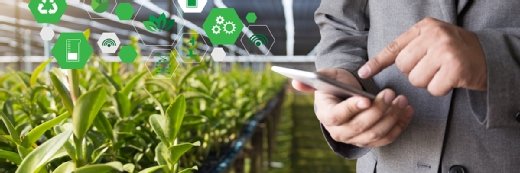
adiruch na chiangmai - stock.ado
How AI can be used in agriculture: Applications and benefits
The use of agricultural AI optimizes the farming industry by decreasing workloads, analyzing harvesting data and improving accuracy through seasonal forecasting.
Agriculture has constantly improved with the introduction of various technologies, from motorized equipment to biotechnology. Following business trends, the agricultural industry is looking to maximize efficiency by turning to AI technologies. AI technology has been implemented to help yield healthier crops, reduce workloads, organize data and improve a wide range of tasks in this $5 trillion industry.
Let's examine some of the applications and benefits of this technology in an agricultural setting.
IoT powered data analytics
Market research by Business Insider predicts the number of data points gathered on an average farm will grow from 190,000 today to 4.1 million in 2050. The volume of data collected -- through technologies like farm machinery, drone imagery and crop analytics -- is too abundant for humans to process. Farmers and agricultural technology workers are turning to AI to help analyze data points, thus enhancing the value derived from these data sources.
With the implementation of agricultural AI, farmers can analyze weather conditions, temperature, water usage and soil conditions collected from their farm to make informed decisions on business choices -- like determining the most feasible crop choices that year or which hybrid seeds decreased waste. Big data analysis also determines optimized irrigation, helps reduce greenhouse gas emissions, and pinpoints the exact soil, light, food and water requirements necessary for propagation.
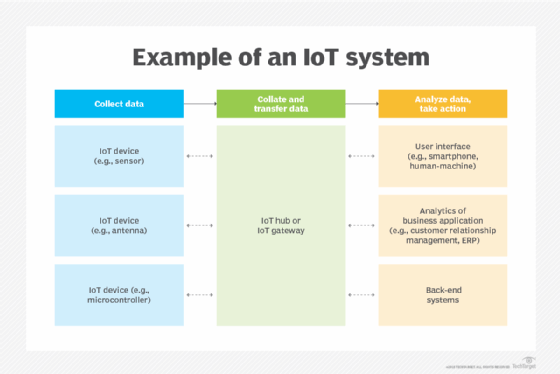
In addition to ground data, computer vision and deep learning algorithms process data captured from drones and unmanned aircraft systems. AI combined with unmanned aircraft systems and drones can capture images of the entire farm and analyze them in near-real time -- monitoring and analyzing soil health and the condition of crops across the entire farm, as well as identifying problem areas.
Predictive analytics and precision farming
Using AI systems to improve harvest quality and accuracy is a management style known as precision agriculture. PA uses AI technology to aid in detecting diseases in plants, pests and poor plant nutrition on farms. AI sensors can detect and target weeds while deciding which herbicides to apply within the right buffer -- preventing overapplication of herbicides and herbicide resistance.
Farmers are using PA to improve agricultural accuracy by creating probabilistic models for seasonal forecasting. These models can look months ahead and use data collected to provide farmers with base predictions for the most suitable crop varieties for the season, ideal planting times and locations. Agricultural AI technologies can then optimize farm management by basing decisions on predicted weather patterns during the coming season.
Risk management
Precision forecasting is the backbone of another agricultural AI tool: risk management. On its own, machine learning and AI are wonderful tools for reducing error in businesses process, and farmers are taking advantage of forecasting and predictive analytics to reduce the risk of crop failures.
Growing a sustainable crop in mass quantities requires a farmer to take enormous financial risks that rely on razor-sharp agricultural yield estimates to fulfill supply chain orders.
While climate and weather can only be predicted to a small degree, there are many other variables that can be controlled on farms. Plant population, plant strain, irrigation, soil preparation and pest control are being managed by harvesting IoT data to populate predictive algorithms that drive decisions for the year's harvest.
Risk management offerings and algorithms are used to determine economic trends, commodity prices, supply chain management, if a farmer should use government insurance and minimum yield requirements.
Pest control
Pest control companies are using AI to automate and improve everything from pesticide route planning, to spray times and pest prediction. By using drone technology, agricultural farmers and pest control companies can virtually walk every crop and provide nearly full-time monitoring to look for irregular crop degradation, pests, disease spots or dead soil. A farmer can then collect data from a specific crop area and stop the spread of disease.
Blue River Technology, a subsidiary of John Deere, is working with Facebook AI and machine learning to create camera-enabled machines that use image recognition technology to label weeds at point of contact and immediately remove or spray them.
Agricultural robotics and digital workforce
Traditionally, farms have needed many workers -- mostly seasonal -- to produce and harvest crops. However, fewer people are entering the farming profession due to the physical labor and high turnover rate of the job. Furthermore, most agricultural efforts use highly mobile migrant labor, which presents challenges for a stable and predictable workforce.
AI solves critical farm labor challenges by augmenting or removing work and reducing the need for large numbers of workers. Agricultural AI bots are harvesting crops at a higher volume and faster pace than human laborers, more accurately identifying and eliminating weeds, and reducing cost and risk. AI farmers present a permanent solution for the unpredictable and fluctuating agricultural workforce.
The crux of smart farming is using a blended workforce of digital help alongside traditional farmers and tools. Land O'Lakes deployed a line of smart tractors that use data insights to remotely plant seeds in the most optimized way. Predictive analytics data is being remotely applied to inform not only the farm, but the machinery.
After the seeds are planted, IoT devices continue to monitor growth, weeds, soil and water retention, and other factors which in turn inform next year's crop. Instead of relying on human measurements and labor, automated food and irrigation systems ensure the crops have the proper nutrients.
Additionally, farmers are taking advantage of chatbots to seek advice and recommendations on specific problems. Chatbots are already used in numerous industries with great success, so it's no surprise that AI-powered chatbots should help farmers as well. Agricultural AI and cognitive technologies enable farms across the world to run more efficiently to produce the fundamental staples of our dietary lifestyles.
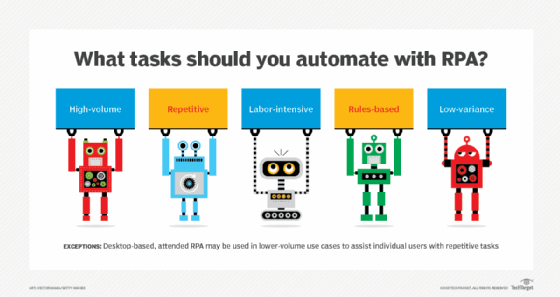
Future of AI in agriculture
As global population size increases, farmers now have to produce more food to feed a growing community, and the introduction of robotics and a digital workforce can offer automated assistance.
Genetically modified ingredients and food products promise customers access to fresh seasonal food year-round, which means farms have to depend on data to create longer seasons, bigger fields or different grow times.
The future of AI in agriculture will need a major focus on universal access, as most cutting-edge technologies are only used on large, well-connected farms. Increasing connectivity and outreach to even small farms in remote areas across the world will cement the future of machine learning automated agricultural products and data science in farming.