6 key steps to develop a data governance strategy
Data governance shouldn't be built around technology, but the other way around. Existing infrastructure, executive support, data literacy, metrics and proper tools are essential.
Data governance at one time was typically the concern of IT managers, database administrators and other data management professionals. That scenario has changed dramatically.
A combination of serious data leaks causing reputational damage to major corporations, a public outcry about the need for better data privacy protections and a strong response from government regulators in Europe and the U.S. have helped to place data governance on the C-suite agenda.
Why you need a data governance strategy
Make no mistake, a data governance strategy is imperative. Ungoverned, the increasingly large volumes of data collected and used for more and more purposes in modern business operations can create a legal, technical or reputational morass. With good governance, enterprise data can be more consistent even across divisional boundaries, more accessible and easier to use, enabling better informed business decisions.
Data governance policies don't restrict innovation. Quite the opposite. By creating a more reliable data foundation and reducing the risk of misused data, good governance enables new ideas to flourish.
It can be tempting for IT leaders and business executives to purchase a governance strategy by contracting a consultancy or software vendor that promises a more-or-less packaged set of policies and tools. But this approach can be disruptive and counterproductive. Existing governance processes may break down when a new strategy is introduced, and busy end users will often ignore it in the rush to get their work done.
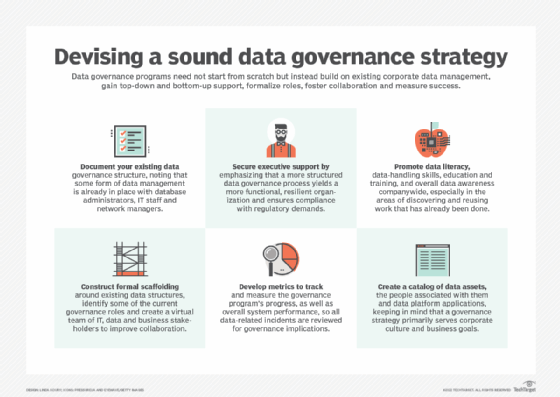
The best data governance strategy is developed organically in-house to harmonize with business operations. Following are six undramatic but entirely practical steps to develop an effective data governance strategy.
1. Document where you are now with data governance
For many enterprises, just getting started with data governance can be a big, burdensome step that requires a budget. But the simple truth is, your company is probably already doing some level of data governance that can be developed into a strategy.
The data governance may not yet have been documented as a policy, but people are already in place to manage corporate data, including a database administrator who sets permissions for access, IT staff that diligently back up and restore data and a network manager who checks that the business intelligence tools are licensed properly.
From there, a directory of the company's data assets and a list of those who exercise any responsibilities for that data and their direct reports should be created. And don't be surprised if some sobering, if not shocking, oversights and gaps in coverage are revealed that need quick attention.
This informal approach may reflect the messy reality of your company's current governance processes, but the result will be a simplified catalog of assets, roles and accountabilities. Once completed, it's time to get more strategic.
2. Secure executive sponsorship for a governance program
Much of the daily work of data governance occurs close to the data itself. The tasks that emerge from the governance strategy will often be in the hands of engineers, developers and administrators. But in too many organizations, these roles operate in silos separated by departmental or technical boundaries. To develop and apply a governance strategy that can consistently work across boundaries, some top-down influence is required.
How can you win executive support for an initiative that may not show a clear advantage to the bottom line? One method, usually chosen by default, is to promote fear, uncertainty and doubt. Horror stories of fines for breaching the EU's GDPR law on data privacy and protection might keep business leaders awake at night. This drastic approach may generate some interoffice memos or even unlock some budgetary constraints, but that would be a defensive reaction and possibly create resentment among stakeholders, which is no way to secure long-term good data governance.
Instead, try this incremental approach, which should be much more attractive to executives: "Data governance is something we already do, but it's largely informal and we need to put some process around it. In doing so, we will meet regulatory demands, but we will also be a more functional, resilient organization."
Executive buy-in is critical because data governance demands the participation, cooperation and support of individuals, departments and the entire enterprise.
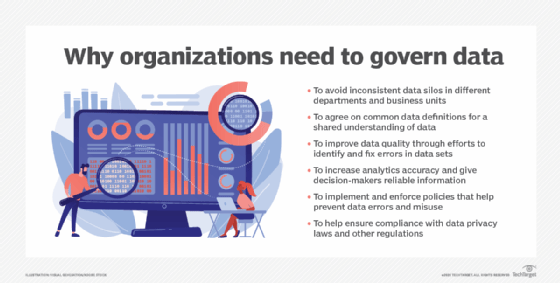
3. Increase data literacy and skills in your organization
Bottom-up support for data governance is just as important as top-down sponsorship. Business users who understand the value of data better understand the need to protect data assets. That's why improving data literacy is a worthwhile step in developing a successful governance strategy.
