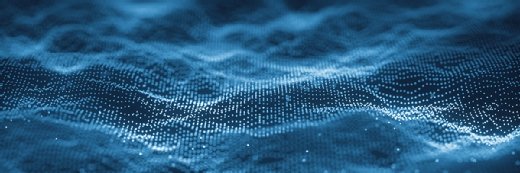
Nabugu - stock.adobe.com
The benefits and challenges of AI in network monitoring
Using AI to track network activity streamlines management and predictive maintenance. But questions about data output integrity, security risks and integration complexities remain.
As enterprises push for more AI-driven trials, IT practitioners want ways to optimize the performance and security of their infrastructures. AI shows promise as one of these tools.
AI-based network monitoring is becoming a prominent method to capture network performance data. It establishes the location of bottlenecks and flags other performance and security anomalies that might disrupt service quality. AI-driven network monitoring can automate remediations, preventing service disruptions.
What is AI network monitoring?
The main objectives of AI network monitoring include the following:
- Sustain optimal service levels.
- Gain accurate insight into potential infrastructure issues.
- Capture data before business and network operations are negatively affected.
To help with this process, machine learning (ML) applies algorithms to telemetry and other data streams to set a normal operations baseline. Once the AI network monitoring service establishes the standard for normal activity, it can look for deviations that might indicate infrastructure problems. Conceptually, AI monitoring expedites troubleshooting and minimizes costly network downtime.
AI monitoring is becoming a key element in AIOps, the marriage of AI and IT ops. Here, ML is applied to big data to troubleshoot problems and automate formerly manual processes.
AIOps encompasses a spectrum of network management, including event correlation, anomaly detection and root cause analysis. By providing insights into IT ops, AIOps enables IT teams to make more informed decisions about resource allocation and address potential issues.
AIOps offers many benefits, including the following:
- Optimal resource usage.
- Expedited troubleshooting.
- Cost reduction.
Tapping into AI monitoring -- and, more broadly, AIOps -- to support network operations can be particularly important as organizations deploy more connected devices across their environments. Tracking both traditional IT infrastructure and industrial devices in a consolidated fashion should increase operational efficiencies and potentially reduce costs.
Benefits of AI network monitoring
AI is a key element in non-IT management use cases, such as autonomous driving and natural language processing for smart speakers. Vendors are increasingly integrating AI capabilities into IT monitoring and management solutions.
AI network monitoring offers several potential advantages that create a more finely tuned, reliable, nimble and high-performing enterprise environment. The benefits of AI network monitoring include the following:
- Proactive IT monitoring.
- Security insights.
- Driving automation.
Proactive IT monitoring
Networks continue to evolve in terms of the density of connected components and gear and in their virtualized environments. Monitoring these environments became an involved and often inefficient process. Reactive management results in diminished service quality and outages. IT often uses disparate monitoring tools to isolate sources of service-level degradation.
AI network monitoring, however, promises to eliminate the fog associated with tracking network activity by providing a clear view of all infrastructure elements as they interoperate, which streamlines IT ops. AI monitoring also expedites and improves root cause analysis to find the source of a problem quickly. AI-driven network monitoring also predicts potential problems before service is interrupted.
Security insights
AI monitoring sheds light on potential security issues before they affect enterprise resources. It rapidly and accurately identifies threats and drives faster remediation. This reduces false positive alerts that flood security analysts and helps organizations improve their overall security postures.
Driving automation
AI can inform systems that it can automate previously manual processes. While some of these processes are iterative, ongoing maintenance support elements, some organizations are exploring automation for higher-level tasks. For example, AI can trigger automated mitigation and remediation responses.
Challenges of AI network monitoring
Despite the advantages of AI network monitoring in a production environment, it has challenges that can impede successful implementation. IT ops teams must overcome learning curves to use AI monitoring effectively.
At the outset of deployment, network operations teams should consider the following challenges:
- Data quality.
- Integration.
- Ethical issues.
- False positives.
Data quality
When AI differentiates harmless anomalies from actual network problems, it's only as effective as the data it sources. Missing real-time data or data integrity issues can lead to inaccurate results. As an alternative to real-time data feeds, many organizations use synthetic data created to simulate activity on a production network. Although synthetic data is similar to real network activity, it's not identical and could miss an issue or flag a nonexistent problem.
Integration
Integration can be tricky when deploying any new technology into an existing production environment. If the IT organization cannot sync its AI network monitoring tools with other management and security services, the output might be subpar.
Ethical issues
As AI tools continue to advance, ethical issues emerge surrounding misuse and operational integrity. This is no different in network monitoring, where AI technology analyzes vast amounts of data. Governments are working to regulate how AI uses data, but it's a difficult and evolving task.
False positives
AI development has had significant progress over the last few years, but issues remain. Training systems in areas such as security can produce false positives or miss serious threats. Trust not only takes time, but also accurate output.
Amy Larsen DeCarlo has covered the IT industry for more than 30 years, as a journalist, editor and analyst. As a principal analyst at GlobalData, she covers managed security and cloud services.