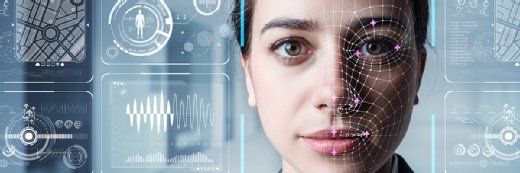
metamorworks - stock.adobe.com
How to overcome 4 major challenges in AI adoption
While companies are stuck in the research phase of AI, a few simple infrastructure analyzations can jumpstart the process -- and ensure successful deployment.
There's not a clear, linear guide on how to deploy AI in the enterprise yet -- due to the newness of the technology and a corresponding lack of industry standards. Even with a clear how-to, successful transition of AI from proof-of-concept to production lags at around 50 percent, and barely 20 percent of AI-enabled companies have managed to deploy it in more than one part of the company, according to a McKinsey consulting firm survey.
Though implementing AI is a far different process than typical IT production applications, considering the challenges in AI adoption and solving them allows for a seamless integration. In order to get beyond the R&D phase with your AI strategy, enterprises need to address potential roadblocks and challenges for AI in existing infrastructure before deployment, not after.
1. Clear AI strategy among processes
It's not always about the technology. Before you jump the hurdles of infrastructure and data, often an AI application won't make it to deployment because the C-suite remains unconvinced that it has proven itself, which is considered a project management failure.
How does such a failure happen? Often, it's a disconnect between the team doing the initial proof-of-concept model and the business owner(s) of the eventual app or process. Executive overseers green light the relatively inexpensive exploratory work, only to pull the plug when costs pile up around shifting requirements, especially unanticipated infrastructure. There can also be disillusionment among the eventual end users when time-to-deployment creeps repeatedly, due to poor change management. Put simply, AI is not only a tough adoption technologically, it's a difficult mindset to adopt when it's new to IT and the business alike.
What's the cure here? Engage successful AI adopters from without. Bring in colleagues from partner companies who have been through their own machine learning tribulations and learn from their experience -- this happens frequently in the healthcare industry, where conference presentations about AI implementations are eagerly anticipated.
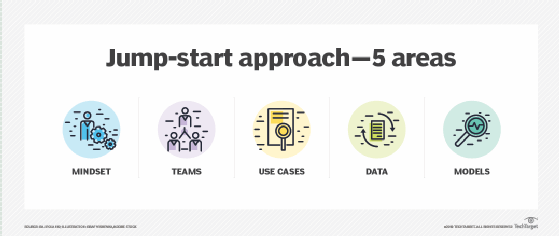
2. Make machine learning models production-friendly
The challenges in AI adoption are largely to do with the fact that its moving parts don't work the same way conventional systems do. Machine learning is the core of most contemporary AI, and it relies upon models that differ from simple process-based tasks, and code within existing legacy systems.
Those legacy systems typically can't be easily integrated with machine learning systems. Their workflow, data management and change control are often out of sync with the needs of a machine learning platform. The data science team developing AI usually can't plan for deployment against legacy systems, and IT personnel tasked with deployment often don't realize that integration of machine learning models with legacy systems isn't going to be practical. It's useful then, to realize that new applications and workflows are needed to optimize the results produced by AI -- as AI won't service existing endpoints, but will create new ones.
Then there are production considerations that often surface too late in a deployment, adding time and expense. The production evaluations include availability -- will the model need to be redundant in production? Is failover a consideration? What will the infrastructure to support this cost? If the AI component exists on more than one machine, how will versioning be handled, to keep things in sync? All of this must be evaluated upfront for a successful deployment.
3. Focus on data quality, quantity and labeling
In order to graduate from R&D into deployed AI, enterprises need to analyze and reconfigure existing data management. Machine learning systems require large amounts of data to function meaningfully, and it's not uncommon that the data needs to be real-time, streaming data. Typically, most enterprise application systems aren't set up this way -- and companies need to reconfigure their data infrastructure.
In a fraud detection AI system, for example, the goal might be to block a fraudulent transaction in the moment at point-of-sale. While the goal is admirable, making it work requires many real-time transactions from many different points to be scored immediately -- and such a flow of data has to be accommodated up front, not as an afterthought. Using AI in such ways requires far more than a good model -- it requires a highly flexible technology stack.
Production considerations have to feed into the design of a conventional app or process before the integration of an AI project, and this starts with asking questions. What's the acceptable latency on the model's output? If it's a real-time system -- visual recognition, or the aforementioned fraud detector -- minimal latency is essential. If it's predictive scoring on marketing campaign content, on the other hand, turnaround doesn't have to be immediate.
4. Ensure scalability
Then there's scaling. Let's say the model deployment is a success. In a conventional deployment, this would mean that scalability has been established. Can the app expand and handle greater throughput? How much? What's been planned for? Too often, getting an AI model deployed at all is considered a holy grail, and having it scale well hasn't been planned for. Requirements for the production performance of the model need to be established in tandem with its development, or the deployment won't be robust, if the model gets deployed at all.
Think big from the start. During R&D, plan to commit to the fact that implementing AI could mean a new technology stack, new apps, new workflows and new processes. Count the cost upfront and take a hard look at the ROI. Don't do this brainstorming in isolation: data science teams, IT, business owners and potential users should plan a new AI venture together with a C-suite member on board to get from research to deployment.