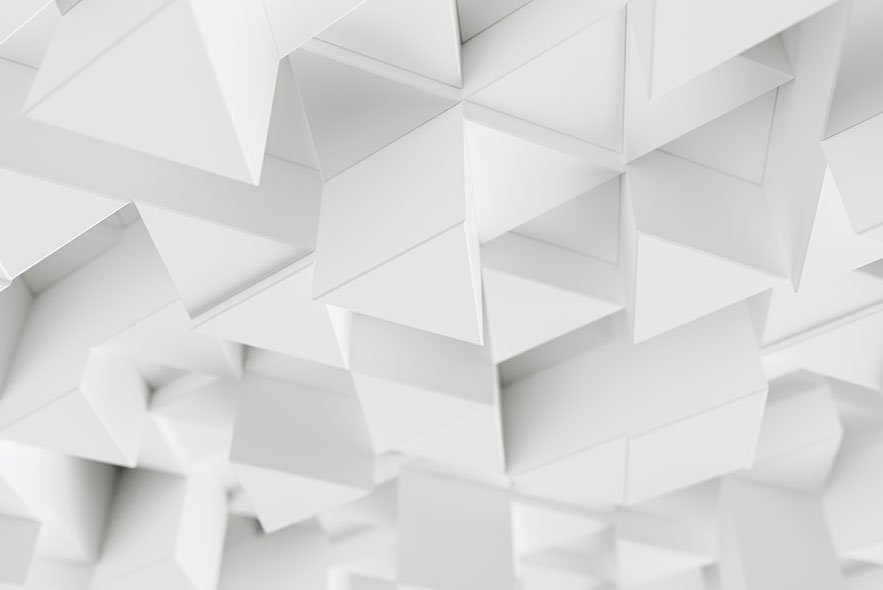
What is user behavior analytics (UBA)?
User behavior analytics (UBA) is the tracking, collecting and assessing of user data and activities using monitoring systems. UBA is commonly referred to as user and entity behavior analytics (UEBA) to reflect that users are just one category of entities with observable behaviors on modern networks. Other entities include processes, applications and network devices.
UBA and UEBA technologies analyze historical data logs, including network and authentication logs collected and stored in log management and security information and event management (SIEM) systems. This is done to identify patterns of network traffic caused by the behavior of users, both normal and malicious. These systems provide cybersecurity teams with actionable insights when the systems detect unusual behavior.
While UBA and UEBA systems don't take action based on their findings, they can be configured to automatically adjust the difficulty of authenticating user accounts that show anomalous behavior or otherwise deviate from normal behavior.
What is UEBA and how does it differ from UBA?
The terms user behavior analytics and user and entity behavior analytics differ in three primary ways:
- UEBA is newer. In 2015, analyst firm Gartner published a market guide for what it coined as user and entity behavior analytics.
- UEBA systems track more than just user activity. UEBA tracks the activity of devices, applications, servers and data. Instead of analyzing only user behavior data, this technology combines user behavior data with behavior data from entities.
- UEBA takes things a step further. UEBA systems produce a higher volume of data and provide more complex reporting options than original UBA systems.
User and entity behavior analytics technologies have the same capabilities as traditional UBA, but UEBA systems use more advanced analytics techniques. While UBA is designed to track insider threats, UEBA is designed to use artificial intelligence (AI) and machine learning to look for more types of anomalous activities associated with a broader range of threats, including advanced threats, that can obscured by legitimate network activities. UEBA has multiple enterprise use cases and is often used in conjunction with SIEM technologies to better analyze information.
This article is part of
What is data security? The ultimate guide
How UBA and UEBA systems work
Both UBA and UEBA systems collect various types of data, including user roles, titles, access, accounts, permissions, activity and geographical location. They also track security alerts. This data is collected from past and present activity. The analysis considers factors such as resources used, the duration of sessions, connectivity and peer group activity as part of anomaly detection capabilities. It also automatically updates when changes are made to the data, such as promotions or added permissions.
