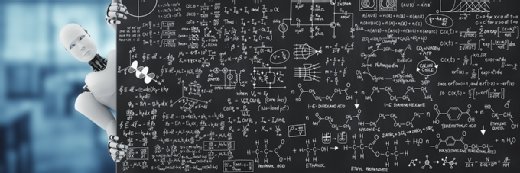
Blue Planet Studio - stock.adobe
What companies are getting wrong about AI
Incorporating AI successfully means reimagining the organization. Learn how a phased approach can combat structural challenges and foster cross-team collaboration and innovation.
A medium-sized agritech company was stuck. It had teams of agronomists across the globe helping farmers optimize the raising of their crops, but the teams were stagnating in silos.
An agronomist in one area often had questions about issues that a colleague elsewhere could address -- for example, "How might this unexpected weather pattern in my area affect weed growth?" But unless the agronomist personally knew the right person, getting the question answered took a lot of time. Most people just didn't bother.
For a while, AI -- particularly in its latest form, generative AI -- was touted as an easy fix. But following what most businesses have done so far and merely injecting AI into the company by asking employees to use OpenAI's ChatGPT or Microsoft Copilot "out of the box" does not work.
While generative AI can answer broad human-generated questions, it cannot provide answers to queries that require deep domain experience or connect people to the requisite expertise within the company. Unless a colleague has actually written and distributed a paper on how specific weather patterns affect weed growth, the agronomist is still out of luck.
Generative AI has captured the imagination of much of the business world. The initial buzz in 2022 has worn off, but executives and entrepreneurs continue to explore how companies can realize the tremendous potential of this breakthrough. But, as the agritech example shows, leaders must also grapple with the structural impediments to AI adoption.
3 key phases to AI adoption
Our collective research on AI adoption as well as executive experience in implementing AI systems at a wide variety of companies has convinced us that piecemeal approaches are likely to fail. Based on our research and experience, we recommend three key phases to AI adoption that organizations, such as the agritech company described above, must follow to realize the full benefits of AI.
First, it's important to understand the current capabilities of generative AI. ChatGPT can understand natural human language and has some visual analysis capabilities as well. Instead of contorting their queries into machine-understandable language, usually with software and other structures, users can now ask questions and provide directions in their natural language and get (usually) reasonable answers in that same language, within a few seconds. This is phase one, which -- although important -- is not enough to tackle the most pressing business problems. Those problems require correct, concise and consistent answers, something current AI is still not able to achieve.
Second, in a business context, it is critical to understand a questioner's intent. What does the person inputting the question really want to know? What's the context for the question? What would best help the questioner? This is phase two, which requires going beyond the current generative AI models and infusing them with expertise in the form of rules relevant to the business.
Several examples of such composite AI systems are beginning to emerge. To illustrate, consider the rental car business. Suppose a manager asks for a comparison between gasoline-powered cars and electric vehicles, not simply to gain knowledge but to assess the relative merits of these vehicles in the context of a potential purchase.
The AI would start by checking whether purchasing cars was the true intent, then suggest purchasing a hybrid car given the low density of charging stations in the vicinity (or, as Hertz realized only too late, higher-than-expected costs of maintaining electric vehicles). Doing so requires a layer of algorithms that reason with the large language model's current data on the user's activities and interactions.
Phase three, in which businesses can achieve the full value of AI, requires senior executives to drive AI adoption and collaboration across the organization. Executives have been talking about breaking down silos for a long time, but most organizations are still separated into largely impermeable groups. It's the old lament of leaders: "If only we knew what we know." Even AI can't solve this problem alone -- it will just make each silo more efficient.
To realize the full potential of AI, companies need to rethink their organizational structures. They need to envision a central AI system that reaches all parts of the organization. That way, it can draw on unstructured data from across the company and send it to the one group that needs it now. Rather than just talk about broad collaboration, companies can put AI in the center. This view is now beginning to reach broad consensus among executives in leading businesses, such as Snowflake and Accenture.
AI will, therefore, not just enable greater efficiency in existing collaboration -- for example, reducing the need for annoying company-wide announcements or large meetings to ferret out knowledge -- but also foster collaboration on issues that most people do not currently discuss with colleagues at all. However, a couple of reminders are in order.
Although AI has enormous potential, it does not work well in all interactions. Employees will continue to be better placed to handle exchanges that build trust, relationships and judgment, as opposed to transactional requests for knowledge. AI might play a supporting role, but only human beings can build real trust.
Second, as companies establish AI, they will need to rethink how to measure and reward individual performance. They must encourage people to make emerging knowledge available to the central AI platform.
Thought leaders have described AI as a revolutionary change, on par with electricity. While information about the apparently miraculous feats of AI inundates our newsfeeds every day, businesses have struggled to adopt it and drive real value meaningful to them. We believe that a systematic, phased approach to AI adoption that increasingly drills down from an information-systems focus into underlying organizational structures can drive genuine AI-enabled value creation in organizations.
Ram Bala is an associate professor of AI and analytics at Santa Clara University and co-founder and chief AI scientist at Samvid AI. He is a researcher, leadership coach and entrepreneur who has been focused on applying AI to pricing, marketplace design and supply chain management over the last two decades.
Natarajan Balasubramanian is a professor of AI strategy at Syracuse University and a leading researcher on AI and machine learning adoption in organizations.
Arun Rao is CEO and co-founder of Samvid AI. He is an entrepreneur and seasoned Silicon Valley executive with previous technology leadership roles at The Gap, C.H. Robinson and Flex.
Amit Joshi is a professor of AI, analytics and marketing strategy at IMD. An award-winning professor and researcher, he has extensive experience with AI and analytics-driven transformations in industries such as banking, fintech, retail, automotive, telecoms and pharma.