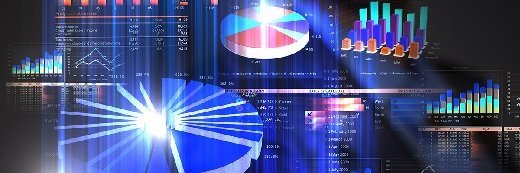
Sergey Nivens - Fotolia
How using predictive analytics helps public agencies predict demand
Predictive analytics aren't just for the private sector. Government agencies are tapping into analytics to gain more lead time in determining demand for services.
Government exists, in part, to dispense services to the public. This distribution of public services is a perfect environment for analytics, which can suggest efficiencies that administrators and service-consuming citizens appreciate.
But the consumption of government services also shines light on possibilities for organizations using predictive analytics.
When citizens apply for public benefits and services, they may contact several government agencies at once -- and in predictable patterns. For example, a young, single mother with three children and little income will apply for food stamps, health coverage for her and her children, and possibly subsidized child care.
In municipal community services, clusters of people in similar circumstances will select a common set of services. If person A consumes service B, he is likely to also consume services D and G, and so on. These service mappings help community service administrators anticipate fluctuations in demand for government benefits. There is predictive power in identifying the clustering of services by customer type.
So, why does this matter? After all, there is no meaningful difference between making predictions of service consumption based on the trending of consumption individually by service, or by services consumed in groups. The fluctuations in consumption are the same, regardless of how you measure them, right?
While individual service consumption won't change, organizations using predictive analytics can achieve greater lead time on the predictions. If I understand ahead of time which customer circumstances are behind the fluctuations in service consumption, I can turn my attention to the patterns of fluctuation in customer circumstances -- and that means, even though my predictive numbers are the same, I can arrive at them faster than previously. A single fluctuation means I can know much sooner when an array of services will also fluctuate. That lead time can have a huge impact on planning and logistics.
Beyond government
This idea that clustering services into groups and mapping them to categories of customers can increase lead times isn't difficult to implement. A great method -- principal component analysis -- works on both ends of this, for analyzing both the services and the customers. The idea is to map which services are related for which sets of constituencies, and then to map the circumstances to increased demand.
The same ideas and methods can be used in many organizations and in many applications:
Product and service distribution. The biggest application of the service-mapping concept is prediction of changes in product and service demand. If, for instance, people buying new homes in the suburbs are also buying home technology products and services in certain combinations, sellers of those products and services should measure the drivers of suburban home sales.
Online resources. Internet service providers, cable and satellite providers, and other service platforms available to the public typically bundle services into packaged offerings. This creates an opportunity to apply analytics to the resulting customer clusters, as well as use social monitoring to measure trends affecting customer preference and choice within those groups.
IT resource deployment. IT can benefit from this style of analysis. If a cloud vendor is allocating storage and site hosting for ground-to-cloud site migration and detects differences in allocation between customers, the vendor needs to start tracking the drivers of mobility among the customers and their users.
Mapping customers and services or products as groups is one of many examples of how using predictive analytics can inspire new ways of thinking about efficiency. And it's a reminder that efficiency isn't always about effort, or even cost, but about time.