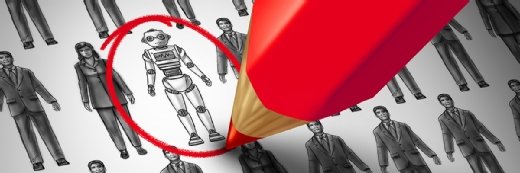
freshidea - stock.adobe.com
The threats of AI must be taken seriously to prevent harm
The risks of AI use are growing as the technology becomes more pervasive. Rather than laugh off the threats, businesses should move to mitigate them before they become headaches.
Technology futurist and entrepreneur Elon Musk has frequently mused on the threats of AI, most recently in a talk at the South by Southwest technology conference in which he called AI "more dangerous than nuclear warheads."
While mainstream technologists and social scientists may dismiss Musk's foreboding proclamations, it's worth thinking about the current state of the integration of machine learning and artificial intelligence into everyday applications and to ask whether we are ready to rely on algorithms.
AI is not a new phenomenon. Research on AI started back in the late 1950s, with various stops and starts throughout the past six decades. In that time, many machine learning approaches and algorithms have been developed. However, until relatively recently, the AI practice has largely taken place behind the scenes. So what has changed to trigger the renewed interest in -- and, in the case of Elon Musk, growing fear of -- artificial intelligence?
In the earlier days of AI and machine learning, the roles of the software developer and the analyst were conflated -- to be able to use the algorithm, one had to know how to program it. The tipping point came following two technology advances.
First, machine learning libraries encapsulated the workings of the analytical models, enabling an analyst to understand what these algorithms were capable of doing without having to understand how they worked.
Second, these machine learning libraries were incorporated into open source software distributions available to the general public. By providing a lower barrier to entry, the emergence of production machine learning and AI tools has allowed businesses to increasingly use clustering, anomaly analysis, segmentation, classification and prediction to enhance their business applications for IoT event analytics, cybersecurity, production quality, predictive maintenance, fraud analysis, recommendation, product pricing, sentiment analysis and more.
Simplifying the use of analytical algorithms has created new opportunities for information specialists who are not computer scientists to effectively design and build applications that exploit AI and machine learning. Yet, despite the reported successes, there are lingering doubts about trusting automated intelligent algorithms that lend credence to Elon Musk's suspicions about the threats of AI.
It is true that the increasing integration of analytics algorithms will generally improve operations, but we are far from the point where we can rely on algorithmic integrity. Consider these examples:
- In light of continued investigations into Russian interference in the 2016 elections, there have been allegations of malicious actors effectively reverse-engineering how the Facebook advertising placement algorithms work, enabling them to game the system to bypass the intent of the algorithm and influence voter behaviors.
- Uber's recent fatal self-driving car accident demonstrates that the algorithms only perform well in situations similar to those that they are trained for, but they cannot accommodate situations in which the inputs are limited or when they encounter unanticipated behaviors.
- Deep learning and other similar algorithms and models do not provide rational explanations of how they work, which can lead to potential abuse, misuse and difficulty explaining recommendations. This makes it hard to recover from a virtual failure.
Not only that, the increasing reliance on big data to fuel analytics is going to start bumping up against shifting consumer expectations for how their data is used. Many people don't realize that when they sign up for what is advertised as a free service, the terms of service they agree to specifically state that they are yielding control over how their personal data is used.
In other words, not only are you granting the company a license to use any content you submit through any of their services -- such as phrases you search for, the content of emails sent via Gmail or the documents you edit in Google Docs -- you are allowing them to scan the content so that they can personalize the presentation of advertisements back to you. In essence, you are paying for the free service with the currency of your private information.
When these concerns over the threats of AI are viewed together, it becomes clear why there are growing calls for increased scrutiny over the ways that intelligent agents and machine learning algorithms consume and take advantage of data.
Organizations that are thriving on analytics should think about developing information policies and governance frameworks to provide auditable methods to comply with regulations and observe data use agreements to protect themselves against what is likely to be a storm of legal and regulatory actions.