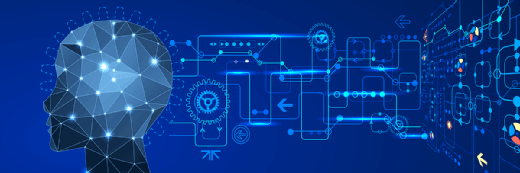
Artificial general intelligence examples remain out of reach
Artificial general intelligence remains largely an aspiration goal of researchers, but as technologies advance, so too does the dream become more realistic.
The reality of artificial intelligence is far from that of science fiction. The edge of AI research and capabilities has not yet reached a level of sophistication that would put them in the category of artificial general intelligence.
Currently, AI technologies enable the ability to recognize objects and features in images, have somewhat passing conversations with people, provide greater ability for pattern and anomaly detection, and provide deeper predictive analytics. These applications of artificial intelligence are not of the general intelligence that is breathlessly anticipated in science fiction.
Despite what Elon Musk, Stephen Hawking and many others have insisted, AI researchers are still a breakthrough or two -- or more -- away from cracking the code on making machines truly intelligent.
Narrow AI vs. AGI
One of the challenges of the term artificial intelligence is its vague nature as a term. A conversation about AI has to differentiate which technology under its umbrella is being discussed, as well as what level of intelligence is the aim. On top of this, those working with AI must define what the concept of intelligence is.
To address these various issues, researchers in the industry crafted terminology to make these concepts more concrete. When talking about developing technologies that implement specific cognitive abilities -- say, being able to recognize something in an image or understand parts of speech -- we call these application-specific cognitive abilities narrow AI. At the other end of the spectrum, research efforts that aim to achieve the full spectrum of all the cognitive capabilities that humans are capable of are known as artificial general intelligence (AGI) initiatives.
A narrow AI system is only capable of the specific intelligence tasks that the system has been trained to do, whereas an AGI system is capable of the full range of cognitive capability, handling application of intelligence to new domains, adapting to new circumstances and deriving new information even in environments where there's sparse or vague information. Essentially, AGI must match the capabilities of humans when it comes to cognition and proof of intelligence.
Machine learning and neural networks lead the way
One of the problems with achieving AGI is the impressive complexity of the human brain. We might know how it works from a biological perspective, but the actual method by which our brain accomplishes many of the feats of cognition remain a mystery and, therefore, nearly impossible to replicate. This mystery prevents technologists from implementing cognitive capabilities with any ease. Instead, researchers have a variety of theories and approaches that mimic aspects of cognitive ability.
The current peak of intelligent machines is advanced forms of machine learning that attempt to identify and derive patterns from data. These deep learning neural networks have had the best overall performance at narrow intelligence tasks.
Artificial neural networks are a software approach that aims to mirror the brain's structure by connecting a large set of simple artificial neurons in a network of complex interactions that can accomplish many learning tasks. The most sophisticated of these are deep learning neural nets, which use many hidden learning layers between the input and output neurons. These neural nets have a variety of forms, or architectures, from convolutional neural nets to recurrent neural nets, like long short-term memory networks, to transformer architectures, such as Bidirectional Encoder Representations from Transformers and Generative Pre-trained Transformer 3, which are garnering a lot of attention lately.
Furthermore, researchers are also diving into reinforcement learning approaches that aim to find optimal solutions to problems through iterative, trial-and-error approaches. These efforts have seen significant success, especially when applied to solving puzzles and playing games.
DeepMind's AlphaZero and efforts by the Google-owned AI lab have shown just how far we can go with these advanced forms of learning. And yet, despite all the research, effort and money being pumped into these research efforts, AGI still remains beyond our grasp.
Where is AGI now?
There are researchers looking into making AGI a reality with lots of funding and resources being put toward this goal. Efforts to achieve AGI vary widely from efforts as diverse as large deep learning neural nets that can accomplish increasingly more complicated tasks to biologically inspired efforts that aim to find the core of what makes intelligence work. AI researcher Carlos Perez has mapped out the various approaches to AGI.
From his perspective, formulaic approaches to AGI aim to find a small set of patterns that can be collectively used for greater intelligence, whereas ecological approaches aim to find the large set of patterns that can explain the range of intelligence capabilities. Functionalist approaches focus on research that can find a small set of capabilities that can explain a wide set of intelligent phenomena, while an enactivist approach aims to create lots of capabilities that can be combined in many complex ways.
From this perspective of a classification of AGI efforts, DeepMind, which aims to create intelligent systems by "self-play" -- iterating against itself many times to find a solution without trying to identify the single truth -- is an ecological, enactivist approach to AGI. On the other hand, OpenMind's efforts to create large models that can be used for a wide range of tasks are more functional, enactivist approaches.
Other researchers aim to find the truth in symbolic approaches to AI or other approaches that use genetic algorithms or evolutionary techniques that aim to replicate the years of evolution that have resulted in the human brain. These approaches are being pursued by many different researchers at academic and commercial institutions. Despite all these efforts, no one has cracked the code yet, and as such, any of these approaches could be potentially feasible.
The future of AGI
AI, when compared to the fields of physics, biology and chemistry, is still a relatively young field of study. AGI is in its infancy as researchers are just at the beginnings of understanding the complexities of what makes intelligence work. As such, there's no doubt that many decades ahead of research await those pursuing AGI.
What is most frustrating about the pursuit of AGI is that, at times, the capabilities of narrow AI systems seemingly replicate the capacity of a real AGI system. However, a closer look at those narrow AI approaches reveals that they remain far away from that lofty goal of AGI. Simple intelligence tasks, such as having a normal conversation, as well as basic common sense, remain out of reach for these systems.
Deep learning was a revolution in AI that, in combination with big data and loads of computing power, has made many narrow AI tasks much more possible than previous approaches. And yet, deep learning research is decades old. There are many in the industry that feel we are in dire need of a new breakthrough in the field to achieve the next level of AI capability.
The enthusiasm for the field hasn't wavered, and some even feel that we are just a few years away from that breakthrough. This certainly explains the billions of dollars in AI research that firms like Microsoft and Google have committed to thus far. However, there are others that feel that AGI is much further away from reality. The truth is that we just don't know. The future possibility of AGI, like the future possibility of colonizing other planets, is at once just ahead of us and possibly forever outside our grasp.