There's another practical reason why increased data literacy helps raise up data governance efforts. A very common dysfunction among data-centric organizations is the frequent re-creation of reports, dashboards, spreadsheets and even entire databases because employees often don't know how to discover or reuse the work that has already been done. Reusability is more efficient, more consistent and less prone to error but requires some knowledge of data-handling techniques and best practices. Fostering wider organizational data literacy and skills through training is a major step toward better governance.
4. Work within the existing organizational structure at first
It's too much to ask -- and too risky -- for a company to reorganize just to improve the governance of data. Instead, construct some formal scaffolding around the existing ad hoc data structures -- at least as a starting point. Identify some of the key roles involved in the governance program and, depending on complexity, create a virtual team to improve coordination and collaboration. Consider the following questions when developing a governance strategy:
- Who advises or is consulted on what the data governance controls should be? To learn who has helpful insights to share, cast the net widely by including business users, the office of general counsel and IT teams.
- Who implements the controls? Some aspects of this work can be quite technical, such as applying row- and column-level permissions in a database, and other aspects may be more administrative, like HR ensuring that a new hire is assigned the right job title to automatically secure the right permissions.
- Who measures the success of the data governance program and is accountable for doing so?
- A larger question is, who's responsible for the governance work overall? Who coordinates across different departments and business units?
By establishing a data governance council that includes business representatives and those with data responsibilities, collaboration on setting policies and making decisions can be extremely valuable. Over time, when governance activities are more established and formalized, full-time roles will emerge, such as a data governance manager or even a vice president. Operationally, some workers will take on the role of data steward, responsible for directly implementing governance policies.
5. Decide how to measure the governance program's success
For a data governance strategy to gain traction and recognition, it's important to determine the program's success. Data governance may not measurably affect a company's bottom line, but bad governance, or no governance at all, may indeed have a financial impact. Regulators have the power to impose heavy fines, not to mention the financial cost of making restitutions and suffering brand reputational damages for violating data protection and usage laws.
Since "avoiding being fined" is not a reliable key performance indicator, how can the success of a governance strategy be measured?
One way to measure success is to specifically identify data-related incidents as part of an existing IT issue management system, while ensuring that all data-related incidents are reviewed for governance implications. Users losing access to a database may be a simple incident, for example, but if it's resolved by elevating their permissions to allow access to more data than strictly necessary, that would be a governance issue. Recording, investigating and tracking such cases will quickly increase a body of knowledge and associated metrics about how data governance is improving or degrading over time.
It's also worthwhile to measure overall system performance. For example, tracking how many people have access to a system, their permissions and how often they use them provides a practical benchmark and greater insight into how data is being used. In addition, track the quality of data, including accuracy, completeness, consistency, timeliness and duplication.
Although these metrics may not be an immediate indicator of governance success, they will be helpful nonetheless. For instance, more analytics users may be the result of increased data literacy, but if those new users are creating more copies of the data in more reports instead of reusing existing reports, that would suggest poor governance.
6. Choose technology that fits your strategy, not the other way around
It's recommended above that you create a directory of data assets and the people associated with them -- an informal catalog, not a full data catalog. Data catalogs that provide a single, consistent interface to curated data for use cases across an entire enterprise are useful tools. The same goes for analytics catalogs, which help manage how data is used through objects like dashboards, reports and data visualizations.
However, don't build a data governance strategy around technology. Instead, select tools that specifically serve the strategy and your organization's needs. The governance program will then correspond with the corporate culture and goals rather than work against them.
Taking your data governance strategy forward
These six steps to develop a viable and successful data governance strategy may not be exciting technically, but the value of a pragmatic process will steadily increase. Most important, data governance will be viewed not as a challenging discipline that's being imposed on your organization, but as one that improves how the business is run and helps ensure that the organization recognizes and values data as a critical business asset.
Thought leaders will emerge, data skills will be upgraded, and the result will likely be a more insightful and collaborative organization. Data governance alone won't produce these improvements, but it provides an important foundation to make such advancements possible. For those among your company's teams who are trying to do more with data, better governance gives them the freedom and capabilities to do just that.