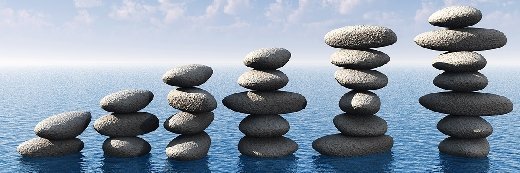
Sergey Galushko - Fotolia
Data hub vs. data lake: Deciphering the differences
Data lakes and data hubs are approaches to data management that are typically opposed. Here are the main differences between these two storage options.
Companies have realized that the more data they gather, the better they can understand their customers and users. And the way a company stores its data can allow for a more balanced and intelligent view of its operations.
Two storage options are data lakes and data hubs. A data lake stores raw data similar to a regular lake, while a data hub is composed of a core storage system at its center with data in spokes reaching out to different areas.
There has been an ongoing debate on data hub vs. data lake and which is the best way to approach data gathering and storage. Though these are both common terms, differentiating between the two can still be a challenge.
Defining a data lake
A data lake is a centralized option in which all forms of data can be stored in a variety of ways. There is no need to translate data to a singular form, as a data lake can hold a vast amount of raw data in its original format.
Data lakes were created by companies because they understood the value of their data, said Hossein Rahnama, MIT machine intelligence professor and founder and CEO of Flybits. Bringing all that data together allows companies to better predict the needs of their customers and the needs of their business.
A data lake acts as a repository for data from all different parts of an organization. This makes data storage easier than other storage solutions but can become a problem when it comes to drawing that data back out. In order to retrieve desired data from a data lake, it must be queried, and data lake users may struggle with accessibility. Highly technical skills are often required to find relevant information and draw conclusions from that data.
"Companies that are going to be successful leveraging their data lake are the ones that are also building a creative and interactive layer on top of that data lake so non-IT experts can also leverage data assets to build new capabilities," Rahnama said.
Data lakes are often associated with a Hadoop framework; however, many vendors now support data lake architectures, including Amazon, Cloudera and Microsoft. Many even offer the option to deploy data lakes in the cloud.
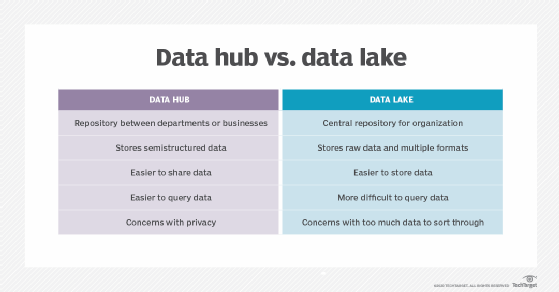
Establishing a data hub
A data hub can be thought of as a hub-and-spoke approach to storing and managing data. Data is physically moved and reindexed into a new system. This provides more structure to the data and permits diverse business users to access information that they need more rapidly than in a data lake.
Data hubs are usually created as a joint effort between complementary businesses, Rahnama said. It could be between a telecom operator, a bank and a supermarket, and they will all come together to share insights and elements of data.
Each spoke of this wheel would have access to some or all of the collective data gathered, depending on what they were looking to gain from it.
"The telecom operator may have a data cloud [storing] telecom information, the financial organization may have another cloud owning transaction data and the supermarket may have another data set," Rahnama said. "Now, these organizations have two options to create a data alliance or a data hub; they may agree to host their data in a centralized repository that can be accessible by all three of them."
This brings up concerns about privacy, as information collected by a bank could find its way to a completely different company. To ease these worries, it is critical for companies using data hubs to ask for user consent to sharing their data.
Similar to data lakes, data hubs were originally built on a Hadoop framework, but there are now other popular vendors, including MarkLogic and Google.
Data hub vs. data lake
Creating a data hub does not mean that data lake architecture is unavailable, however.
"A data hub, at the same time, may or may not use a data lake architecture," Rahnama said. "I can use a data lake with different stakeholders to participate in. Or I can completely decentralize it and leverage something like a blockchain or edge of the cloud or other decentralized mechanism to still form the alliance but in a decentralized way."
Giving numerous businesses access to a communal data lake would, for example, combine both a data lake and a data hub in one solution. This would increase the amount of participating companies but would do nothing to mitigate the accessibility of data lakes.
Data hub and data lake use cases
Both models are strong contenders to reduce data silos, as they are built to be accessible across business divisions' access to the same data.
The multipronged approach of a data hub is popular for use cases that require multiple interpretations to the same data. For example, analyzing similar data for both marketing and financial analytics. This makes data hubs popular for enterprises that analyze various types of data to perform tasks, such as fraud detection and customer service.
Because data lakes are built to store data until it's necessary, they tend to be more popular among enterprise with a less urgent need for data. Data lakes are popular for storing IoT data and archival data. Active archive data stored in a data lake can be used by data scientists for research across industries, including health sciences.
The debate between data lakes vs. data hubs isn't straightforward. With both filling different needs and having a combination as a possibility, the right data management approach boils down to company needs.