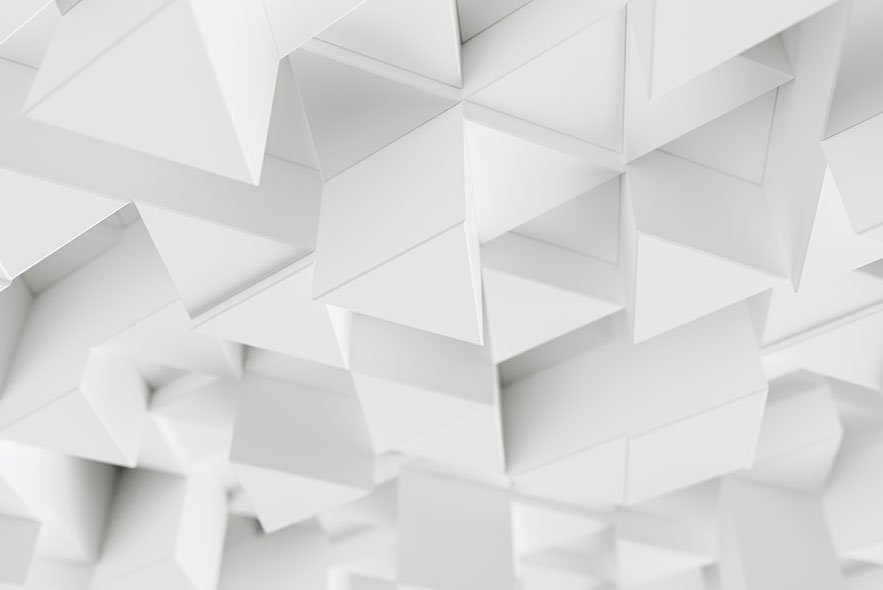
data lifecycle management (DLM)
What is data lifecycle management?
Data lifecycle management (DLM) is a policy-based approach to managing the flow of an information system's data throughout its lifecycle: from creation and initial storage to when it becomes obsolete and is deleted.
DLM products automate lifecycle management processes. They typically organize data into separate tiers according to specified policies. They also automate data migration from one tier to another based on those criteria. As a rule, newer data and data that must be accessed more frequently is stored on faster and more expensive storage media, while less critical data is stored on cheaper, slower media.
What are the 3 main goals of data lifecycle management?
Organizations are handling more data than ever, and that data might be stored on premises, at colocation facilities, in edge environments, on cloud platforms or any combination of these platforms. The need for an effective DLM strategy has never been greater, but the strategy must be a comprehensive one to be effective.
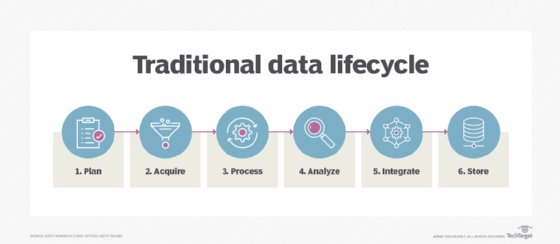
Many resources cite the following three goals -- or a close variation of them -- as the most important ones to achieve in an effective DLM strategy:
This article is part of
What is data security? The ultimate guide
- Data security and confidentiality. Data must be stored securely at all times to ensure that private, confidential and other sensitive information is continuously protected against possible compromise.
- Data integrity. The data must be accurate and reliable regardless of where it's stored, how many users are accessing or working with that data, or how many copies of the data are maintained.
- Data availability. Approved users should be able to access the data when and where they need that access, without disruptions to their workflows or day-to-day operations.
Data security and confidentiality have become increasingly important as organizations face the mounting body of compliance regulations such as the Sarbanes-Oxley Act (SOX), General Data Protection Regulation (GDPR), Health Insurance Portability and Accountability Act (HIPAA) and California Consumer Privacy Act (CCPA).
Data management experts stress that data lifecycle management is not a product, but a comprehensive approach to managing an organization's data, involving procedures and practices as well as applications.
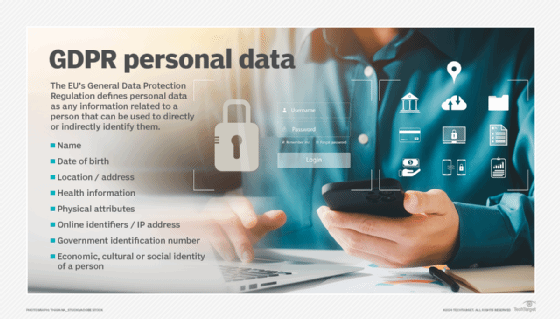
What are the main phases of data lifecycle management?
