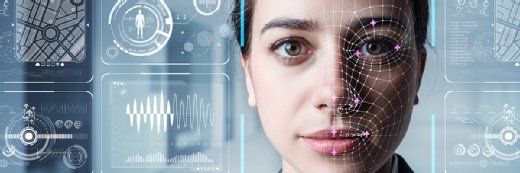
metamorworks - stock.adobe.com
Why algorithmic auditing can't fully cope with AI bias in hiring
Critics say you can't trust AI yet with diversity and inclusion in hiring -- or the elaborate audits meant to root out AI bias. Hiring software vendors beg to differ.
AI is often touted as an antidote to bias and discrimination in hiring. But there is growing recognition that AI itself can be biased, putting companies that use algorithms to drive hiring decisions at legal risk.
The challenge now for executives and HR managers is figuring out how to spot and eradicate racial bias, sexism and other forms of discrimination in AI -- a complex technology few laypeople can begin to understand.
Algorithmic auditing, a process for verifying that decision-making algorithms produce the expected outcomes without violating legal or ethical parameters, is emerging as the most likely fix. But algorithmic auditing is so new, lacking in standards and subject to vendor influence that it has come under attack from academics and policy advocates. Vendors are already squabbling over whose audits are the most credible.
That raises a big question: How can companies trust AI if they can't trust the process for auditing it?
Why is algorithmic auditing important?
Before delving into answers, it's important to understand the goals companies are trying to achieve by applying algorithmic auditing to automated hiring software.
Many companies strive to include people of color and other minorities in their workforces, a goal that grew more urgent with the rise of diversity programs and the Black Lives Matter movement. Ultimately, they don't have much choice: Discrimination has been illegal for years in most jurisdictions around the world.
In the U.S., the 1964 Civil Rights Act prohibited employment policies that have a disparate impact and adversely affect members of a protected class (Figure 1). The Uniform Guidelines on Employee Selection Procedures, part of a 1991 revision of the Civil Rights Act, quantified what disparate impact (also commonly called adverse impact) meant. The guidelines include a four-fifths rule that requires the selection rate of any race, sex or ethnic group to be no less than four-fifths (80%) of the group with the highest rate.
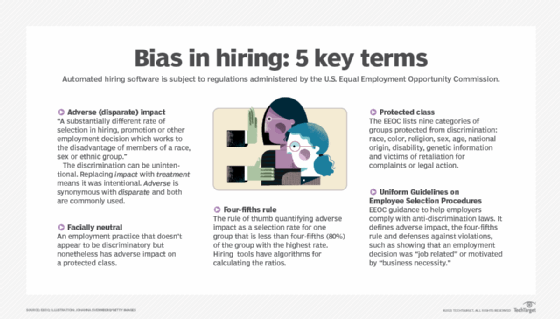
Companies have been operating under these rules, which are administered by the federal Equal Employment Opportunity Commission (EEOC), for half a century overall. But it's only in the past couple of decades that they've had recruitment and talent management software to help with employment decisions -- such as hiring, promotion and career development -- that expose them to disparate impact challenges. With AI now automating more data analysis and decision-making, organizations are anxious to know whether the technology is helping or hurting.
But investigating the algorithms used in hiring is complicated, according to Alex Engler, a fellow at The Brookings Institution, a Washington, D.C., think tank. Engler is a data scientist who has sharply criticized AI-based hiring tools and algorithmic auditing.
"You're not working necessarily with [vendors] that always want to tell you exactly what's going on, partly because they know it opens them up to criticism and because it's proprietary and sort of a competitive disadvantage," Engler said.
How AI can introduce bias into hiring
It's more than a little ironic that the controversy over hiring algorithms is swirling around vendors that hype AI as key to reducing hiring bias on the theory that machines are less biased than people.
But bias can creep into the AI in recruitment software if the data used to train machine learning algorithms is heavy with historically favored groups, such as white males. Analyzing performance reviews to predict the success of candidates can then disadvantage minorities who are underrepresented in the data.
