-making and data transparency in AI adoption.
Algorithmic decision-making
AI algorithms can learn the patterns of human experts' decision-making, enabling them to automate the process of making similar choices in the future. However, these models can suffer from hallucination or bias, leading managers to impose incorrect decisions on experts who should be making the calls.
For example, UnitedHealth, one of the largest health insurance providers in the U.S., was criticized for imposing algorithmic decision-making on doctors. The company faced a lawsuit alleging that its algorithms systematically denied elderly patients' needs for extended care against doctors' advice.
Insurance providers looking to scale algorithmic decision-making shouldn't do so at the expense of experts familiar with a particular field. While faster than human decision-making, algorithmic decision-making needs oversight, especially when it fails to align with the judgment of human experts.
Data transparency
Insurance companies have a constantly increasing data foundation for estimating risks and pricing products. However, opportunities to use data must be balanced with customer interests.
For instance, the New York Times reported earlier this year that many new GM smart car data service subscribers discovered that their insurance rates went up significantly after data about their mileage, braking and acceleration was shared with hundreds of third parties. These included LexisNexis, a global data broker that compiles risk reports for insurance agencies.
Due to backlash, GM relented and announced the company would no longer share such personal data. However, the insurance companies that raised rates have not yet made similar promises. Building trust with customers requires transparency about what data goes into training algorithms. When companies acknowledge this, customers can make better decisions -- not only for insurance purposes, but also for road safety.
It's important to provide end-user transparency and obtain consent for what data is collected and how it is used, Arity's Pepera said. Investing in the infrastructure required to manage large data sets, train AI models, and maintain a monitoring and governance framework to track performance over time is also critical.
George Lawton is a journalist based in London. Over the last 30 years, he has written more than 3,000 stories about computers, communications, knowledge management, business, health and other areas that interest him.