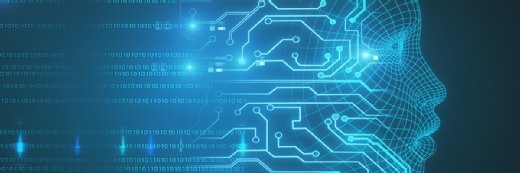
peshkov - stock.adobe.com
AI vs. predictive analytics debate shows powerful combination
Artificial intelligence and machine learning, when combined with predictive analytics, allow companies and organizations to get the most out of their data.
The debate between predictive analytics and artificial intelligence shows the how intertwined these technologies are. Splitting hairs between the two shows that these terms are hierarchical and that when combined, they complete one another in the enterprise.
Deciphering the differences between AI and predictive analytics comes down to applications. AI operates as an umbrella term for numerous other technologies, while predictive analytics is focused on specific applications.
At the core of AI
Artificial Intelligence is the replication of human intelligence by machines. This includes numerous technologies such as robotic process automation (RPA), natural language processing (NLP) and machine learning.
These diverse technologies each replicate human abilities but often operate differently. RPA is the use of AI and machine learning to handle high-volume tasks that can easily be repeated.
NLP is defined by a computer program that is able to understand human language as it is spoken. This subset of AI has moved past requiring humans to use a programming language with its precision and structure in order to communicate with it.
Machine learning is a form of AI that allows software applications to become progressively more accurate at prediction without being expressly programmed to do so. These algorithms are where predictive analytics and AI converge.
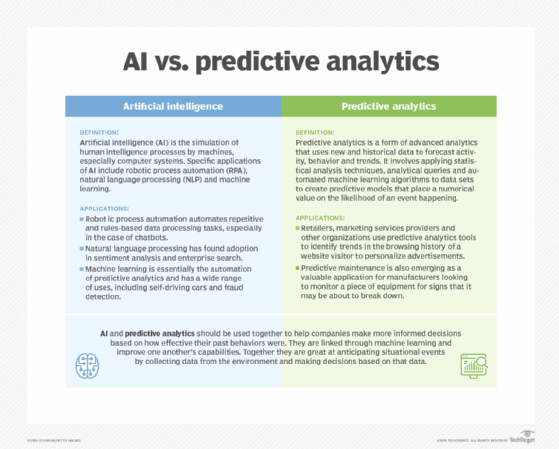
Predictive analytics and AI
As a form of advanced analytics, predictive analytics uses new and historical data in order to predict and forecast behaviors and trends.
Software applications of predictive analytics use variables that can be analyzed to predict the future likely behavior, whether it is for individuals, machinery or other entities.
"Analytics gives data meaning. Internally, that means enabling companies to be better at whatever they do," Roman Stanek, the CEO of GoodData, said. "Externally, it means extending the power of the data to drive new revenue streams and opportunities, not only for the company, but for that company's customers, partners, their entire business network."
Machine learning has the ability to increase the speed at which data is processed and analyzed and is a clear candidate through which AI and predictive analytics can coalesce. One of the smartest AI technologies, machine learning does as its name suggests and learns from the data it trains on.
Through this process, AI can train on even larger data sets and perform even deeper analysis. This ties into the need for business to gather the most amount of data possible while maintaining its quality.
"Best practices are critical when it comes to data, as critical as quality control in manufacturing or drug testing or any other venture," Stanek said. "No one should be loose about data privacy, security, data cleanliness or timeliness because all of that impacts the downstream worth of the incoming data."
Maintaining best data practices as well as focusing on combining the powers of AI and predictive analytics is the only way for organizations to keep them in a good position.