Another AI technology, natural language processing (NLP), can stumble on heavily accented English. Facial analysis tools have been shown to misread the expressions of darker-skinned people, filtering out qualified candidates and ultimately producing a less diverse talent pool. The use of AI in automated interviewing to detect emotions and character traits has also come under fire for potential bias against minorities.
Few vendors have had to thread the needle on the ethical use of AI in hiring more than HireVue Inc., a pioneer in using NLP and facial analysis in video interviews.
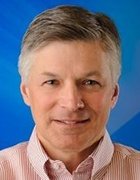
HireVue dropped facial analysis from new assessments in early 2020 after widespread criticism by Engler and others that the AI could discriminate against minorities. More recently, the software's ability to analyze vocal tone, which Engler said holds similar risks, has come under scrutiny after customers expressed "nonspecific concerns," according to HireVue CEO Kevin Parker. He said the company has decided to stop using that feature because it no longer has predictive value. It won't be in new AI models and the company will remove it from older ones that come up for review.
HireVue will instead rely on NLP, which has improved so much that it can produce reliable transcripts and not be thrown by heavy accents, said Lindsey Zuloaga, HireVue's chief data scientist. "It's the words that matter -- what you say, not how you say it."
Steps taken to mitigate bias
Three vendors interviewed -- HireVue, Modern Hire and Pymetrics -- all say they regularly test their AI models for bias.
"We do a lot of testing and issue a pretty voluminous technical report to the customer, looking at different constituencies and the outcomes, and the work we've done if we discovered bias in the process," Parker said.
Zuloaga described the bias mitigation process in more detail. "We have a lot of inputs that go into the model," she said. "When we use video features, or facial action units [facial movements that count toward the score] or tonal things -- any of those, just like language, will be punished by the model if they cause adverse impact." The company reviews models at least annually, she added.
Pymetrics Inc. takes a different approach to hiring, applying cognitive science to games designed to determine a candidate's soft skills, though it also offers structured video interviews.
Pymetrics essentially uses reporting to monitor for bias, according to CEO Frida Polli.
"The best way to check if an algorithm has bias is to look at what the output is," Polli said. "Think of it like emissions testing. You don't actually need to know all of the nuances of a car engine … to know whether it's above or below the allowed pollution level."
Before releasing any algorithm it builds for an employer, Pymetrics shares a report to confirm that the algorithm is performing above the four-fifths rule -- "so, basically free of bias," Polli said. "We show them ahead of time that we are falling above that threshold, that the algorithm is performing above that before we ever use it." Then, after the algorithm has "been in use for some period of time, we test it continually to ensure that it hasn't fallen below that guidance," she added.
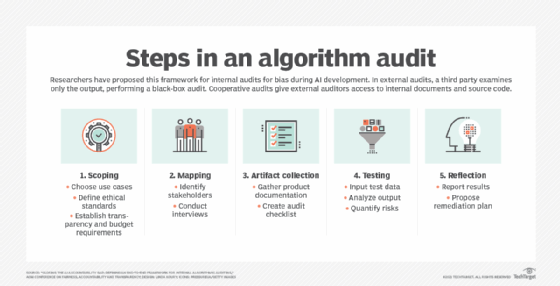
How algorithmic auditing can mitigate AI bias
Auditing algorithms could be the most practical way to verify that an AI tool avoids bias. It also lets vendors reassure customers without exposing their intellectual property (IP). But in its current form, algorithmic auditing is extremely labor-intensive.
Jake Appel, chief strategist at O'Neil Risk Consulting & Algorithmic Auditing (ORCAA), explained the process. His company conducted an audit for HireVue that was released in January.
First, ORCAA identifies the use case for the technology. Next, it identifies stakeholders and asks them how an algorithm could fail them.
"We then do the work of translating their concerns into statistical tests or analytic questions that could be asked of the algorithm," Appel said. If problems are detected, ORCAA suggests fixes, such as changing data sets or removing an algorithm.
Vendors, academics and algorithmic auditors disagree on whether it's necessary to see the program code to determine bias.
