AI hiring bias: Everything you need to know
Bias and discrimination can creep into your hiring process in all sorts of places. Here are the ones to watch out for, and tips on mitigating their negative effects.
AI hiring tools can automate almost every step of the recruiting and hiring process, dramatically reducing the burden on HR teams.
But they can also amplify innate bias against protected groups that may be underrepresented in the company. Bias in hiring can also reduce the pool of available candidates and put companies at risk of not complying with government regulations.
Amazon was an early pioneer in using AI to improve its hiring process in 2018. The company made a concerted effort to weed out information about protected groups, but despite its best efforts, Amazon decided to terminate the program after discovering bias in hiring recommendations.
What is AI bias and how can it impact hiring and recruitment?
AI bias denotes any way that AI and data analytics tools can perpetuate or amplify bias. This can take many forms, the most common being increasing the ratio of a particular sex, race, religion or sexual orientation. Another form may be differences in hiring offers that increase disparities of income across different groups. The most common form of bias in AI comes from the historical data used to train the algorithms. Even when teams make efforts to ignore specific differences, AI inadvertently gets trained on biases hidden in the data.
"Every system that involves human beings is biased in some way, because as a species we are inherently biased," said Donncha Carroll, a partner in the revenue growth practice of Axiom Consulting Partners who leads its data science center of excellence. "We make decisions based on what we have seen, what we have observed and how we perceive the world to be. Algorithms are trained on data and if human judgment is captured in the historical record, then there is a very real risk that bias will be enshrined in the algorithm itself."
This article is part of
Ultimate guide to recruitment and talent acquisition
Bias can also be introduced in how the algorithm is designed, and how people interpret AI outputs. It can also arise from including data elements such as income that are associated with biased outcomes or insufficient data on successful members of protected groups.
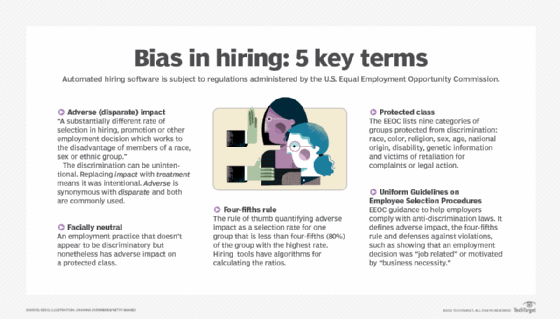
Examples of AI bias in hiring
Bias can get introduced in the sourcing, screening, selection and offer phases of the hiring pipeline.
In the sourcing stage, hiring teams may use AI to decide where and how to post job announcements. Various sites may cater more to different groups than others, which can add bias to the pool of potential candidates. AI may also recommend different ways to word job offers that may encourage or discourage different groups. If a sourcing app notices that recruiters reach out to candidates from some platforms more than others, it may favor placing more ads on those sites, which could amplify recruiter bias. Teams may also inadvertently include coded language, like "ambitious" or "confident leader," that has greater appeal to privileged groups.
In the screening phase, resume analytics or chat applications may use certain information to weed out potential candidates that may also be indirectly associated with a protected class. They may look for characteristics the algorithm associates with productivity or success, such as gaps between jobs. Other tools may analyze how candidates perform in a video interview, which may amplify bias toward specific cultural groups. Examples include sports that are more often played by particular sexes and races or extracurricular activities more often undertaken by the wealthy.
In the selection phase, AI algorithms might recommend some candidates over others by using similarly biased metrics.
Once a candidate has been selected, the firm needs to make a hiring offer. AI algorithms can analyze a candidate's previous job roles to make an offer the candidate is likely to accept. These tools can amplify existing disparities in starting and career salaries across gender, racial and other differences.
Government and legal concerns
Government organizations like the U.S. Equal Employment Opportunity Commission (EEOC) have traditionally focused on penalizing deliberate bias under civil rights laws. Recent EEOC statistics suggest that the jobless rate is significantly higher for Blacks, Asians and Hispanics compared to whites. There are also significantly more long-term unemployed job seekers age 55 and over compared to younger ones.
Last year the EEOC and the U.S. Department of Justice published new guidance on steps companies can take to mitigate AI-based hiring bias. The guidance suggested enterprises look for ways that AI could be taught to disregard variables that have no bearing on job performance. It is also important to recognize that AI can still create issues even when precautions are in place. These mitigation steps are important because enterprises face legal ramifications from using AI to make hiring decisions without oversight.
EEOC chair Charlotte Burrows recommends that enterprises challenge, audit and ask questions as part of the process of validating AI hiring software.
Four ways to mitigate AI bias in recruiting
Here are some of the key ways organizations can mitigate AI bias in their recruiting and hiring practices.
1. Keep humans in the loop
Pratik Agrawal, a partner in the analytics practice of Kearney, a global strategy and management consulting firm, recommended firms include humans in the loop rather than relying exclusively on an automated AI engine. For example, hiring teams may want to create a process to balance the different categories of resumes that are fed into the AI engine. It's also important to introduce a manual review of recommendations. "Constantly introduce the AI engine to more varied categories of resumes to ensure bias does not get introduced due to lack of oversight of the data being fed," Agrawal said.
2. Leave out biased data elements
It is important to identify data elements that bring inherent bias into the model. "This is not a trivial task and should be carefully considered in selecting the data and features that will be included in the AI model," said Carroll. When considering adding a data point, ask yourself if there is a tendency for that pattern to be more or less prominent in a protected class or type of employee that should have nothing to do with performance. Just because chess players make good programmers does not mean that non-chess players couldn't have equally valuable programming talent.
3. Emphasize protected groups
Make sure to weight the representation of protected groups that may currently be underrepresented on the staff. "This will help avoid encoding patterns in the AI model that institutionalizes what you have done before," Carroll said. He worked with one client who assumed a degree was an essential indicator of success. After removing this constraint, the client discovered that non-degreed employees not only performed better but stayed longer.
4. Quantify success
Take the time to discern what qualifies as success in each role and develop outcomes such as higher output or less rework that are less subject to human bias. A multivariate approach can help remove bias that might be ingrained in measures like performance ratings. This new lens can help to tease apart traits to look for when hiring new candidates.