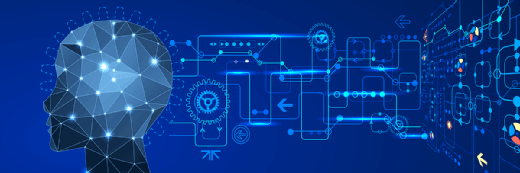
Tackling the AI bias problem at the origin: Training data
Though data bias may seem like a back-end issue, the enterprise implications of an AI software using biased data can derail model implementation.
Ethical AI is a top priority for business leaders, but given all the hype about AI, it's easy for non-data scientists to focus on potential opportunities without giving adequate thought to its risks. As the use of AI continues to expand, organizations need to shift focus to ensure trustworthy, ethical outcomes.
Organizations are increasingly becoming responsible for harm reduction in their software -- in their own development, or through software. Algorithmic (data) bias is one of the main drivers of ethical AI discussions because the presence of bias in AI systems negatively impacts consumers, customers and brands.
As data scientists explore data bias affecting real-world AI applications, it's never been timelier to solve the AI bias problem.
Types of bias
AI bias comes in many different forms. Some are human cognitive biases and others are data-related biases. Some of the most common forms are not necessarily mutually exclusive -- enterprises need to carefully examine internal and external biases in their data sets as well as their AI applications.
- Confirmation bias is a human cognitive bias. It involves selecting and analyzing data in a way that reflects a pre-existing point of view (proving one's own correctness).
- Confounding variable is a variable that influences both the dependent variable and independent variable. Essentially, it's a variable that impacts the outcome which was not taken into account.
- Overfitting is an analysis that's too closely tied to a specific data set. A classic example is a predictive model that performs well on the original training data, but its accuracy level falters when new data is introduced.
- Sample bias is a sample which does not represent the population it's supposed to represent.
- Selection bias is cherry-picking data for analysis or inadvertently choosing a non-representative sample (which is more likely).
- Simpson's paradox occurs when a trend disappears or reverses at different levels of aggregation. For example, a trend may be present when several groups are analyzed separately, but the trend may not exist or may be the opposite when the groups are combined and analyzed as a single population.
- Skew is an asymmetric probability distribution that can be but is not necessarily caused by outliers.
Data bias influences enterprise initiatives
Though data bias may seem like a back-end issue, the enterprise implications of an AI software using biased data can derail diversity and HR initiatives, reduce ROI and create consumer distrust.
What's now referred to as the "white guy problem" -- data's inherent biases based on developers and training sets -- has taken several forms. There's algorithms confusion around non-white faces, ethnic or racial discrimination, unhelpful generalizations, etc. In consumer-facing applications, these biases cause over-policing, sweeping generalizations, incorrect predictive analytics and financial prejudice.
"One of the biggest [bias] issues is data collection -- how I'm going to collect the data, what data sources I'm using, how big the sample is, whether the sample is representative or not," said Dan Simion, Capgemini North America's VP of AI and analytics. "These are the main reasons you have data that's skewed."
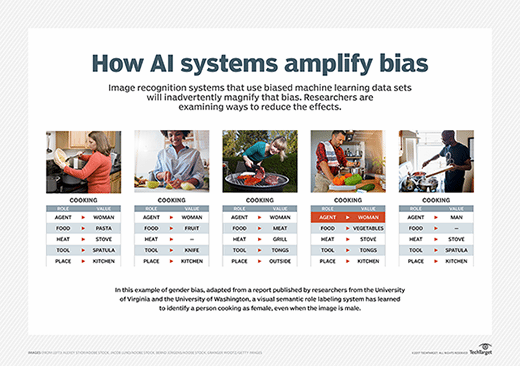
John Frownfelter, chief medical information officer at clinical AI company Jvion, said the conclusions drawn from a blood pressure study of white male veterans were generalized to other populations including women and people of color and applied to medical decisions. This skewed data negatively influenced their consumer knowledge.
"One of the most common forms of bias that we try to eliminate today is starting with the wrong population. I think that's pretty well understood and mitigated, but you may still have blind spots and you may not know what they are," said Frownfelter.
An example of that is concluding that an affluent population is less healthy than a poor population simply because there's more data available on the patients who can afford medical treatment. Sometimes data, such as personally identifiable information (PII) is omitted from an analysis by law or to reduce a risk. However, it may be possible to infer the same information using other data.
"When you're bringing in people and business problems, you're bringing in: What are the implications of this algorithm? How is this algorithm going to be used?" said Meredith Butterfield, principal data scientist at data science consulting firm Valkyrie.
Addressing the data bias problem
Bias needs to be identified before it can be addressed. One way is to see whether an outcome is having a disproportionate impact on one or more subsets of a population. Another is to consider the historical biases an organization has had and see how that's reflected in the data. It's also possible to use a supervisory AI to identify potential areas of bias.
However, even if bias has been eliminated, models need to be continuously monitored for drift.
"We have to continually monitor whether a model is becoming more or less biased over time and we can only do that if we understand the relationships the model is learning. At model-building time, we make sure it's not biased towards a particular class it shouldn't be showing bias towards," said Scott Zoldi, chief analytics officer at data analytics company FICO. "And in production, we continue to monitor latent features in the models to make sure that the data isn't skewing towards a protected class."
As part of the monitoring, FICO proactively sets thresholds so when the distributions change it's clear that a model should not be used for all customers or a subset of customers.
Valkyrie's Butterfield said if she were to build a model predicting the success of companies that have a CEO other than a white male, she might look at how the algorithm was performing comparably for male and female CEOS as well as people of color. If the model is performing differently for the different groups, then there's probably bias in the model, so either the model needs to be altered or different models need to be created for the different groups.
Ethical AI: From principles to practice
More organizations have articulated ethical AI principles and values with the goal of implementing "trustworthy AI" or "responsible AI," but some of them are having difficulty translating those concepts into something that can be implemented in practice. Central to every set of AI principles and values are fairness and explainability, but those buzzwords become convoluted when data scientists and IT pros are stuck in a black box.
"We have the three Es: explainable AI so I understand what drives my model, ethical AI where we take those features that are ethical and unbiased, and then efficient AI which is around being able to remediate or work with the model in a changing environment," said FICO's Zoldi.
Similarly, when Capgemini builds models, they're designed and trained to ensure fair treatment of all customer groups. Simion said Capgemini also considers traceability important so the models can be audited from an ethical perspective and the organization can ensure that the outcomes are trustworthy.
"I think there's a long journey ahead because companies need to do much more work on explaining the AI and not look at AI like it's something magic or a black box," said Simion.