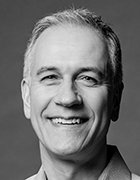
Mike Hudy, chief science officer at Modern Hire, a HireVue competitor, said transparency must be balanced against a vendor's need to protect its IP -- but claimed some vendors use that as an excuse not to share code. He said Modern Hire, which completes 400 audits a year for customers, takes an open source approach that allows it to expose some of its code, though the candidate-scoring algorithms are its IP and must be protected.
Customers can use the data to run their own audits. "But we don't assume our clients are going to do that," Hudy said. Modern Hire also conducts "macro-level" audits across clients to see if its AI models need adjusting.
Vendors criticized for audit quality
Critics like Engler have poked holes in the algorithmic auditing practices of the vendors.
For one thing, Engler said HireVue discourages downloading of the ORCAA audit by requiring a nondisclosure agreement. But HireVue disagreed that it's a true NDA.
"We've invested a lot of time and energy into [the audit]," Zuloaga said. "It's a piece of our intellectual property that could contain what we would consider proprietary methodologies about how we do what we do. Just from a fair use perspective, we want people to read it; we want it to be accessible. We don't want it to be copied. We don't want it to be quoted haphazardly. But anyone that is interested has access to it. We just want to make sure it's used thoughtfully."
Zuloaga said it's tricky to explain the models so customers can understand them without giving job candidates clues for boosting their scores. "We could reveal probably a little bit more, but I think we're doing a pretty good job in terms of the nuts and bolts of how the model actually works." The AI follows the standard AI development practice of supplying the data to train the machine learning algorithms and then optimizing the model, she said.
Engler and others knocked HireVue's audit for being too narrow, but Appel said there were good reasons for keeping a sharp focus.
"We worked hard to get to a use case that we felt we could explore exhaustively," he said. "We need to look in a particular context so we can really understand what the fairness concerns are in that context, and then we can explore them deeply. If the audit feels too narrow, the answer might be to do more audits of more specific contexts rather than make the audit less penetrating."
Another prominent critic of algorithmic auditing is New York University researcher Mona Sloane, who says it is subject to misrepresentation and interference by vendors, on whom auditors are financially dependent.
Sloane, who joined in the HireVue criticism, also criticized a peer-reviewed paper that Pymetrics co-wrote after Northeastern University computer scientists audited its software. She wrote that the cooperative approach advocated by the authors only focuses on whether an AI tool works as intended, ignoring important issues like the diversity of the developers.
Polli characterized the Pymetrics audit as more comprehensive and transparent than HireVue's. She noted that the Northeastern auditors had access to program code and that Pymetrics had them sign an NDA only to protect its IP.
"It's absolutely possible for a company to do that," she said.
She called the Pymetrics and HireVue audits "night and day" with three main differences: The HireVue audit wasn't subject to peer review; the entire Pymetrics platform was subjected to the audit, whereas ORCAA only examined one component of HireVue; and the auditors didn't have access to HireVue code.
"The ORCAA team went in and asked HireVue a bunch of questions, HireVue gave a bunch of answers and nobody confirmed anything," Polli said.
The HireVue officials defended their approach, saying that while they can and do make the code available, it's not what counts in evaluating for bias.
"It is less about the lines of code and the data science component, because most of what we do is based on readily available technologies that third parties have created that do a lot of the number crunching," Parker said. What matters is showing how HireVue has thought through the effects on the different stakeholders in the hiring process, he added.
Playing by the rules -- but what rules?
Simply ensuring that a hiring tool meets the four-fifths standard is not the same as eliminating bias and improving diversity.
"The four-fifths rule is only supposed to be a rule of thumb and is not a wholesale legal defense of your algorithm," Engler said. "It doesn't mean that you're not doing anything discriminatory. It means that you've passed the bare-minimum check."
Parker said another drawback of four-fifths is it only covers groups named as protected classes in EEOC guidelines that are several decades old and, for example, don't mention neurodiversity, which are differences in brain function and social behavior, such as autism.
