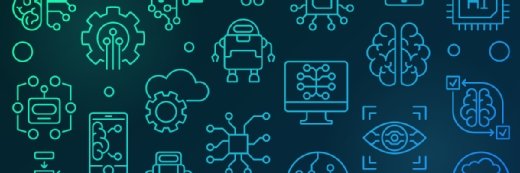
Battle of the buzzwords: AIOps vs. MLOps square up
Another -Ops has entered the arena: MLOps. Is it just another buzzword, or does the term hold its own weight? Learn more about it and how it compares to AIOps.
AIOps and MLOps are terms that might appear to have a similar meaning, given that the acronyms on which they are based -- AI and ML -- are often used in similar contexts. However, AIOps and MLOps mean radically different things.
A team or company might use both AIOps and MLOps at the same time but not for the same purposes. Let's dig into what each is individually and then whether they can be used together.
What is AIOps?
AIOps, which stands for artificial intelligence for IT operations, is the use of AI to help perform IT operations work.
For example, a team that uses AIOps might use AI to analyze the alerts generated by its monitoring tools and then prioritize the alerts so that the team knows which ones to focus on. Or an AIOps tool could automatically find and fix an application that has crashed, using AI to determine the cause of the problem and the proper remediation.
What is MLOps?
Short for machine learning IT operations, MLOps is a technique that helps organizations optimize their use of machine learning and AI tools.
The core idea behind MLOps is that the stakeholders involved in making decisions about machine learning and AI are typically siloed from each other. Data scientists know how AI and machine learning algorithms work. But they don't usually collaborate closely with IT engineers, responsible for deploying AI and machine learning tools, or with compliance officers, who manage security and regulatory aspects of machine learning and AI use.
Put another way, MLOps is like DevOps in that it seeks to break down the silos that separate different types of teams. But, whereas DevOps is all about encouraging collaboration between developers and IT operations teams, MLOps focuses on collaboration between everyone who plays a role in choosing or managing machine learning and AI resources.
Too many Ops?
It's tempting to assume that AIOps and MLOps basically mean the same thing, given that AI and machine learning mean similar -- albeit not identical -- things.
But, in fact, the terms are not closely related at all. You could argue that a healthy MLOps practice would help organizations choose and deploy AIOps tools, but that's only one possible goal of MLOps. Beyond that, AIOps and MLOps don't intersect.
This is a sign that the tech community has overused the -Ops construction. When you can take any noun, add the -Ops suffix and invent a new buzzword -- without logical consistency to unite it with similarly formed buzzwords -- it might be time to move onto new techniques for labeling buzzwords.