UBA and UEBA systems don't report all anomalies as risky. Instead, they evaluate the behavior's potential impact. If the behavior involves less-sensitive resources, it receives a low impact score. If it involves something more sensitive, such as personally identifiable information, it receives a higher impact score. This way, security teams prioritize what to follow up on while the behavior analysis system automatically restricts or makes it more difficult to authenticate the user showing anomalous behavior.
Behaviors that UBA and UEBA systems monitor are generally those associated with specific attacks or other security events. Monitored behaviors include the following:
- Brute-force attacks.
- Improper data access.
- Data loss.
- Lateral movement by unauthorized users.
- Questionable activities by privileged users who might be malicious insiders.
Machine learning algorithms enable UBA and UEBA systems to reduce false positives and provide clearer and more accurate actionable risk intelligence to cybersecurity teams. These systems also use threat intelligence feeds to augment and support machine learning functions.
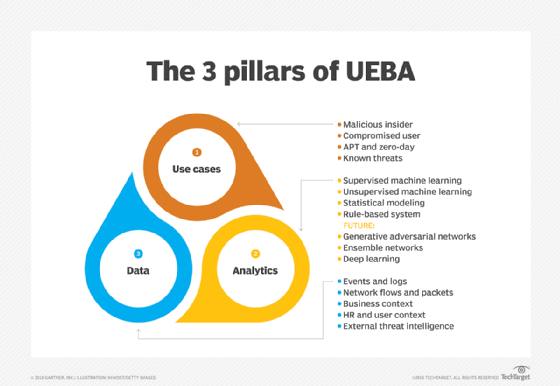
Benefits and drawbacks of UBA and UEBA
UBA and UEBA systems provide multiple benefits for enterprises with security capabilities that meet today's challenges. They include the following:
- Risks mitigation. Behavior analysis systems identify a range of internal and external threats and vulnerabilities to assist security professionals.
- Compliance. These systems keep enterprises in compliance with an evolving landscape of laws and regulations. They help organizations enforce security rules and alert security teams when policies are violated.
- Cost savings. Recovering from data breaches and other security incidents is costly for organizations. UBA and UEBA systems can spare them from these incidents and their associated costs. Also, these systems help reduce cybersecurity budgets, as organizations don't have to expend as much time or effort to identify threats or hire as many security experts.
Despite these benefits, these systems have drawbacks, such as the following:
- Human intervention. While fewer security professionals are needed, enterprises might need more skilled data analytics pros to interpret the complex data UBA and UEBA systems produce. People must ultimately draw conclusions about potential threats these systems identify.
- Additional tools. These systems inform security pros about potential threats and provide them with the data needed to defend their organization. However, they aren't equipped to stop such threats on their own. Other security systems and tools are required for that.
- Upfront costs. The short-term costs of purchasing UBA and UEBA systems are likely affordable for large enterprises, but smaller companies might consider these costs a barrier to using a behavior analysis system.
Why companies need UBA and UEBA
Behavior analysis systems first appeared in the early 2000s as tools to help marketing teams analyze and predict customer buying patterns.
Current user behavior analytics tools have more advanced profiling and exception monitoring capabilities than SIEM systems and are mainly used for two functions:
- To determine a baseline of normal activities specific to the organization and its individual users.
- To identify deviations from the norm. UBA and UEBA use big data and machine learning algorithms to assess these deviations in near real time.
While applying user behavior analytics to just one user might not be useful for finding malicious activity, running it on a large scale can detect malware and other potential cyberthreats, such as data exfiltration, insider threats and compromised endpoints.
How to implement UBA and UEBA tools
There are certain best practices enterprises should consider when implementing behavior analysis systems:
- Scope. An organization should define its goals for a behavior analysis system and the specific types of behavior the system is expected to monitor. This includes having the means of collecting the necessary data in place.
- User access. Team members who need permission to use the system and receive alerts must be identified and their accounts set up to reflect this.