Furthermore, Polli said software vendors and their customers are allowed to -- and frequently do -- violate the rule if they can show that an employment practice is "job-related" and predictive of outcomes.
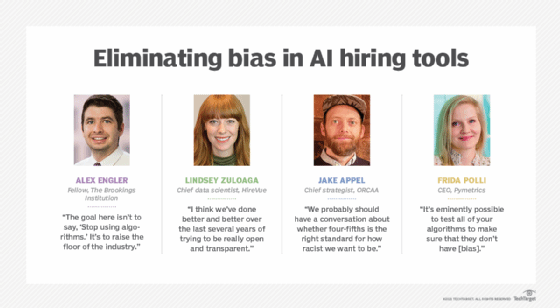
Government regulation seen as necessary and inevitable
U.S. law requires companies to minimize the impact of bias on their hiring of protected classes of people. That was true long before AI came to recruiting. Now, governments are turning their sights on the technology. For example, in the European Union, the General Data Protection Regulation (GDPR) restricts automated decision-making that uses personal information and requires transparency into the process.
U.S. federal agencies, state governments and municipalities have also begun to adopt enforcement mechanisms. The state of Illinois led the pack in January 2020 with a law regulating AI-based video interviews.
"The goal here isn't to say, 'Stop using algorithms,' it's to raise the floor of the industry," Engler said. "It's to put the regulators in a position where they can look across the industry and say, 'These firms are really half-assing this, we're going to take them to task [and] make it harder to run a lazy, unethical shop.'"
There are indications the EEOC may be preparing to take action, Engler said, citing a recent article by EEOC Commissioner Keith Sonderling. In the article, Sonderling warned that algorithms "could multiply inequalities based on gender, age and race" if they aren't used carefully. "Employers who use AI to make hiring decisions must understand exactly what they are buying and how it might impact their hiring decisions," Sonderling wrote.
Engler said the EEOC has broad authority to review an entire sector. "They could be pushing companies that contract with the government more to evaluate these systems because they are also part of the Department of Labor, [which] works with government contractors."
The Federal Trade Commission is also taking notice, Engler added, and could prohibit vendors from claiming their AI is unbiased. Meanwhile, the New York City Council is considering a bill requiring automated employment decision tools to come with free annual bias auditing.
Polli favors requiring platform vendors to collect the results of audits and report them publicly.
"It's eminently possible to test all of your algorithms to make sure that they don't have [bias], and not release any that do," she said. "It's much easier than people make it out to be." The real problem, she added, is vendors currently have no incentive to do so.
"That's what we would be advocating for: some sort of mechanism by which you aggregate your results across all of the times you've implemented your platform, and that you publicly report on those numbers." Polli said Pymetrics made that case before the EEOC and advised it on possible reporting mechanisms.
According to Appel, the best place to start is to take existing rules about fairness and nondiscrimination and translate them into terms algorithm builders and users can understand. The four-fifths rule is a good example, but it's more complicated than that.
"Hirers can optimize to the four-fifths rule -- be just that racist but not more racist, and know that we're going to be inbounds legally," he said. "We probably should have a conversation about whether four-fifths is the right standard for how racist we want to be. But once there's a guardrail that can be understood and obeyed by a builder of an algorithm, then there is the infrastructure for accountability."
Other technical solutions that could help
Since algorithmic auditing is so labor-intensive, is there an opportunity to automate it?
Engler said a tiny but growing industry in algorithmic auditing software looks promising. He mentioned Arthur, Fiddler and Parity AI as players.
Zuloaga isn't sold. "We played with a couple of packages and felt like we were already doing way more than what they were doing," she said.
Appel said automation targets are limited because interviewing stakeholders about their notions of fairness is too important to skip. He said generic software exists for asking standard questions to apply to hiring models, but it's not the right approach because questions should fit a specific context.
"Having said that, once you work with a particular client and you've figured out the questions for that context, the statistical tests and monitors that you build are generally pretty straightforward and may be repeatable," he said. "That can be an automated process."
