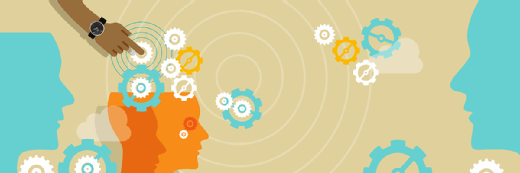
Combining AI and predictive analytics crucial for the enterprise
Predictive analytics, when combined with artificial intelligence, can assist organizations with their risk management, as well as their planning and optimization.
AI-enabled predictive analytics enables companies to make better decisions based on how effective their past behaviors were.
Since the advent of modern computing, organizations have been using their data to augment their decision-making processes. Predictive modeling has become a standard when it comes to anticipating your enterprise's future needs, such as inventory stocking, purchasing and trends of all sorts.
However, as more advanced tooling has become available, companies are increasingly using machine learning to further harness the power of predictions.
How AI is boosting predictive analytics
While companies have been using data to make predictions for decades, the use of machine learning, statistical algorithms and advanced modeling have enabled companies to be able to process more data than ever before.
Industries ranging from insurance and financial services to travel and hospitality are finding benefits from predictive analytics. Predictive analytics helps reduce risks, optimize operations and increase revenue. Companies are now able to gain insights more quickly than before and can be proactive rather than reactive in decision-making.
With any AI system, the goal is to get the machine to process data quickly and to be able to analyze data more efficiently. The ultimate application of predictive analytics is to look for patterns in data sets, see how those patterns have developed over time and then use that information to predict future trends.
The goal is to ultimately help humans make better decisions based on what the computer predicts to be the likely outcomes for the future. Since this is the fundamental goal, there are lots of applications that this pattern has in the real world.
Applying predictive analytics
For companies looking to derive benefit from predictive analytics, there are a few approaches that can provide benefit. Classification models are fairly simple but can provide significant value. They are best used when asking simple, yes-no questions. Companies are able to take their historical data and have the model make a decision based on that data.
Another way companies can apply AI-enabled predictive analytics is through clustering. Clustering models sort your data into separate groups based on similar attributes. In this unsupervised learning approach, the model works on its own and learns to sort, or cluster, your data. This approach sees computers cluster data in ways humans may not think to do, resulting in potentially unique insights.
Forecasting is also a popular way to apply the predictive analytics pattern. Forecasting models can be applied to historical numerical data to help make predictions. One of the most common use cases is weather forecasting, but it can also be used for predicting how many customers will convert, how many people are expected to enter a store in a given hour or how much inventory should be kept in stock.
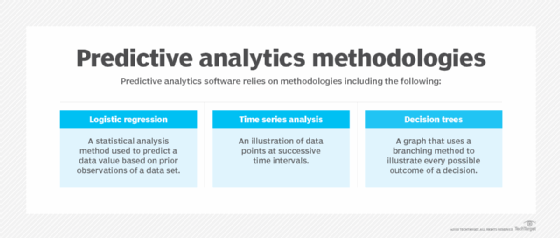
Use cases of AI-enabled predictive analytics
The retail industry in particular has found great value in AI-enabled predictive analytics for a variety of applications in forecasting and planning. Before the start of every new season, retailers base their demand estimates on a variety of factors, including prior-year sales, intuition and industry knowledge.
However, the world is an unpredictable place with innumerable factors that have to be weighed. AI-based approaches help ease the burden and limit forecasting errors, saving the company money.
Predictive analytics is also helping with logistics and supply chains. It can help predict what type of staffing is going to be necessary for certain months or seasons based on past data. AI-enabled predictive analytics also helps companies better understand how to optimally deliver products and how different environmental factors may influence factors like delivery times or demand for certain products. And it can also be used to predict what types of inventory need to be supplied, reordered or discontinued.
In recent years, meteorologists have turned to forecasting models and advanced predictive analytics to help improve predictions. These models utilize satellite imagery, past forecasts, temperature changes and overall historical patterns for a geographic area to make their predictions. This large amount of data, with the help of machine learning, can create models that are more accurate than ever before.
In fact, a modern five-day forecast is as accurate as a one-day forecast was in 1980, according to a report published in Science. Being able to know weather patterns days in advance can help companies better divert or reroute shipments and provide customers more immediate notifications of any delays or issues with orders.
For many companies, AI-enabled predictive analytics solutions are helping to optimize processes and resources, while simultaneously reducing costs and improving efficiency. Given machine learning's remarkable ability to spot patterns and identify trends and its ability to process large amounts of data quickly and identify patterns, it should come as little surprise that predictive analytics will continue to see widespread adoption.