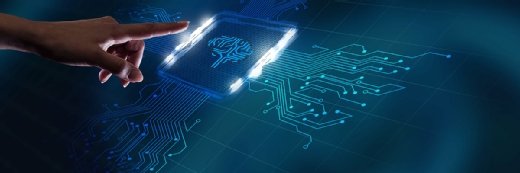
putilov_denis - stock.adobe.com
A guide to the key stages of AIOps
The key stages that make up AIOps all play an important role in achieving desired results. Successful adoption hinges on a team's ability to master them.
AIOps can increase the efficiency of IT workflows. Because AIOps encompasses a variety of key stages, learning its fundamental areas and best practices is essential for a successful rollout.
AIOps comprises a number of key stages: data collection, model training, automation, anomaly detection and continuous learning. ITOps has always been fertile ground for data gathering and analysis. Combining IT with AI and machine learning (ML) creates a foundation for a new class of operations tools that learn and improve based on the data they gather.
What is AIOps?
AIOps refers to the process of integrating AI into operational workflows to improve IT services and gain automated functions for services and infrastructures. AIOps has become more attractive due to the complexity of distributed workforces, along with hybrid and multi-cloud environment adoptions. Implementing AIOps creates a more proactive workforce that can quickly uncover unknowns, find answers and streamline processes to build better software.
DevOps teams generally start by automating their IT and technical services by applying ML to monitor infrastructure, operations and data. AIOps also employs natural language processing, event correlation and statistical models to achieve results that benefit the ITOps workflow. The key stages of AIOps -- data collection, model training, automation, anomaly detection and continuous learning -- all work together to achieve these results.
Key benefits of AIOps include monitoring systems, automating runbacks, activating responses to real-time events, and correlating related events and incidents into single issues. AIOps processes can also uncover context, pinpoint root causes, alert the right IT administrators or team members, and even respond to cyberthreats.
Data collection
One of the key stages of AIOps is data collection. The data that an AIOps platform depends on includes historical systems data and events, logs, network data and real-time operations. During data collection, DevOps teams gather this information. They analyze past system states and identify trends and anomalous patterns.
The main problem that an organization wants to resolve influences the types of data that DevOps teams research. Teams might want to ask the following questions:
- What is the source of alerts?
- How critical are the alerts?
- What telemetry data does an organization accrue?
- Which systems need to be constantly monitored?
AIOps typically uses a big data platform to bring together siloed data from other IT components within an environment. After effectively aggregating data through extracting, transforming and loading, ITOps teams can then use the data to inform the processes that they undertake.
Model training and automation
Once a team aggregates the necessary data, they can pipeline that data to train ML algorithms and create a functioning model.
One goal for IT might be to proactively scale their traditional infrastructure to meet new demands. In contrast to manually monitoring CPU or RAM usage, IT teams can use AIOps to program an autoscale event based on deep learning algorithms, which include timelines, inbound traffic projections and the various compute instances that serve applications. For companies that want to undertake massive scale-ups on end-user activity, the shift from reactive to proactive scaling offers cost reductions by predicting optimal capacity points.
IT organizations can use training data sets to guide network usage and test their AI models. Whether it's the responsibility of site reliability engineers or DevOps teams, employing automation and ML can help ensure AI model accuracy and high automation levels. Successful automation depends on creating model effectiveness, monitoring pipeline performance for anomaly detection, gathering inferences from anomaly types and then generating alerts. These AIOps processes can then effectively take actions like performing automatic patching and triggering real-time rollbacks to more secure states.
AIOps can also then employ reliable information accessible via analytics dashboards to record these alerts, gain new insights and gather useful recommendations. Teams can use this data-centric approach to counter siloed IT monitoring and to automate scripts and minor manual operations to achieve effective workflows, predictive processes and business automation.
Anomaly detection
Along with analyzing data from apps and IT infrastructure and making comparisons with historical information, AIOps detects anomalies via response times, CPU output and memory usage to alert administrators in emergency cases. Using these data analyses and making inferences, AIOps can reduce false alarms and minimize the effects of irrelevant notifications. That reduction is critical in terms of strengthening overall infrastructure security. When detecting malware exposures, advanced ML algorithms can uncover other breaches as well to ensure efficient real-time responses.
Such gains are also possible when employing AIOps to manage storage. For example, IT teams can train models to handle output workloads based on the highest efficiency and usage. Administrators rely on automatically generated alerts if performance reaches lower IOPS or if a disk has reached capacity. AIOps can automatically adjust storage capacity by proactively installing new volumes where necessary on a proactive basis.
Continuous learning
Successful deployment of AIOps hinges on the ability to ensure continuous learning. Applying a continuous cycle of improvement for an AIOps deployment ensures tool set integration. Part of applying a continuous cycle means continually evaluating to ensure the team is meeting preset standards and grading performance.
For example, as AIOps systems become adept at detecting anomalies and performing other predictive analytics on large data volumes, they can learn and expand the scope of problems that they handle. IT teams must be cognizant of the accuracy that is necessary in the model training phase. To achieve the highest levels of AIOps, organizations should integrate as many systems as possible under one umbrella. Without effective integration, problems can appear somewhere else in a system. For instance, a network issue related to cyberweakness or a slow database could cause end-user problems.
AIOps best practices
Adopting AIOps includes a series of best practices to consider. For one, a key motivator for AIOps adoption is ensuring cyberware protection. Several factors contribute to deficits in this area, and some cyberware questions for adopters to consider are the following:
- Does the current system suffer from downtime, service interruptions or service degradation that affect service-level objectives?
- Are ITOps teams negatively affected by alert fatigue and noise that inhibit them from identifying and responding to critical issues?
- Can ITOps teams quickly identify root causes of the breaches, or is it difficult to isolate the source?
AIOps is complex and requires undertakers to have data science and ML knowledge. Without employees who are skilled in these areas, organizations run the risk of unsuccessful adoption. It's also critical to roll out AIOps incrementally and only after defining the challenge that needs solving. Detail the nature of the problem, the impact it has on the business, the IT infrastructure and its expected outcomes. Then, begin the rollout process gradually.
Lastly, consider the ethical implications of using AI to perform ITOps. Organizations must ensure that the autonomous decision-making of machines aligns with established goals and values to confirm AIOps integrity.