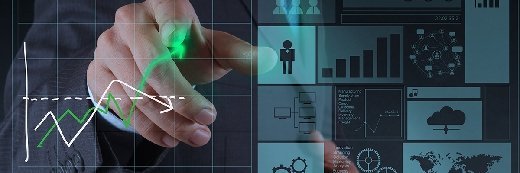
everythingpossible - Fotolia
Synthetic data could ease the burden of training data for AI models
Sometimes it's better to manufacture training data for machine learning models than it is to collect it.
Machine learning models are gluttonous. They need to consume a lot of training data -- and the right training data -- if they're going to work properly. In fact, one of the hurdles to capitalizing on machine learning technology is collecting enough data to satisfy the models.
But new techniques could ease that burden, according to David Schatsky, managing director at Deloitte LLP, and Rameeta Chauhan, a senior analyst at the firm.
In research published in November, Schatsky and Chauhan cited reducing the need for training data as one of five areas of progress in machine learning that will lower the barrier of entry for the enterprise. One method to get enough data is to use synthetic data or artificially manufactured data that looks and acts enough like real-world data to train AI models effectively.
Synthetic data can be valuable in situations where data is restricted, sensitive or subject to regulatory compliance, said Schatsky, who specializes in emerging technology. And it can advance projects that are hindered by a too-arduous process of acquiring the necessary training data.
Synthetic data use cases
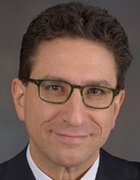
Indeed, one of the first synthetic data examples Schatsky encountered was for computer vision, technology that enables machines to recognize faces or identify objects in digital photos. Researchers today are building sophisticated computer vision features where the technology can follow an eye gaze or detect an emotion on someone's face. But gathering the amount of data needed -- and labeling it -- is laborious.
"And, so, what researchers did is they took a 3D-digital model of a human face and then manipulated it," Schatsky said. They can generate as many permutations of facial expressions or eye positions as they want -- and they can do so "quickly and cheaply, compared to collecting a comparable number of images," he said.
Another synthetic data use case is training robots to perform complex and agile tasks such as picking up or manipulating objects of different shapes and sizes, which is a big challenge for roboticists. "One approach is to generate an initial training data set by having a human being demonstrate what they want done -- in virtual reality," Schatsky said.
The human model moves a hand, picks up an object and puts it down. The entire set of actions is captured digitally, which means the images can be easily manipulated. "The digital model of that behavior can be rerendered in countless ways -- with different backgrounds or at different angles and so forth -- without having a human do it a thousand times," he said.
Synthetic data a boon to crowdsourcing?
Synthetic data could give companies with sensitive data a chance to tap into third-party data science help. To use crowdsourcing competition platforms such as Kaggle, companies have to publish data sets. This bars companies with sensitive data from taking advantage of these crowdsourcing platforms. However, creating a "ghost of the privileged data" that shares similar characteristics for data science competitions could effectively anonymize sensitive data sets, Schatsky said, giving data scientists a chance to develop a model that works on the manufactured data. "And if it's done right, that model will also work on the real data without having to share the real data," he said.
Synthetic data can also be generated mathematically. Schatsky said data scientists can take a small set of real-world data and perform a statistical analysis to develop a kind of profile of the data. If the data set had a thousand variables, its profile might include things like the coincidence of the variables or the distribution of the frequency of the variables. Based on the profile, the data scientists generate a set of synthetic data statistically that has as a similar profile.
A work in progress
Synthetic data isn't a relevant solution in every scenario, according to Schatsky. "For instance, if you are a financial services company that has lots of historical data about transactions and good records about which ones turned out to be fraudulent, then you have all the data; you understand which ones were fraudulent transactions, so the labeling is already done for you,"' he said. "So, the value of reducing training data is not as high in that situation."
Nor should CIOs buy into the idea without skepticism. Deloitte, in fact, took an experimental approach to synthetic data when it was doing work for a client. The consultancy built a model for an application "in the conventional way," Schatsky said. "And then we used this technique of generating synthetic data just to see if we could have done this same work with less training data."
It turned out they could. Using 20% of the training data to generate the synthetic data, Deloitte got the same results produced by the conventional mode.
Still, he said, while synthetic data worked well in this case, it might not work in other scenarios and it would be wrong to think of it as a panacea. "I don't know that I can give you a bright line describing exactly where this falls short," he said. "I bring it out in our research just to say that it's an important area of development that should definitely be considered in certain circumstances. But the effectiveness of it needs to be verified experimentally."