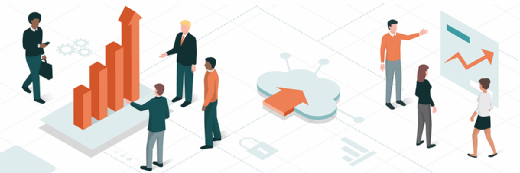
O'Reilly machine learning survey: Enterprises aim for maturity
A new survey finds companies are using fairness and bias as metrics to evaluate the success of a machine learning model.
survey findings by O'Reilly Media Inc. suggest enterprise machine learning is on the road to maturity.
The report's authors, Ben Lorica, chief data scientist at O'Reilly, and Paco Nathan, a data science and machine learning expert, set out to answer basic enterprise adoption questions, such as how companies are using machine learning and how far along companies are toward enterprise-wide use.
"We were surprised to hear about the level of adoption and maturity," Lorica said. "We decided to see if what we're on a rather biased sample resonated with the broader community."
The short O'Reilly machine learning survey of more than 11,400 decision-makers, data scientists, data engineers and analysts found that "sophisticated" companies, or those with machine learning models in production for more than five years, treat machine learning as a distinct discipline that requires unique titles and processes. They're also taking a more comprehensive look at the model performance by including model explainability, transparency, fairness and bias metrics in the process.
Beyond software engineering
The results of the survey, The State of Machine Learning Adoption in the Enterprise, suggest that sophisticated companies don't shoehorn enterprise machine learning into the category of software engineering. "I think the community is starting to realize more and more that this is not quite the same as regular software development," Lorica said.
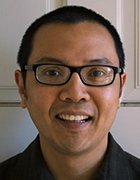
Indeed, more experienced companies appear to be adjusting their culture and are experimenting with processes to meet new challenges presented by enterprise machine learning -- for good reason.
"If you talk to people who do a lot of this, one of the things they'll tell you is that a lot of the work actually happens once you deploy the model to production," Lorica said. "Models degrade, they can misbehave, and so you have to monitor them; you have to know when to retrain them."
The survey found, for example, that more experienced companies have adopted new titles, such as research scientist, a developer of sophisticated algorithms and machine learning engineer -- a bridge between data engineers and data scientists. The titles are less popular for early adopters and companies just beginning to use machine learning, which the survey referred to as explorers.
More experienced companies also rely on data scientists, rather than product managers or executives, to determine the key metrics for project success. Almost three-fourths of respondents representing the most experienced companies use their team of data scientists to build machine learning models, compared to 66% of early adopters and 32% of explorers.
And in response to a question about what methodology they use for machine learning work, 48% of respondents selected Agile. The authors were reluctant to label it as the default methodology, because the results showed 32% of respondents -- mostly explorers -- selected "no methodology." And another 11% -- mostly experienced companies -- selected "other methodology."
"There are companies that are taking that Agile methodology and adjusting it to data science," Lorica said, pointing to a speaker who will be doing just that at the upcoming Strata Data Conference next month.
Uptick in fairness, transparency
For Lorica, the most surprising results in the O'Reilly machine learning survey also provided glimmers of hope. "Most people are starting to take seriously some of these other considerations when you're doing machine learning," he said. "So, besides optimizing your business metric and your machine learning metric, people are looking at other things, like fairness and bias."
In total, 17% of respondents reported using metrics of bias and fairness in measuring the success of their enterprise machine learning program. The percentages are even higher for sophisticated companies, with 26% reporting the use of bias and fairness as metrics, compared to 14% of explorers.
Lorica also said the findings confirmed some of the anecdotal data he was on auto ML, or automated machine learning services, and cloud adoption. Only 3% of respondents reported that they were using cloud machine learning services. For sophisticated companies and early adopters, the percentages were even smaller at 2%, respectively.
"It turns out that if you're going to use deep learning heavily, the economics favor going on-prem," Lorica said. Deep learning models need a lot of data and time to be trained effectively, which makes brining the work in house more attractive.