DLM can be broken into multiple phases that provide a framework for working with data throughout its lifecycle. Although different resources identify these phases in various ways, they often follow a structure similar to the following:
- Generate and collect data. Structured and unstructured data is continuously being created by users, devices, applications, machinery, IoT devices and other means. The way in which that data is captured depends on how it's generated and the types of data and applications. In some cases, not all generated data is collected. For example, machinery data might generate enormous amounts of sensor data, but only anomalous data is collected.
- Store and manage data. Data must be stored in a stable environment and properly maintained to ensure its integrity, security and protection. During this phase, the data is typically processed in some way, such as being encrypted, compressed, cleansed or transformed. This phase also makes certain that systems are in place to ensure availability and reliability and to implement redundancy and disaster recovery.
- Use and share data. Data is valuable only if authorized users can work with it as needed to carry out their day-to-day operations. During this phase, users access and modify data as needed and carry out other data-related operations, such as collaboration, business intelligence, advanced analytics or visualization. Data usage can also result in additional data being created, which must then be stored and perhaps further processed. In effect, this phase is what enables authorized users to be able to do their jobs.
- Archive data. At some point, data is no longer needed to support an organization's everyday applications and workflows, in which case, the data can be archived to a secure, long-term storage system such as tape storage or a cloud platform. The data might still be needed at some point for compliance, analysis, reporting or other purposes, which means it must remain available and viable, but it isn't required for daily operations. The data should also be fully protected, just like active data.
- Destroy data. When data has reached end-of-life, it can be permanently deleted, but it must be done securely and without violating applicable data protection regulations.
Not all DLM phases are strictly linear. As already pointed out, the third stage might result in additional data being generated. In fact, the first three stages often occur simultaneously, with data being continuously generated, collected, stored, managed and made available for authorized usage.
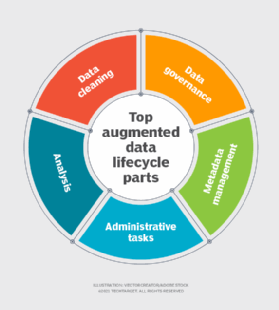
DLM and other systems
Hierarchical storage management (HSM) is sometimes confused with DLM, but HSM is only one type of DLM product. The HSM hierarchy represents different types of storage media, such as solid-state drives (SSDs), hard disk drives (HDDs), optical storage or tape systems. In this model, each storage type represents a different level of cost and performance.
Using an HSM product, an administrator can define guidelines for how often different types of files should be copied to a backup storage device. Once a guideline has been deployed, the HSM software manages everything automatically.
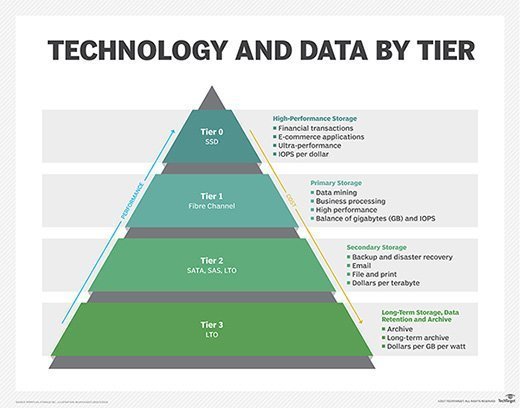
Another source of confusion is the difference between DLM and information lifecycle management (ILM). Although they're sometimes used interchangeably, they differ in important ways. According to Karen Dutch, who was once vice president of product management at Fujitsu Softek, DLM products deal with general file attributes such as type, size and age; ILM products have more complex capabilities.
For example, an administrator can use a DLM product to search stored data for a certain file type of a certain age. In contrast, the administrator can use an ILM product to search various types of stored files for instances of a specific piece of data, such as a customer number. This type of control has become increasingly important in the age of compliance regulations.
The GDPR, for example, guarantees an individual's right to be forgotten. An ILM product can help locate the individual's personal data, but a DLM product cannot.
Explore the benefits of building a strong data governance strategy