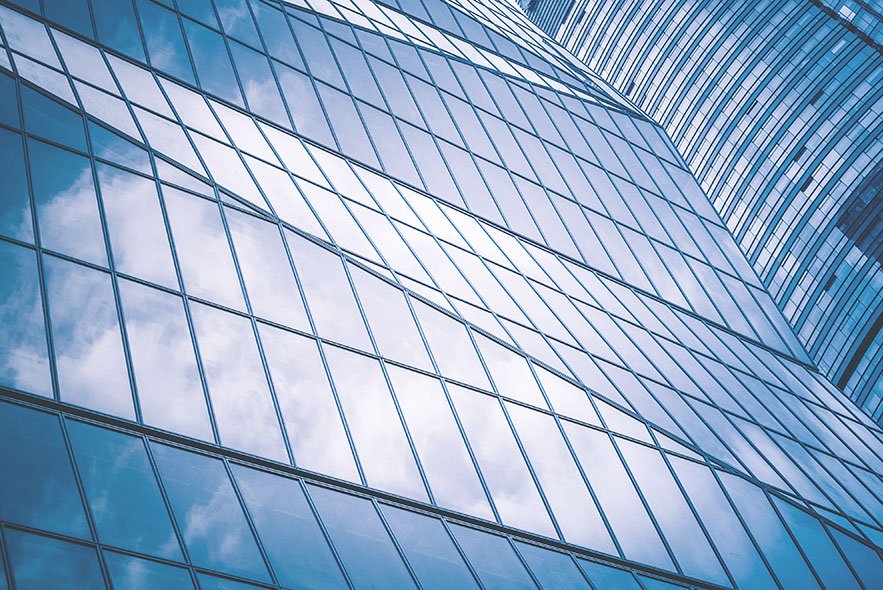
neuromorphic computing
What is neuromorphic computing?
Neuromorphic computing is a method of computer engineering in which elements of a computer are modeled after systems in the human brain and nervous system. The term refers to the design of both hardware and software computing elements. Neuromorphic computing is sometimes referred to as neuromorphic engineering.
Neuromorphic engineers draw from several disciplines -- including computer science, biology, mathematics, electronic engineering and physics -- to create bio-inspired computer systems and hardware. Of the brain's biological structures, neuromorphic architectures are most often modelled after neurons and synapses. This is because neuroscientists consider neurons the fundamental units of the brain.
Neurons use chemical and electronic impulses to send information between different regions of the brain and the rest of the nervous system. Neurons use synapses to connect to one another. Neurons and synapses are far more versatile, adaptable and energy-efficient information processors than traditional computer systems.
Neuromorphic computing is an emerging field of science with no real-world applications yet. Various groups have research underway, including universities; the U.S. military; and technology companies, such as Intel Labs and IBM.
This article is part of
A guide to artificial intelligence in the enterprise
Neuromorphic technology is expected to be used in the following ways:
- deep learning applications;
- next-generation semiconductors;
- transistors;
- accelerators; and
- autonomous systems, such as robotics, drones, self-driving cars and artificial intelligence (AI).
Some experts predict that neuromorphic processors could provide a way around the limits of Moore's Law.
The effort to produce artificial general intelligence (AGI) also is driving neuromorphic research. AGI refers to an AI computer that understands and learns like a human. By replicating the human brain and nervous system, AGI could produce an artificial brain with the same powers of cognition as a biological one. Such a brain could provide insights into cognition and answer questions about consciousness.
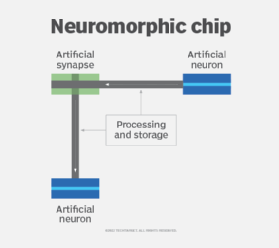
How does neuromorphic computing work?
Neuromorphic computing uses hardware based on the structures, processes and capacities of neurons and synapses in biological brains. The most common form of neuromorphic hardware is the spiking neural network (SNN). In this hardware, nodes -- or spiking neurons -- process and hold data like biological neurons.
Artificial synaptic devices connect spiking neurons. These devices use analog circuitry to transfer electrical signals that mimic brain signals. Instead of encoding data through a binary system like most standard computers, spiking neurons measure and encode the discrete analog signal changes themselves.
