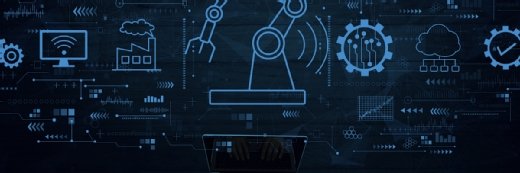
Getty Images/iStockphoto
AI a top trend for supply chain, but experts urge caution
AI is being adopted for company supply chains, but organizations should guard against hype and assess the business value and feasibility of these applications.
Adoption of AI has become a significant trend for supply chain technologies, but organizations should be wary of overhyped promises.
AI, including generative AI (GenAI), is an integral component of several supply chain trends for 2024 identified in a recent report from Gartner. The annual report coalesces research by several Gartner analysts to provide recommendations for supply chain leaders in planning supply chain technology strategy and selection.
One trend, for example, is the development of what Gartner calls composite AI, which is defined as "the combined application of multiple AI techniques" to solve business problems that can lead to supply chain performance improvements, according to the report.
Other trends in the supply chain report that are underpinned by AI include AI-enabled vision systems, augmented and connected workforces, machine-centric procurement, next-generation humanoid robots, cyber extortion, supply chain data governance and end-to-end sustainable supply chains.
One of the report's authors, Dwight Klappich, research vice president at Gartner, said it's important to remember that AI is not one thing, but a portfolio of advanced analytics techniques that organizations can employ to solve problems.
"We sometimes dismiss things because we only want to talk about AI for optimization," Klappich said. "There are places where heuristics still make sense or where traditional combinatorial optimization is the best mechanism to solve a particular problem. It's not just about all the hype around GenAI."
Machine learning (ML) is a traditional AI category commonly being embedded in applications such as forecasting, demand planning and labor planning, he said.
"Some vendors are using machine learning algorithms to do that," Klappich said. "Today, people may be doing a lot of spreadsheets with seat-of-the-pants algorithms, but they've exhausted those, so they're looking for ML to do that."
GenAI gets the hype now, but what it's good at -- creating content from large language models -- doesn't have as much use in supply chain processes yet, he said. GenAI is being used for applications such as intelligent chatbots that can query logistics systems to flag late orders or other issues.
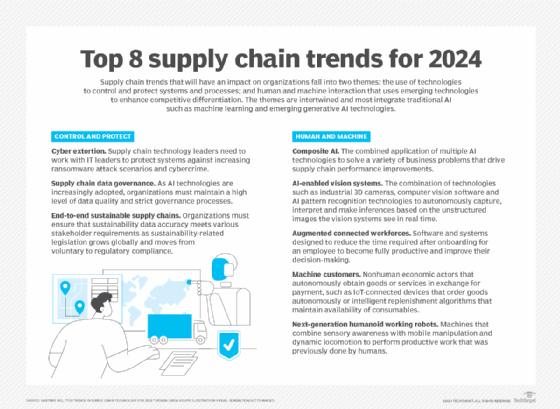
Build or buy for AI
The questions now for supply chain leaders are how early they want to get in on AI and whether they should build their own applications or use AI-embedded applications, Klappich said.
"There's always going to be companies that want to build their own thing," he said. "But most companies right now are saying that if they're looking at buying applications, they want to know that the vendor is building around the most contemporary technology that will give them the best solution overall."
The adoption of AI-enabled applications in supply chain management also depends on an axis of the feasibility of the application and its business value, Klappich said. For example, using autonomous trucks in transportation management has been talked about for years, but it's not necessarily feasible because of safety concerns or high implementation costs. On the other hand, organizations are already using predictive arrival times in transportation management, as it ranks high on both the feasibility and business value scales.
"That uses machine learning based on conditions to figure out if something was supposed to be here on Tuesday by noon, but won't arrive until Thursday," he said. "Some things like damage detection or visual inspection with AI-enabled vision systems are [also] feasible."
Beware of bright, shiny objects
Simon Ellis, an analyst at IDC, agreed that the growth of traditional and GenAI adoption is a supply chain trend and that organizations should be realistic about the hype around these technologies.
"I worry about getting too seduced by the bright, shiny object," Ellis said.
Nevertheless, organizations are interested in AI for supply chain and are beginning to plan for implementation, he said. To this end, Ellis has found that there are three basic questions coming up in AI discussions from supply chain technology buyers.
The first is around data and whether an organization's internal data is good enough and comprehensive enough for effective AI. The second is whether they should think of AI broadly -- and GenAI specifically -- through embedded use cases in traditional supply chain applications or as a layer to build applications on. Third is whether companies should be early adopters to gain first-mover advantage, or be fast followers or second movers on AI applications.
"There are no easy answers for these, and [answers are] going to vary by company," Ellis said.
The supply chain AI use cases are coming, he said, as AI will be a trend that characterizes this year.
"Most of the early use cases are productivity-related for things like processing documents more efficiently or figuring out logistics," Ellis said. "It's accelerating time to expertise, making people more productive faster, or having automation and AI do the routine, rote tasks to help people be more productive and efficient."
One of the concerns that people have around the rapid increase in AI-enabled applications is that AI will replace the people doing the work, but Ellis maintained that these concerns are overblown.
"Yes, there are certain jobs that get replaced with technology, but there are a whole lot more that get created as a result of technology," he said. "It might not be all one-for-one, but in aggregate, there are more jobs, not fewer jobs."
A more immediate concern is that people will need to know how to use AI applications to get the best results from them, Ellis said. Using AI applications will require superior critical thinking skills to discern if AI-supplied recommendations pass a commonsense test. For example, if an AI-enabled forecasting application recommended producing 10,000 of something this year, while the company made 100 the previous year, the output would need further investigation.
"Maybe the tool was using bad data or was conflating things, because it doesn't know until it knows," he said. "These are learning systems, and they're narrow learning systems. They have a very specific role, and they're only as good as the data they're trained on."
Jim O'Donnell is a senior news writer for TechTarget Editorial who covers ERP and other enterprise applications.