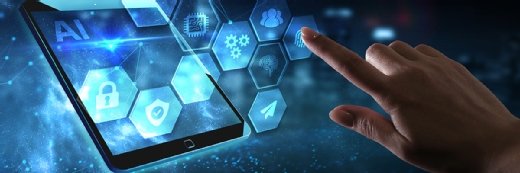
putilov_denis - stock.adobe.com
How AI in weather prediction can aid human intelligence
AI and machine learning models are becoming more widely used in climate prediction and disaster preparedness to aid experts without replacing them.
As more and more industry use cases for AI and machine learning come to fruition, weather and climate experts are utilizing these technologies to assist, but not replace, human intelligence.
Increasingly, AI models are improving weather, climate and disaster predictions. AI and machine learning models have predictive qualities not inhibited by human errors. Also, new workflows use better weather models to improve business outcomes, operations and responses to weather events.
These models are being used alongside or as alternatives to large physics-based weather and climate models and offer some compelling advantages, according to James Bednar, director of custom services at data science company Anaconda. Traditional physics approaches require enormous computing power and take a long time to run while AI and machine learning (ML) models can learn and map directly from observed data to predict future outcomes without simulating the underlying physical process.
"Such direct mapping can be much faster to calculate and, in principle, could account for physical processes that are not yet known or that are computationally prohibitive to simulate directly," Bednar said.
While traditional physics-based models still outperform AI models in practice, they operate at a tremendous computational cost that AI models can mitigate when used correctly. Currently, these AI models are applied to a variety of use cases to assist experts.
AI in climate and weather prediction use cases
Improving climate resilience. Delivering a project in association with the World Bank and government of India, farmers in India use an AI-powered weather advisory system from Cropin to optimize farming practices for seed, crop, nutrient and soil management. On this deployment, the company has digitized over 30,000 farm plots covering 77 crop varieties and increased farm revenue by 37%, according to Cropin founder and CEO Krishna Kumar.
Wind energy forecasting. Wind projects tend to be located distant from weather infrastructure. Classic numerical weather prediction models typically do not resolve this terrain very well, resulting in erroneous weather output predictions. ML techniques combine onsite meteorological observation data, historical data and data about turbine operations to keep the models from both overpredicting and underpredicting.
Generation demand. Temperature forecasts are one of the primary inputs that utility companies rely on when planning for energy demand. However, decision-making can be imperfect due to sources of error in prediction models. New ML approaches can learn to infer information that's not resolved by the numerical models to correct for these errors, according to Scott Mackaro, head of insights and innovation for weather forecasting platform Vaisala Xweather.
Predicting power outages. AI vendors can train AI algorithms on satellite and weather data to predict the impact of storms and mitigate outages. Satellite AI can also help utility companies understand weather paths and the severity of incoming storms to minimize damage, plan a response, restore safety to communities and communicate accurately with response teams and local authorities.
AI can assist disaster preparedness
As one of many examples, aerial imagery technology and location data company Nearmap combines satellite imagery from as far back as 2014 with data about individual properties such as building and roof characteristics, surface permeability, roof condition, nearby construction and building footprints, as explained by Nearmap. This technology allows users to see predictive insights, like levels of tree cover in proximity to buildings, that could result in increased vulnerabilities caused by fire spread or damage from fallen trees in the event of wildfires or hurricanes.
"AI enables the ability to see aerial imagery of locations over time, which can help predict an area's state of preparedness for future weather events," a Nearmap spokesperson said.
AI models can even analyze roof types and conditions to determine if a roof can withstand certain types of wind events or if it should be replaced. By analyzing imagery captures over time, they can understand the condition of a house before a weather event occurs to see the actual damage. This data can be beneficial for post-catastrophe insurance claims processing.
AI and ML models help utility companies plan, prepare and reduce risks and outage times caused by storm impacts and wildfires. These models account for how infrastructure has responded to past storms, including differences in network hardening. This helps utilities position crews to reduce outage times. In the case of wildfires, AI models can ingest wildfire variables to forecast when and where wildfire conditions will exist to help determine fire risk. Some utilities include additional decision factors to help mitigate wildfire risks, such as response times for firetrucks or whether to perform work at a specific location on a particular day.
Finally, AI is being used to process weather, traffic and electronic vehicle trackers to provide shipment recommendations to customers, as evidenced by Delbert Cope, chief technology officer at supply chain visibility platform FourKites. If severe weather is expected to impact a specific leg of a journey, transportation managers can see that in advance and proactively reroute a shipment. The models' output can be used to determine optimal locations for sourcing required goods and routes that will avoid further disruptions. Properly trained models can also trigger inventory moves in preparation for a weather event to ensure operations continue.