The high-performance computing architecture and functionality used in neuromorphic computers is different from the standard computer hardware of most modern computers, which are also known as von Neumann computers.
Von Neumann computers
Von Neumann computers have the following characteristics:
- Separate processing and memory units. Von Neumann computers have separate central processing units for processing data and memory units for storing data.
- Binary values. Von Neumann computers encode data using binary values.
- Speed and energy issues. To compute, data must be moved between the separate processing and memory locations. This approach is known as the von Neumann bottleneck; it limits a computer's speed and increases its energy consumption. Neural network and machine learning software run on von Neumann hardware typically must provide either fast computation or low energy consumption, achieving one at the expense of the other.
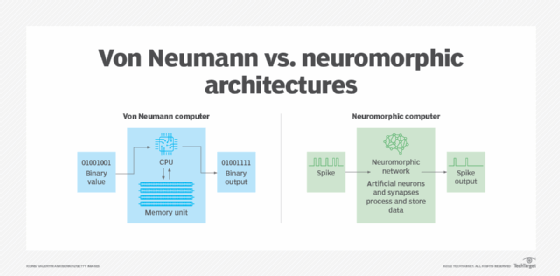
Neuromorphic computers
Neuromorphic computers have the following characteristics:
- Collocated processing and memory. The brain-inspired neuromorphic computer chips process and store data together on each individual neuron instead of having separate areas for each. By collocating processing and memory, neural net processors and other neuromorphic processors avoid the von Neumann bottleneck and can have both high performance and low energy consumption at the same time.
- Massively parallel. Neuromorphic chips, such as Intel Lab's Loihi 2, can have up to one million neurons. Each neuron operates on different functions simultaneously. In theory, this lets neuromorphic computers perform as many functions at one time as there are neurons. This type of parallel functioning mimics stochastic noise, which is the seemingly random firings of neurons in the brain. Neuromorphic computers are designed to process this stochastic noise better than traditional computers.
- Inherently scalable. Neuromorphic computers do not have traditional roadblocks to scalability. To run larger networks, users add more neuromorphic chips, which increases the number of active neurons.
- Event-driven computation. Individual neurons and synapses compute in response to spikes from other neurons. This means only the small portion of neurons actually processing spikes are using energy; the rest of the computer remains idle. This makes for extremely efficient use of power.
- High in adaptability and plasticity. Like humans, neuromorphic computers are designed to be flexible to changing stimuli from the outside world. In the spiking neural network -- or SNN -- architecture, each synapse is assigned a voltage output and adjusts this output based on its task. SNNs are designed evolve different connections in response to potential synaptic delays and a neuron's voltage threshold. With increased plasticity, researchers hope neuromorphic computers will learn, solve novel problems and adapt to new environments quickly.
- Fault tolerance. Neuromorphic computers are highly fault tolerant. Like the human brain, information is held in multiple places, meaning the failure of one component does not prevent the computer from functioning.
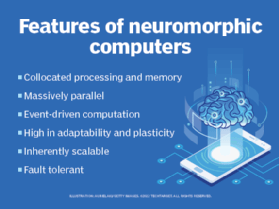
Challenges of neuromorphic computing
Many experts believe neuromorphic computing has the potential to revolutionize the algorithmic power, efficiency and capabilities of AI as well as reveal insights into cognition. However, neuromorphic computing is still in early stages of development, and it faces several challenges:
- Accuracy. Neuromorphic computers are more energy efficient than deep learning and machine learning neural hardware and edge graphics processing units (GPUs). However, they have still not proven themselves to be conclusively more accurate than them. Combined with the high costs and complexity of the technology, the accuracy issue leads many to prefer traditional software.
- Limited software and algorithms. Neuromorphic computing software has not caught up with the hardware. Most neuromorphic research is still conducted with standard deep learning software and algorithms developed for von Neumann hardware. This limits research results to standard approaches, which neuromorphic computing is trying to evolve beyond. Katie Schuman, a neuromorphic computing researcher and an assistant professor at the University of Tennessee, said in an interview with Ubiquity that adoption of neuromorphic computing technologies "will require a paradigm shift in how we think about computing as a whole …. Though this is a difficult task, continued innovation in computing depends on our willingness to move beyond our traditional von Neumann systems."
