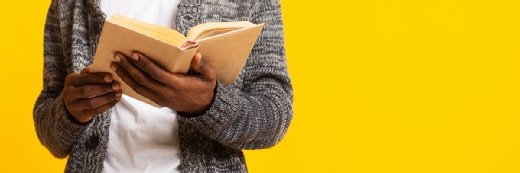
Getty Images/iStockphoto
6 top AI books to read in 2021
Many sources provide comprehensive information on the fundamentals and applications of artificial intelligence, so we narrowed the field to six of the more diverse AI books.
Whether you're a data scientist, data engineer, researcher or Silicon Valley business leader focused on artificial intelligence, one way to improve your understanding of AI is to turn the pages of books that provide comprehensive information about the fundamental concepts and practical applications of AI and machine learning.
Many AI books offer insights into the technical processes and applications of AI, so we narrowed the choices to a more manageable six of the top books written by computer scientists and practitioners in the AI industry.
-
Artificial Intelligence: A Modern Approach (Pearson Series in Artificial Intelligence), fourth edition
Authors: Stuart Russell and Peter Norvig
First published in 1995, the fourth edition of Artificial Intelligence: A Modern Approach offers a wide-ranging examination of artificial intelligence. This edition provides coverage of the latest technologies, cohesively presents ideas and includes new and expanded information about deep learning, machine learning, transfer learning, robotics, multi-agent systems, natural language processing, causality, data privacy, fairness and safe AI.
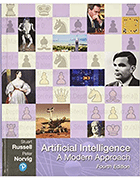
Considered a standard text in the field of artificial intelligence, this AI book has been used extensively in colleges and universities worldwide. Although it's intended for undergraduate students in courses such as basic artificial intelligence, statistical reasoning and robotics, it can also be used by graduate students. The textbook also covers probabilistic programming, logic and continuous mathematics as well as applications like robotic planetary exploration and microelectronic devices.
-
You Look Like a Thing and I Love You: How Artificial Intelligence Works and Why It's Making the World a Weirder Place
Author: Janelle Shane
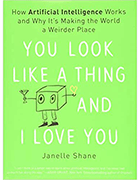
"You look like a thing and I love you" is one of the best pickup lines ever, according to an artificial intelligence program trained by Janelle Shane, a scientist and creator of the humor blog AI Weirdness. Shane develops AIs that learn how to create the best recipes, name paint colors and even flirt -- albeit badly -- with people. Shane aims to understand this technology that governs much of our everyday lives.
In her book, Shane offers real-world examples, illuminating cartoons, entertaining experiments and humorous assessments to explain what robots are thinking and how intelligent machines learn, fail, adapt and reflect the best and worst of humanity, and she provides the tools to question claims about AI's role in a smarter future.
-
Competing in the Age of AI: Strategy and Leadership When Algorithms and Networks Run the World
Authors: Marco Iansiti and Karim R. Lakhani
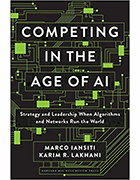
Competing in the Age of AI focuses on the increase in data-driven decision-making and the digital transformation of brick-and-mortar businesses. It includes a new preface examining how the COVID-19 pandemic has forced organizations like Verizon, Ikea and Massachusetts General Hospital to rapidly transform their business models.
The AI book explains how reinventing a company's traditional business processes into operations based on data, analytics and artificial intelligence eliminates the typical constraints on scope, scale and learning that have limited growth for decades and drives more accurate, complex and sophisticated predictions. It also examines how "collisions" between analog and AI-driven companies reshape competition, alter the economy's structure and compel traditional businesses to revamp their operating models.
-
Artificial Intelligence Engines: A Tutorial Introduction to the Mathematics of Deep Learning
Author: James V. Stone
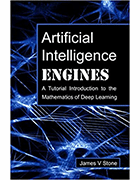
Artificial intelligence engines provides information on how to build, use and think about deep learning networks, illustrating key neural network learning algorithms with detailed mathematical analyses. Topics include historically important neural networks such as perceptrons, Hopfield nets, Boltzmann machines and backpropagation networks as well as modern deep neural networks like variational autoencoders, convolutional networks, generative adversarial networks and reinforcement learning.
Written in an informal style, the AI book contains a comprehensive glossary, tutorial appendices such as Bayes' theorem and maximum likelihood estimation, and a list of further readings. Online computer programs collected from open source repositories offer hands-on experience with neural networks, and PowerPoint slides provide teaching support.
-
Artificial Intelligence By Example: Acquire advanced AI, machine learning and deep learning design skills, second edition
Author: Denis Rothman
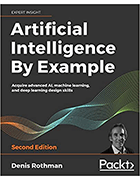
The second edition of Artificial Intelligence By Example studies some of the more advanced machine learning models to help readers understand how to create reliable programs, apply AI to blockchain and IoT, and develop an emotional quotient in chatbots using neural networks.
Targeted toward developers and others interested in AI who want to become adaptive thinkers and apply concepts to real-world circumstances, the book includes new examples of hybrid neural networks combining reinforcement learning and deep learning; chained algorithms combining unsupervised learning with decision trees; random forests combining deep learning and genetic algorithms; conversational user interfaces for chatbots; neuromorphic computing; and quantum computing. Readers should have experience with statistical knowledge and Python programming.
-
Fundamentals of Machine Learning for Predictive Data Analytics: Algorithms, Worked Examples and Case Studies, second edition
Authors: John D. Kelleher, Brian MacNamee and Aoife D'Arcy
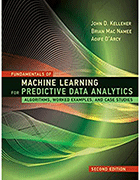
The second edition of Fundamentals of Machine Learning for Predictive Data Analytics provides data strategies, theoretical concepts, machine learning algorithms, mechanical predictive models and practical applications. It includes recent machine learning developments, particularly in a new chapter on deep learning as well as two new chapters that cover reinforcement learning and unsupervised learning.
The book describes four approaches to machine learning: information-based, similarity-based, probability-based and error-based learning. A nontechnical explanation of the concept behind each approach is followed by mathematical models and algorithms illustrated by detailed worked examples. Two case studies describe specific data analytics projects through each phase of development -- from formulating the business problem to deploying the analytics application.