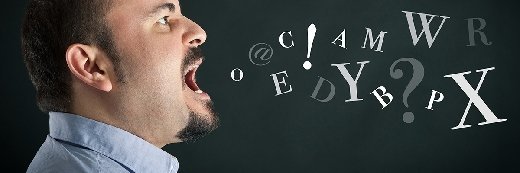
pio3 - Fotolia
NLG tools powered by AI energize, customize, optimize content
Though in its early stages, natural language generation automates content collaboration platforms to streamline employee communications and personalize customer engagement.
By using AI to dynamically generate content, natural language generation promises to automate many aspects of content collaboration platforms.
NLG applications include internal content for employee communications and external content such as personalizing product descriptions, customizing reports and generating simple news stories. It can also make messaging more targeted and personalized, which could increase customer engagement, optimize SEO efforts, streamline routine tasks and free up employees to focus on higher-value tasks.
"The sweet spot for natural language generation for content customization as it relates to creating, sharpening and perfecting content lies in its ability to generate hyper-personalized narratives at an unprecedented speed and scale," said Tyler Amos, principal engineer at NLG software provider Automated Insights Inc.
The technology is still in its early days, but NLG tools are getting better. Measuring good results requires an iterative approach to determine if the resulting narratives make sense, provide value to users and are more engaging than human-generated content. To streamline the process, companies also need to organize the flow of data from databases, content management systems and other sources.
"It is a solid technology, but it's not AI magic that can produce something entirely new, at least not today," said Michael Gerard, chief marketing officer at e-Spirit Inc., which has integrated NLG into its CMS.
NLG tools for data-driven content
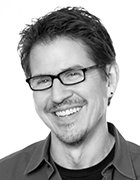
NLG can comprise data-driven, content summarization and content reorganization tools. Data-driven approaches use structured data organized into specially curated data sources to dynamically generate content like company reports, sports stories or product descriptions. Content summarization and rewording approaches craft new content from existing text.
Narrative Science's enterprise reporting platform Quill uses well-structured data and internal scoring algorithms to determine which facts are important to a targeted user. Dominion Dealer Solutions, for example, uses Quill to automatically generate vehicle descriptions from 15 different data sources, while the PGA Tour uses the platform to create player recaps for fans.
"The starting point is highly analytical, but the endpoint is natural language," noted Kris Hammond, chief scientist at Narrative Science, computer science professor at Northwestern and co-chair of the Illinois Technology Association's AI Council.
Hammond said two of the more important aspects of creating NLG applications are the elements that need to be included in a story and how to order those elements. Subject matter experts need to work with developers to separate the genuinely important from the less interesting elements, depending on the user.
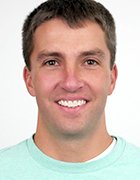
Narrative Science also examined ways to write reports on the performance of ad campaigns with the targeted audience. "Understanding what actions the reader will take is a substantial part of the issue," Hammond said.
Automated Insight's NLG platform Wordsmith automates simple news stories for the Associated Press -- allowing a smaller team to grow these types of stories from 300 to as much as 4,400 per quarter, according to Amos. Smart home services provider Vivint, he added, uses Wordsmith to boost SEO efforts and increase sales with localized website content -- doubling the amount of content to nearly 30,000 pages.
Rewording content and the human element
Although NLG tools can handle tasks like writing stories, they lack the human approach to understanding the underlying words. Text reorganization tools promise the ability to generate summaries of longer text or even reassemble new stories for curated content.
Qualex (QLX) Consulting Services used the text editor AI-Writer to help generate a selection of stories for an issue of Esquire Singapore. That process required considerable work in curating the content for these stories, according to Gary Stephen Jackson, a QLX data science executive director.
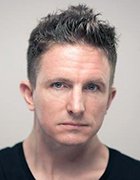
One challenge was minimizing bias. Jackson said, "To get it to learn different perspective and viewpoint," he explained, "I personally had to curate diverse data sets and written content that came from LGBTQ, Asian, women. The first passes at writing it sounded like a white bread accountant. Then when I started forcing it to learn from other data points, it began to have a snarky personality."
Software and language translation services provider Smartling developed an AI content authoring tool called Draft that's designed to simplify the content creation process for authors using style guides, a terminology base and predictive text that's reading from a database of previously drafted content. "This is so incredibly important, not only for creating source content, but also for translating all of this content into other languages efficiently," Smartling CEO Jack Welde said.
NLG content myths and realities
"A prevalent misconception is that natural language generation takes any data in any form and then reads, learns, understands and writes insights," Automated Insight's Amos said. NLG requires clean, structured data. Companies can struggle to get NLG off the ground quickly because their data needs to go through a rigorous cleaning process first. Perfecting the automated narratives can also take time to set up.
Amos recommended starting with existing data-driven stories that are generated manually. The data for generating text should be cleaned, structured and free of errors, which are the primary cause of a faulty narrative.
Another misconception is that NLG thrives on vast amounts of data. Often, this approach can result in too much content. "No one wants to read all the data, just the important things," Narrative Science's Hammond reasoned.
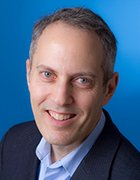
In addition, automatically generated content runs the risk of being repetitive and boring for applications like company reports. One way to counteract that problem, Hammond said, is to present similar things in slightly different ways so that users don't feel like they're reading the same report.
It's also important for managers to collaborate with writers and editors to devise and build out a good NLG process. "Editors need to accept NLG as contributors or almost co-workers," e-Spirit's Gerard said. NLG tools, for example, can create a sports report based on a huge set of available metadata such as who played and who scored, but only a journalist can get a quote from the coach or capture the emotion of the fans. Also, skilled writers are needed to train NLG to produce better, more lively texts.
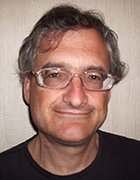
Then there's the issue of quality assurance. "Problems in requirements and QA are the most common reasons for failure of NLG projects to deliver value," said Ehud Reiter, chief scientist at Arria NLG and computer science professor at the University of Aberdeen. He pointed to an application designed to summarize end-of-shift reports that was ultimately abandoned because the generated reports weren't useful enough to the nurses. An agile approach that focuses on prototyping and iterative development may be useful from a requirements perspective, he added, but not so much from a quality assurance perspective.
Another good practice is to create feedback loops for measuring how NLG content is doing. "It is going to require a lot of fine tuning," Smartling's Welde said. Metrics to track could include whether the content was reviewed, how much of it was edited and whether some kinds of content are edited more than others. This data can help the enterprise make informed decisions on how to manage NLG tools at scale and help businesses train the AI models.