- Inaccessible. Neuromorphic computers aren't available to nonexperts. Software developers have not yet created application programming interfaces, programming models and languages to make neuromorphic computers more widely available.
- Benchmarks. Neuromorphic research lacks clearly defined benchmarks for performance and common challenge problems. Without these standards, it's difficult to assess the performance of neuromorphic computers and prove efficacy.
- Neuroscience. Neuromorphic computers are limited to the known structures of human cognition, which is still far from completely understood. For instance, there are several theories that propose human cognition is based on quantum computation, such as the Orch (OR) theory proposed by Sir Roger Penrose and Stuart Hameroff. If cognition requires quantum computation as opposed to standard computation, neuromorphic computers would be incomplete approximations of the human brain and might need to incorporate technologies from fields like probabilistic and quantum computing.
What are the use cases for neuromorphic computing?
Despite challenges, neuromorphic computing is still a highly funded field. Experts predict neuromorphic computers will be used to run AI algorithms at the edge instead of in the cloud because of their smaller size and low power consumption. Much like a human, AI infrastructure running on neuromorphic hardware would be capable of adapting to its environment, remembering what's necessary and accessing external sources, like the cloud, for more information when necessary.
Other potential applications of this technology include the following:
- driverless cars;
- drones;
- robots;
- smart home devices;
- natural language, speech and image processing;
- data analytics; and
- process optimization.
Neuromorphic computing research tends to take either a computational approach, focusing on improved efficiency and processing, or a neuroscience approach, as a means of learning about the human brain. Both approaches generate knowledge that is required to advance AI.
Examples of the computational approach
- Intel Lab's Loihi 2 has two million synapses and over one million neurons per chip. They are optimized for SNNs.
- Intel Lab's Pohoiki Beach computer features 8.3 million neurons. It delivers 1,000 times better performance and 10,000 times more efficiency than comparable GPUs.
- IBM's TrueNorth chip has over 1 million neurons and over 256 million synapses. It is 10,000 times more energy-efficient than conventional microprocessors and only uses power when necessary.
- NeuRRAM is a neuromorphic chip designed to let AI systems run disconnected from the cloud.
Examples of the neuroscience approach
- The Tianjic chip, developed by Chinese scientists, is used to power a self-driving bike capable of following a person, navigating obstacles and responding to voice commands. It has 40,000 neurons, 10 million synapses and performs 160 times better and 120,000 times more efficiently than a comparable GPU.
- Human Brain Project (HBP) is a research project created by neuroscientist Henry Markram with funding from the European Union that is attempting to create a human brain. It uses two neuromorphic supercomputers, SpiNNaker and BrainScaleS, collaboratively designed by various universities. More than 140 universities across Europe are working on the project.
- BrainScaleS was created by Heidelberg University in collaboration with other universities. It uses neuromorphic hybrid systems that combine biological experimentation with computational analysis to study brain information processing.
Neuromorphic computing and artificial general intelligence
AGI refers to AI that exhibits intelligence equal to that of humans. Reaching AGI is the goal of AI research. Though machines have not, and may never, reach a human level of intelligence, neuromorphic computing offers the potential for progress toward that goal.
For example, the Human Brain Project is an AGI project that uses the SpiNNaker and BrainScaleS neuromorphic supercomputers to perform sufficient neurobiological functions attempting to produce consciousness.
Some of the criteria for determining whether a machine has achieved AGI is whether the machine has the following capabilities:
- reason and make judgments under uncertainty;
- plan;
- learn;
- showcase natural language understanding;
- display knowledge, including common-sense knowledge; and
- integrate these skills in the pursuit of a common goal.
Sometimes the capacity for imagination, subjective experience and self-awareness are included. Other proposed methods of confirming AGI include the Turing Test, which states that a machine is sentient if an observer cannot tell it apart from a human. The Robot College Student Test is another test, in which a machine enrolls in classes and obtains a degree like a human student.
