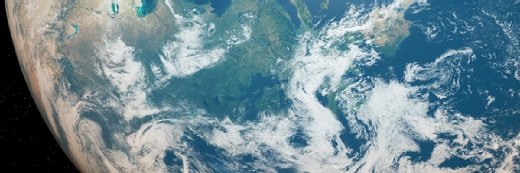
Getty Images
How machine learning can combat climate change
Machine learning can produce sustainability insights and help plan effective climate action. But researchers face challenges around data, compute and AI's own environmental impact.
As the climate crisis intensifies, AI is emerging as a tool for climate action, including modeling, prediction and optimization.
Machine learning models can provide environmental data insights, simulate changing climate patterns and even autonomously take actions that promote sustainability. At the 2023 ClimateTech conference, hosted by MIT Technology Review, a set of sessions highlighted AI's potential to combat climate change through use cases such as energy optimization and extreme weather prediction.
AI overall has seen a massive uptick in interest over the past year, and climate applications are no exception. In her presentation "Tackling Climate Change with Machine Learning," MIT assistant professor and Climate Change AI co-founder Priya Donti noted that venture capital investment in climate tech reached an all-time high at the end of last year.
Likewise, Climate Change AI Executive Director Maria João Sousa said in her talk "Fighting Fire with Firetech" that her organization is receiving growing interest from tech professionals, with more than 10,000 individuals from 165 countries registering for the nonprofit's recent summer school program. "We really see an immense demand for this," she said.
Despite excitement around AI-enabled climate breakthroughs, however, the field is still in its early days, and climate models -- like other forms of machine learning -- require extensive data and computing resources. Researchers emphasized the importance of collaborating to build trustworthy, accessible climate models and more environmentally sustainable technology overall.
"We have a choice," Donti said. "We can choose to shape the trajectory of how we develop technology in a way that is better aligned with climate goals."
Machine learning's complex effects on climate change
In her presentation, Donti discussed the many use cases for AI and machine learning in promoting sustainability, including her own research on power grid optimization. Overall, she sees these technologies aiding climate action efforts in several important areas:
- Information gathering, such as analyzing satellite and aerial imagery to track deforestation.
- Forecasting and simulation, such as using historical data and imagery to predict extreme weather events.
- Automated decision-making, such as optimizing buildings' heating and cooling systems based on weather conditions and usage patterns.
- Predictive maintenance, such as detecting and patching methane leaks in natural gas infrastructure.
- Novel scientific research, such as accelerating discovery of electrofuels, a more sustainable type of fuel created using electricity, water and carbon dioxide.
But Donti also voiced concerns about AI's ability to cause environmental harm. Although machine learning can support sustainability efforts and enable research breakthroughs, the process of training and running models has a significant environmental impact of its own.
"AI itself also has a footprint, both through the emissions associated with running AI [and] the computations of AI algorithms, as well as the hardware," she said. "This is not just an emissions footprint, but ... also a water footprint and a materials footprint."
And beyond the direct climate impacts of building and running models, there's the question of how AI's real-world use affects the environment. For example, Donti named actively damaging machine learning applications such as oil and gas exploration and extraction.
"I really do think that AI can be an accelerator of climate action and that it can really strengthen or support our progress on many climate change-related solutions," she said. "But I would be remiss to not mention the fact that AI is ultimately a general-purpose technology. It's one that's used in many ways across society, and not all of those are in support of climate action."
For companies, this means assessing the environmental benefits and harms of AI applications on a project-by-project basis, Donti said. This is particularly important in light of the rise of generative AI, including energy-intensive large language models.
But, she pointed out, not all forms of AI require the same energy investment as highly hyped forms of generative AI such as massive foundation models; in fact, some models are lightweight enough to run on a laptop. This flexibility might be preferable or even necessary for some businesses, as training and running large models also requires a more substantial financial investment.
"I think part of it is being smart about [things like], what do I actually need for this application?" Donti said. "Do I really need a huge, energy-intensive model? ... Don't limit your questions about AI emissions to climate change-related venues. You really should be asking these across AI contexts as a whole as well."
Using machine learning in the real world for wildfire management
Machine learning models can analyze large, complex data sets and perform simulations at a scale that would be extremely difficult or impossible for humans, enabling them to produce granular, localized insights and predictions -- an advantage for many climate science problems.
AI for wildfire management is an emerging area of interest, with successful applications in fire-prone regions such as California and Canada. In her presentation, Sousa gave examples from her own research, which aims to detect wildfires as early as possible by using intelligent sensor networks and AI to analyze data such as thermal imagery.
Sousa noted that the details of wildfire detection and management need to be tailored to the diverse characteristics of specific regions, making machine learning an especially useful tool. These differences across regions highlight the need for an integrated approach that combines prevention, prediction, monitoring and suppression.
When it comes to analysis and detection, for example, "in terms of what technology would work best ... for different fires, the answer would be different," Sousa said. Likewise, monitoring might be a higher priority than suppression for wildfires in remote regions that are unlikely to endanger humans.
Addressing climate change is a cross-disciplinary problem
The tech sector, of course, has an essential role to play in developing AI applications that promote sustainability. But speakers also emphasized that combating climate change with machine learning must be a collaborative effort that represents voices from a wide range of sectors and backgrounds, including technologists, scientists and policymakers.
In his presentation "A Digital Twin for Weather and Climate," Nvidia senior product manager Ram Cherukuri emphasized the importance of cooperation and cross-disciplinary input in evaluating and improving the still-nascent field of climate modeling. In particular, he noted that climate data gaps remain a challenge and called for collaboration among the scientific community.
"Right now, as we go about these problems, we feel a big void in terms of the number of participants from the climate science community," Cherukuri said. "It's not that they're not there -- it's just we need to make sure that these tools are accessible to them."
On a practical level, this can be as simple as making sure that individuals with programming and IT skills create and maintain applications that climate scientists can use without issue. By building practical and reliable tools, technologists can support and accelerate scientists' research -- for example, by enabling them to run faster, more accurate weather simulations.
"We don't want [scientists] to be spending time on, 'Oh, how do I get this thing installed? How do we run it, and how do we even get started?'" Cherukuri said. "Scientists should be doing science-related experiments."