- Additional tools. UBA and UEBA systems are for threat detection and analysis; they don't stop threats. Other tools, such as firewalls, perform actions needed to thwart such threats.
- Training. Employees must be trained to use these systems properly, including new hires.
- Monitoring and fine-tuning. Organizations need to continuously update UBA and UEBA systems to keep up with new types of threats and data use.
UBA and UEBA tools
The market for user behavior analytics tools continues to grow and evolve. The following are some of the leading UBA and UEBA products, according to Gartner analysis:
- Code42 Incydr.
- Forcepoint Insider Threat.
- Fortinet Security Operations Platform.
- IBM QRadar User Behavior Analytics.
- LogRhythm UEBA.
- Microsoft Defender for Identity.
- OpenText ArcSight Intelligence.
- Proofpoint Insider Threat Management.
- Rapid7 InsightIDR.
- Securonix UEBA.
- Splunk User Behavior Analytics.
- Varonis Data Security Platform.
Newer systems are able to integrate with data sources and the use of AI and machine learning to make correlations between data points and detect anomalies. In addition to SIEM systems, UEBA systems typically integrate with databases, data warehouses and data lakes. As the market continues to consolidate, UEBA and UBA functions are also increasingly incorporated into larger cybersecurity packages from leading vendors.
UEBA vs. SIEM vs. SOAR vs. XDR: Key differences in terminology and technology
UEBA products are just one way to address threat detection. Other related technologies include the following:
- SIEM. These systems collect and aggregate data from multiple sources to identify potential threats and issue alerts. SIEM systems are commonly used for detecting compliance issues and use statistical models to identify suspicious behavior patterns correlated with cyberattacks. However, SIEM products can overwhelm information security teams with a high volume of alerts, many of which might be false positives.
- Security orchestration, automation and response. SOAR systems improve on SIEM. They gather rich event data and use automation to identify potential threats and anomalies. SOAR systems must be integrated with security data sources and require significant effort from information security analysts.
- Extended detection and response. XDR is the latest generation of endpoint detection and response (EDR) and network detection and response (NDR) systems. EDR is focused on endpoints, such as end-user computers and organizational servers. NDR monitors network transmissions. XDR is converging with UBA and UEBA functionality, as well as with SOAR and SIEM.
Given the abundance of related technologies, there is considerable overlap among the different types of threat detection tools. UBA and UEBA are two of many tools in the cybersecurity toolkit. Which system is used depends on the organization's use cases.
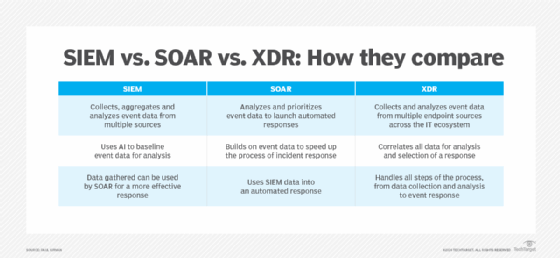
UEBA and SIEM technology: Why use them in tandem?
Many organizations are opting to use SIEM and UEBA together. Looking at the differences between these two types of systems highlights how they complement each other:
- UEBA systems focus on real-time data capture and analysis. SIEM systems examine event data over a set length of time.
- UEBA systems rely on machine learning to automatically flag potential threats as they're happening, while SIEM systems provide cybersecurity professionals a powerful tool to manually search for security threats.
- UEBA systems amass events from many different types of data, including logs and both structured and unstructured data sets. UEBA depends on machine learning to assemble evidence of threats and identify approaches to mitigating threats. In contrast, SIEM systems tend to have more structures imposed on the inbound data, which usually comes from structured logs.
- UEBA systems depend on risk scoring to compare different threats and to identify which systems are at risk and in what ways. SIEM systems depend on alerts and notices generated automatically when the system detects a pattern of activities that correlates with known attacks.
User and entity behavior analysis systems require data logs from log management and SIEM systems. Learn about security log management and logging best practices.