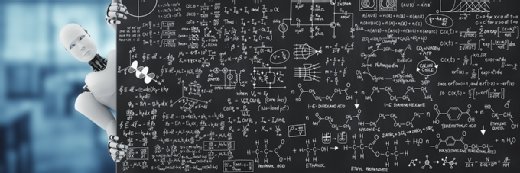
Blue Planet Studio - stock.adobe
Where the future of AIOps use cases will take IT ops
AIOps is poised for rapid growth and maturity as it becomes accepted in the market. Organizations should draw plans to integrate it into DevOps and systems management.
The use of AI in an IT operations sense -- i.e., AIOps -- is still a relatively new approach. The idea is to simplify IT management and automate problem resolution while accelerating processes in complex modern IT platforms.
Hybrid cloud-based platforms create masses of data -- far too much for operations staff to analyze in real time. Even data aggregators struggle to deal with such volumes; the need to intelligently filter out the noise from the real data has grown significantly.
AIOps aggregates data sets, filters and analyzes them to identify the root cause of any problem, and then either automatically remediates the problem or raises it with a suitable resource to address the problem. This can be applied to the physical hardware layer, the virtualized platform layer, or the VMs, containers and applications running on top of the platform.
But AIOps use cases are only a small step forward from many long-established systems management platforms.
Problem feedback loops
Rather than wait for problems to appear -- and for users to contact the help desk -- AIOps enables IT admins to compare different variables against a baseline created at instantiation.
For example, a memory leak within an application could cause damage to performance in a slow and manageable timeline -- or it could start to demand extra resources at a cost to the company. AIOps identifies where memory use is growing and whether that growth drops down to normal levels. Therefore, it can flush the memory store and, if possible, bring performance back in line, as well as raise a formal problem ticket for the development team to address the underlying issue. As AIOps technology improves over time, it can begin to identify the root causes on its own to prompt developers to make the requisite changes and pass code back down the DevOps stream.
Continuous learning
The initial baseline an AIOps system creates is not the full extent and authority of information. The AIOps system must ingest large amounts of data to learn as much as it can -- for example, a group of new users added to a platform will affect many variables, such as resource requirements or licensing.
Admins should create new baselines on an ongoing basis. The AIOps system must be able to report on why the new baseline is as it is, and what that change means for the company now and -- via intelligent extrapolation -- in the future.
Predictive issue identification
Based on global data, vendors will be able to rapidly analyze the performance of an IT kit from single chips through assemblies to whole servers, storage systems and network hubs.
Knowing when an item in the physical infrastructure is likely to cause problems can help IT admins organize equipment replacement schedules before failure happens. It can also extend the life of equipment where analysis shows that such failure is unlikely and replacement to avoid possible failure can be delayed safely.
Workload relocation
Currently, orchestration software relocates workloads from one platform area to another. However, it still tends to require a manual trigger. With AIOps, AI can move that same workload based on variables such as resource availability, resource cost and number of users accessing the workload. It can also optimize overall costs by offloading licenses across the board, from application through virtual services to OS and cloud use.
Event-driven processes
Microservices-based composite applications are partly hard-wired -- even now, at time of publication -- in which one service calls another through named calls. AIOps enables IT admins to sidestep this activity with call abstraction. This then enables AIOps to help optimize the whole flow of processes, by metrics such as value to the company, volume or maximum costs.
A calling microservice can hand over a set of metadata that defines its needs. AIOps then has access to all the data across the entire platform, including, where appropriate, public services. The calling service's needs can then be matched with a responding service's capabilities in real time, and the process is complete. AIOps, a major data-aggregating service itself, can then maintain a full audit of what was done while handing over other data to external systems, such as billing systems.
Security
As AIOps monitors so many data sources and carries out basic analysis, pattern matching and advanced heuristics, it is well placed to monitor what is happening in the realm of security.
Future AIOps systems should recognize -- at least to an extent -- intrusion, DDoS, Trojan, worm and zero-hour attacks; possibly malicious abnormal activity by users; and phishing attacks. Whereas the AIOps system itself will unlikely be able to directly remediate any such issues, it should put in place measures to alleviate any problems while other systems deal with the root cause -- alerted directly via the AIOps feedback loop system.