Responsible AI champions human-centric machine learning
Encompassing ethics, transparency and human centricity, responsible AI is an effective approach to deploying machine learning models and achieving actionable insights.
Due to the rapid growth and adoption of AI over the past several years, there has been no lack of articles forecasting dystopian futures in which all jobs and professions have been replaced by robots. That's inherently misleading.
By natural evolution, we have seen the texture of professions with access to big data change. The days of punch card data entry analysts, for example, have given way to the explosion in data ecosystem roles. What's often left out of the AI discussion, however, is augmentation versus automation and keeping humans in the loop.
Responsible AI research is an emerging field that advocates for better practices and methodologies in deploying machine learning models. The goal is to build trust while simultaneously minimizing potential risks not only to the companies deploying these models, but also the customers they serve.
Responsible AI can play a key role in personalizing customer engagements, determining the credit risk of individuals, detecting fraudulent transactions, predicting the likelihood of hospital readmissions and optimizing critical supply chains. Involving humans throughout the AI and machine learning process -- planning, deployment and outcomes -- is paramount.
A major framework of considerations for responsibility in machine learning is often FAT or FATE, which typically stands for fairness, accountability, transparency and ethics. For obvious reasons, having a human in the loop dramatically increases human accountability for AI and machine learning systems, which we have seen a general lack of in industry. Additionally, when considering fairness and ethics issues, having human domain expertise to interpret complex model inferences will become increasingly critical -- for example, a well-defined human-centric machine learning system wherein a data scientist can confirm that protected classes aren't being disparately treated or that protected features aren't being reverse engineered by the models.
Human-centric machine learning is one of the more important concepts in the industry to date. Leading institutions such as Stanford and MIT are setting up labs specifically to further this science. MIT defines this area as "the design, development and deployment of [information] systems that learn from and collaborate with humans in a deep, meaningful way."
The future of work is often portrayed as being dominated by a robotic apparatus and thousands of algorithms pretending to be humans. But the reality is that AI adoption has been largely aimed at "making processes more efficient," "enhancing existing products and services" and "creating new products and services," according to Deloitte's recent survey of corporate executives, who rated "reducing headcount" as their least important goal.
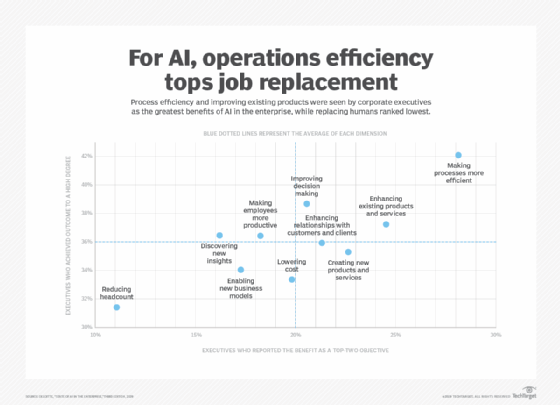
Implementing AI as a job killer has, in many cases, proved to be uneconomical and not a viable option. Many companies that adopt AI are quite mature and often demand a technically skilled and talented workforce. Recruiting, hiring, training and compensating these employees require significant investments. Removing these valuable resources based on a machine learning model that might show some predictiveness is almost certainly the wrong approach.
Ironically, the most common and highest value examples of responsible AI and human-centric machine learning are the least talked about simply because they're not as exciting as, say, a Boston Dynamics military robot. Nonetheless, applications are wide-ranging across industries:
- IoT. Increased intelligence on lifestyle habits through the use of wearables like smartwatches.
- Call center. Real-time AI-driven insights on sales and customer support to monitor and improve agent performance.
- Predictive maintenance. Industrial and manufacturing managers can receive early alerts to prevent costly repairs and downtime of heavy machinery.
- Consultants and analysts. Deeper analytical methods that drive better decisions.
The list of applications is virtually endless. But many of these cases ultimately trace back to our ability to run more sophisticated machine learning models. One side of the value coin is that these models can take a tremendous amount of structured and unstructured data and distill it down to highly accurate recommendations and predictions. The other side of the coin is their ability to automate the rote and redundant workflows so people can devote more time to complex, high-value and high-risk profit-driven tasks as well as make better decisions.
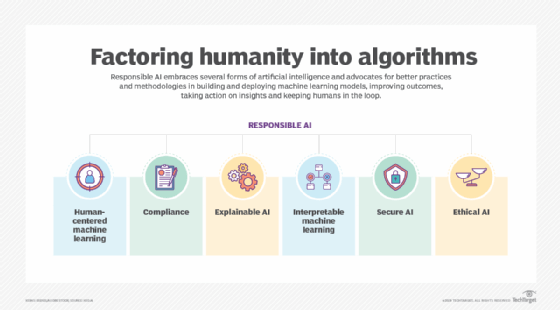
AI in radiology, for example, can quickly draw attention to obvious findings as well as highlight the much more subtle areas that might not be readily caught by the human eye. Responsible AI human-centricity comes into play when doctors and patients, not machines, make the final decision on treatment. Still, augmenting medical professionals with deep quantitative insight equips them with invaluable information to factor into the decision.
By keeping humans in the loop, companies can better determine the level of automation and augmentation they need and control the ultimate effect of AI on their workforce. As a result, enterprises can massively mitigate their risk and develop a deeper understanding of what types of situations may be the most challenging for their AI deployments and machine learning applications.