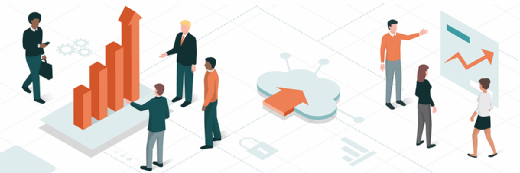
How to choose the right autoML platform for your enterprise
Before autoML can improve model building and deployment, enterprises need to choose a platform. Here, we evaluate autoML platforms by category, key features and accessibility.
Automated machine learning, or autoML, is one of the newest tools in AI, and it's being used to remove barriers to entry that hold back enterprises' machine learning ambitions. The market demanded user-friendly machine learning that could be operated by employees with a limited background in data science or improved by trained data analysts. With autoML, flexibility is key.
The idea behind autoML is relatively straightforward: Automate the machine learning process and strive to make it more enterprise user-friendly, with faster outputs. Companies with data science teams can create their own platforms, tweak algorithms and parameters, and cobble together autoML tools, while those with limited data science teams can use an autoML platform to still get the desired outcome.
With all the competition around vendor platforms, open source tools and data scientist requirements, experts are encouraging the slow and steady approach: Evaluate your problem or need, evaluate individual tools and providers, then choose the tools that most closely address your problems.
Top platforms by category
In evaluating autoML tools, enterprises often first look to popular vendor platforms. But experts warn the decision isn't so clear. There are a variety of platforms and tools that differ widely based on need.
Carlie Idoine, senior director and analyst of data science and business analytics at Gartner, divides autoML platforms into four distinct categories.
- Build your own. In this approach, an enterprise's data science teams build platforms themselves. This approach allows them to control parameters, tuning, machine learning operations and model assessment through individual open source or proprietary code. This type of autoML platform allows for hyperpersonalized augmentation -- you can have SigOpt for parameter tuning and Algorithmia or ParallelM for MLOps.
- Commercial platforms. The commercial platforms category adds some augmented AI capability, but it's an extension of a traditional machine learning platform with autoML capabilities. Commercial platforms give you more breadth and depth of capability, with features to support collaboration between experts and nonexperts. Popular products include Amazon SageMaker, RapidMiner, Alteryx and other large platforms.
- Augmented platforms. These platforms encourage citizen data scientists to build and deploy their own machine learning applications. These platforms are typically built to address specific problems. However, because these platforms are less customizable, they can be limited in the range of problems they can tackle. Some of the leaders in this platform category are DataRobot, Aible, Big Squid and Tazi.
- Cloud machine learning services. These platforms are essentially APIs geared toward application developers and people who want to integrate AI into existing applications. These APIs are purpose-built and tend to focus on specific tasks, like computer vision, language processing and translation. Some top vendors in this category are Amazon Web Services, Google Cloud and Microsoft Azure.
Build vs. buy
When choosing whether to build or buy, examining your data science team, its capabilities and ultimate goals is a major factor to consider. AutoML is an extension of your current machine learning strategy, not a replacement; expert data scientists should still be involved in overseeing and validating the models.
If your data science team is small and wants to offload some of the model building work onto a platform vendor, then choosing a commercial or augmented platform is a viable option. Enterprises choosing this option must evaluate vendors by whether the tools can address their specific machine learning goals.
"There are a lot of service providers that are primarily doing the grunt work by cobbling together a suite of open source packages," said Evan Schnidman, CEO of natural language processing company Prattle, based in St. Louis. "If you're going to work with a service provider, asking what tools they're using is just as important as going out and figuring out what tools you can use in-house."
Building your own in-house tools is a feat left to companies with data scientists and an evolved AI strategy. Choosing your own parameters and personalized model building is not always a simple -- or quick -- entry point into machine learning.
Idoine also said companies should first evaluate the outcome they're seeking from autoML platforms, rather than consider autoML as one broad category. There are separate platforms geared toward different stages of the model-building workflow, including augmented data preparations, augmented analytics and BI, and augmented data science and model building.
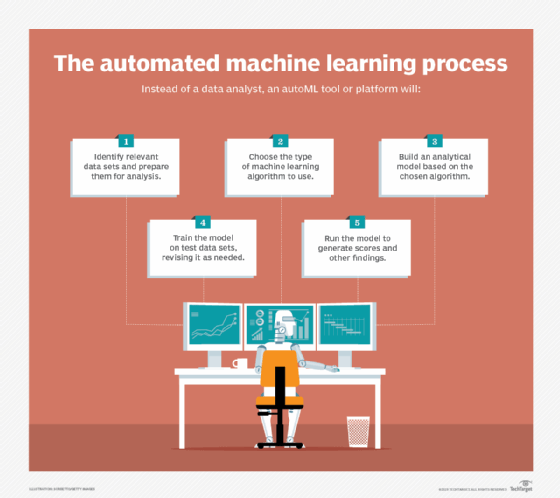
Key features
Whether you build or buy, or need help with data prep or model deployment, autoML platforms have a few key features you should evaluate. Analyze platform capabilities around their pattern modeling and automation specificity, and match them to your current needs.
For some enterprise users, autoML platforms are simply an entrance point into machine learning and AI that doesn't require a heavy investment in data scientists. If enterprises simply need a place to begin -- and begin soon -- Iodine and Schnidman recommend the citizen data scientists platform above.
"A benefit to the citizen data scientist platform is that it's expressly for citizen users, so it's easier to get going faster," Idoine said.
For enterprises that are looking to augment their data scientists' work, speed and streamlined capabilities are some of the most important features of an autoML platform, Schnidman said.
"The vast majority of time in the data science world is still being spent on data engineering and data washing," Schnidman said. "So, the first range of tools is all about how you streamline the data ingestion and data washing process. The next range of the tools is how you streamline model development and model deployment, and then the third range is how you streamline model testing and validation."
For enterprises that already have a team of data scientists, experts encourage collaboration between the users of autoML tools and those who will oversee the machine learning process. Like any AI implementation, the platform and tool choice should be focused on augmenting capabilities, not replacing workers.
"Increasingly, data scientists are saying, 'I want to use these tools, because they help me work more efficiently, they make my process faster and [can] also do things like test bias or eliminate bias,'" Iodine said.