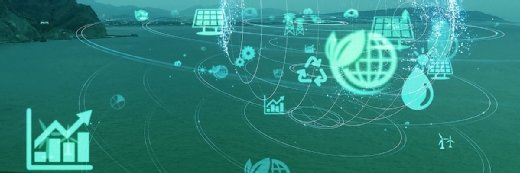
Getty Images/iStockphoto
AI examples that can be used effectively in agriculture
AI technologies can be utilized in agriculture for increased visibility into factors affecting crops, increased efficiency and minimized risk.
The digitization of everything has led to the proliferation of IoT and IIoT devices, working in conjunction with AI software, to collect and analyze information, including in agriculture.
The overarching goal is to maximize revenues from fruits, vegetables and livestock by understanding the factors that impact them, such as weather, temperature or soil composition.
"AI will form an important basis for reducing labor costs and increasing productivity, but for many, the focus has shifted away from moonshot ambitions to problem-solving pragmatism," said John Gottula, director of crop science at advanced analytics software provider SAS.
Farming has always been a labor-intensive undertaking and it still involves considerable human intervention. Many examples of AI in agriculture allow farmers to understand their crops faster, at scale and at a more nuanced level.
Agricultural challenges can be mitigated with AI
For starters, in addition to monitoring sunlight, AI systems involve computer vision to "see" plant health and identify pests. In fact, networks of sensors collect data on temperature, soil, watering, etc. that are combined to provide a better picture of crop health so growers can maximize crop output and quality.
That's a lot of data -- too much for humans to analyze effectively in a timely manner. Therefore, AI, IoT, 5G and alternative network connections (like satellites which extend cellular coverage) work together to monitor crops or automate previously manual processes.
"New AI techniques can automate complex tasks and unlock previously hidden insights across the spectrum of agricultural data," Gottula said.
Examples of AI in agriculture assist in the following ways:
- Research and development. Machine learning, including deep learning, can teach systems to detect outliers in data or match data against known patterns with unprecedented precision and recall. The result is faster innovation and greater efficiency.
- Faster identification of research data. Applying text analytics and natural language processing to key terms or research greatly reduces or eliminates manual online and paper sorting.
- Greater efficiency and effectiveness. AI is being used for intelligent automation, such as drones capturing images of crops so the farm yield can be estimated.
- Early detection of pests, diseases and weeds. Drones and computer vision are being combined for faster assessments of field conditions and to prioritize integrated pest management strategies.
- Precision agriculture. Data analytics, sensor technologies, IoT, machine learning and cognitive computing are monitoring soil moisture, light and humidity on a continuous basis.
- Analyzing market demand. AI can simplify crop selection and help farmers identify what produce will be most profitable.
- Managing risks. AI provides farmers with the forecasting and predictive analytics to reduce errors and minimize the risk of crop failures.
- Weather forecasting. AI enables farmers to forecast temperatures and predict how many fruits or vegetables a harvest will yield. It can also help farmers identify optimal irrigation patterns based on predicted rainfall.
Agricultural AI examples are expected to expand going forward
Essentially, farming is becoming more sophisticated with the help of AI. While much progress has been made, there's still a lot to do. As AI adoption grows, farm operators will benefit from increased access to a growing pool of knowledge about emerging practices and use cases, both industry-wide and worldwide.
"Our ability to collect and process data as well as the autonomy of robotic systems is rapidly improving. In the future, most manual tasks will be automated, reducing the amount of labor required to grow fresh produce," said David Colmenares, senior computer vision engineer at precision agriculture firm Iunu. "This will enable the grower to apply their expertise to higher-level tasks such as crop management and business administration."
There are autonomous robots and tractors mapping and preparing fields as well as platforms for pruning and harvesting, he added. Applying machine learning techniques to agriculture can be difficult given that a plot of farmland is a complex, living system. Therefore, it's critical to test and validate AI and machine learning systems in the field to collect as much data as possible and understand what outcomes drive the most value for growers.
"Building an ML system that correctly handles a wide variety of edge cases seen in the field requires a high degree of collaboration with growers," Colmenares said.
With each new AI advancement comes new opportunities for farmers to streamline their processes, increase efficiency, optimize their supply chains and increase profit margins -- all while decreasing waste and conserving resources. All of this will benefit food distribution, providing the public with a steadier supply of produce and increased food security.