Different companies in the same industry using the same kind of software could then use the same questions. Appel said ORCAA is developing a software "sandbox" that clients and possibly regulators could use to automate this kind of testing.
But testing more frequently isn't necessarily better, according to Polli, who said the Uniform Guidelines prescribe testing frequency, basing it mostly on hiring volumes.
"You do it at an interval that makes sense, and usually that interval is tied to how many people are going through your platform on a daily basis," she said. "If you test too often, you might find some statistical deviation just because of noise," like the daily fluctuations in a person's weight.
Standardizing and mandating audits could be the solution
Parker said algorithmic auditing needs to reach the level of standardization and legal backing of financial auditing. "That's where I think legislative or industry bodies should be out in front leading, saying, 'Here's what we mean when we say an audit, here's what an audit report looks like and here are the things that you need to do.'"
He said it's unrealistic to audit hundreds of AI models in a product and prefers auditing the process used to develop the models to ensure the right controls are in place.
Pymetrics is pushing for cooperative audits like Northeastern's to become the standard. Polli disagrees with Sloane's criticism that they invite vendor influence, saying cooperation is only required to give auditors access to software code. She noted that Northeastern had full independent publishing rights.
"We're not alone in thinking this is useful," Polli said. She named the Algorithmic Justice League -- a nonprofit that successfully lobbied against facial recognition use at Amazon, Google and other tech giants -- as a supporter and said it is developing a framework for algorithmic auditing.
Other methods besides tech can help reduce bias
But there are other ways to mitigate bias in employment practices as part of using the AI tools, according to the vendors.
To start, they all claim to base employment decisions on industrial and organizational (I-O) psychology, the main discipline for applying statistics and psychology to workplace issues and understanding the job-related factors that affect employee performance.
"Just because we have AI and those more advanced analytic tools, it doesn't change what works. It doesn't change the rules of the game," Hudy said. "We're relying on principles that have been around for decades."
Compliance officers, attorneys, HR staff and talent acquisition managers have typically been trained in the Uniform Guidelines, disparate impact and the four-fifths rule, Hudy said. "We're presenting in that language … rather than complicated statistics that data scientists use."
Keeping people in the decision-making loop is another guardrail against AI bias, he said. "We're saying, 'Now it's your turn. This is our recommendation, here's the video, you watch it and use the same criteria.'"
Like Modern Hire, HireVue puts a lot of stock in the standardization provided by structured interviews, which use carefully crafted questions to glean information known to be predictive of success. Parker called structured interviewing "the best way to hire people -- by asking everybody the same questions and making sure they're about workplace competencies, not about whether you were captain of the varsity lacrosse team at Princeton. That consistency improves the fairness of the process."
Another way to keep bias from sneaking in the back door is not to rely on resumes. "Resumes bring a lot of a lot of flawed information. We're not looking at LinkedIn profiles and self-declared competencies," Parker said.
Putting AI in its proper place
With so much controversy, complexity and uncertainty around the technology, how should organizations think about their use of AI in hiring?
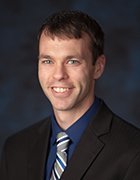
Ben Eubanks, chief research officer at Lighthouse Research and Advisory, said it's important to watch out for bias at every stage in the hiring funnel.
At the front end, job ads might be free enough of bias to attract diverse candidates who later get hung up in the interview process. "If by the end, 2% of the candidates you're making offers to are diverse, you're doing something wrong," Eubanks said. "You've got to go back and figure out where it is."
He said his firm asked job candidates and employers where they are most comfortable with AI tools being used in the hiring process. "It tailed off all the way down until the lowest point was at the actual offer. Very early on, everybody says AI is fine: 'Let it be a bot, let it be an algorithm, we don't care. But the closer we get to making a decision or commitment, the more we want a human in the mix.'"
Nevertheless, whenever people step into the process, bias is introduced, however inadvertently and unconsciously and despite the best intentions. Eubanks said the best role for AI, rather than automating the initial selection of candidates, might then be to nudge people to make sure their final list of candidates is diverse.