There are debates about the ethics and legal issues surrounding the handling of a sentient machine. Some argue that sentient machines should be treated as a nonhuman animal in the eyes of the law. Others argue a sentient machine should be treated as a person and protected by the same laws as human beings. Many AI developers follow an AI code of ethics that provides guiding principles for the development of AI.
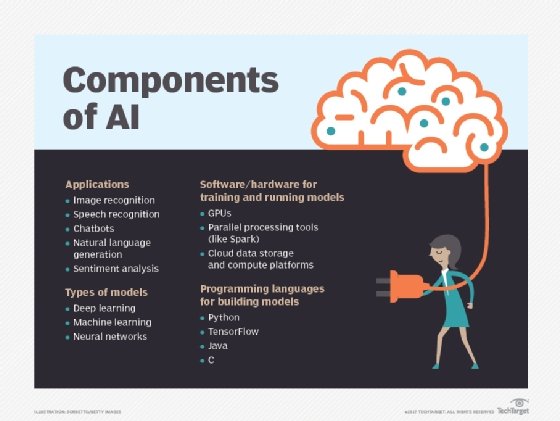
History of neuromorphic computing
Scientists have been attempting to create machines capable of human cognition for decades. Work in this area has been closely tied to advances in mathematics and neuroscience. Early breakthroughs include the following:
1936. Mathematician and computer scientist Alan Turing created a mathematical proof that a computer could perform any mathematical computation if it was provided in the form of an algorithm.
1948. Turing wrote "Intelligent Machinery," a paper that described a cognitive modeling machine based on human neurons.
1949. Canadian psychologist Donald Hebb made a breakthrough in neuroscience by theorizing a correlation between synaptic plasticity and learning. Hebb is commonly called the "father of neuropsychology."
1950. Turing developed the Turing Test, which is still considered the standard test for AGI.
1958. Building on these theories, the U.S. Navy created the perceptron with the intention of using it for image recognition. However, the technology's imitation of biological neural networks was based on limited knowledge of the brain's workings, and it failed to deliver the intended functionality. Nevertheless, the perceptron is considered the predecessor to neuromorphic computing.
1980s. Neuromorphic computing as it's known today was first proposed by Caltech professor Carver Mead. Mead created the first analog silicon retina and cochlea in 1981, which foreshadowed a new type of physical computation inspired by the neural paradigm. Mead proposed that computers could do everything the human nervous system does if there was a complete understanding of how the nervous system worked.
2013. Henry Markram launched the HBP with the goal of creating an artificial human brain. HBP incorporated state of the art neuromorphic computers and has a 10-year horizon in which to better understand the human brain and apply this knowledge to medicine and technology. Over 500 scientists and 140 universities across Europe are working on the project.
2014. IBM developed the TrueNorth neuromorphic chip. The chip is used in visual object recognition and has lower power consumption than traditional von Neumann hardware.
2018. Intel developed the Loihi neuromorphic chip that has had applications in robotics as well as gesture and smell recognition.
The future of neuromorphic computing
Recent progress in neuromorphic research is attributed in part to the widespread and increasing use of AI, machine learning, neural networks and deep neural network architectures in consumer and enterprise technology. It can also be attributed to the perceived end of Moore's law among many IT experts.
Moore's Law states that the number of transistors that can be placed on a microchip will double every two years, with the cost staying the same. However, experts forecast that the end of Moore's Law is imminent. Given that, neuromorphic computing's promise to circumvent traditional architectures and achieve new levels of efficiency has drawn attention from chip manufacturers.
Recent developments in neuromorphic computing systems have focused on new hardware, such as microcombs. Microcombs are neuromorphic devices that generate or measure extremely precise frequencies of color. According to a neuromorphic research effort at Swinburne University of Technology, neuromorphic processors using microcombs can achieve 10 trillion operations per second. Neuromorphic processors using microcombs could detect light from distant planets and potentially diagnose diseases at early stages by analyzing the contents of exhaled breath.
Because of neuromorphic computing's promise to improve efficiency, it has gained attention from major chip manufacturers, such as IBM and Intel, as well as the United States military. Developments in neuromorphic technology could improve the learning capabilities of state-of-the-art autonomous devices, such as driverless cars and drones.
Neuromorphic computing is critical to the future of AI. Learn the top nine applications of AI in business.