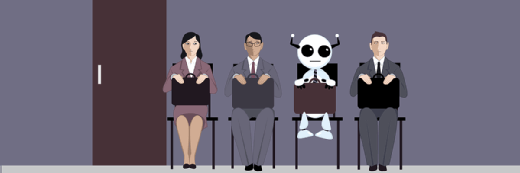
AI in hiring: Why HR should beware of bias
AI in hiring may seem like the perfect solution to remove conscious and unconscious biases that already exist in people, but technology can't solve everything.
The use of AI in hiring has grown, but the technology has a potential danger -- bias.
Both unconscious and conscious biases are reflected in recruiters' and HR teams' hiring decisions, but they may not understand the biases that are built into advanced technology.
"There are many places where algorithmic decisions around human resources don't neatly map onto accepted ways of thinking about workplace decisions," said Peter Capelli, professor of management at the Wharton School and director of its Center for Human Resources. "But in terms of actual bias -- that is, the algorithms generating outcomes that are 'wrong' in some standard way -- the answer has to do with the data on which the algorithms are based."
AI's algorithms can reflect programmers' views and the biases inherent in workforce composition, said Mark Kerzner, cofounder of Elephant Scale, a Houston company that offers training programs in big data and data science.
"If we just feed [the workforce composition] into the program, the program becomes prejudiced," Kerzner said.
Consequently, HR staffers should have at least a basic understanding of AI-powered systems -- for example, by knowing which data underpins the algorithms.
How to create unbiased AI
AI in hiring should be based on a good data set.
"If you don't have a robust, diverse set of data for your AI to look through, it's going to skew the results to a certain area," said Bhushan Sethi, a partner at PwC and joint global leader of its people and organization practice.
It's important for employers to make sure AI tools aren't reflecting bias against certain demographics, he said. That involves processes as much as technology.
"When we talk about AI in recruiting, it's the data set, but it's also [whether you have] the right kind of business requests and the right controls in place," Sethi said.
For example, if employers define a role's requirements too narrowly, their system will likely skew results too narrowly as well. Recruiting models that use machine learning typically try to make the closest match between a job's desired outcome and a candidate's history. Those job requirements could include performance measures that, for example, favor men or don't account for women who've been excluded from positions that receive the highest performance scores. In that case, the algorithms used to scan for the "right" candidates will also be biased. In those cases, an algorithm might find women are less well-matched to the employer's desired results.
"That means when we are generating scores to measure how well a candidate fits the profile of successful hires, women … will get lower scores," Capelli said.
To compensate for that, employers need to be aware of how everything from the job descriptions they write to the databases they search can include bias.
The degree to which AI in hiring is useful is influenced by a range of things beyond the data set, Sethi said. The wording of job requirements and the human controls -- or lack of them -- placed on the back end are just two examples.
It's up to people to ask whether the AI has generated results consistent with business goals, such as wanting to be diverse, Sethi said.
Employ AI deliberately
In many companies, recruiters and HR teams are just beginning to use AI in hiring, and they aren't aware of its potential for bias.
"Recruiters haven't made that connection, as yet," Kerzner said. "They read about the question of 'will a robot take my job away?' So they've heard about this idea that AI may be unfair, but it will be some time before they realize there's a connection between what they do in their models and the fact that there is this discussion."
For their part, some technology providers that use AI in hiring have begun to educate customers on the possibility of unintended consequences.
That education often develops around how the technology's decisions need verification, how its data sets need to be properly governed and how job requirements must align with other elements of an organization's diversity strategy, Sethi said.
Employers should use AI in hiring intentionally, he said. They should also search for candidates in a variety of areas, seek people who are outside their industry and look beyond an established set of peer companies.
"They have to juxtapose [AI] against some broad criteria to ask, 'are we being expansive enough?' especially with the really hard-to-fill roles," Sethi said.