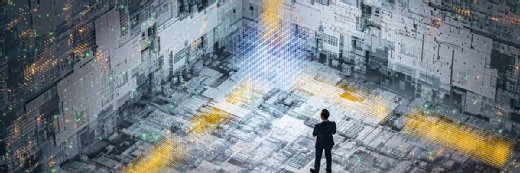
Getty Images
A guide to ChatGPT Enterprise use cases and implementation
ChatGPT Enterprise promises powerful generative AI capabilities for business use cases, but successful implementation requires careful planning for security, costs and integration.
An enterprise version of OpenAI's ChatGPT promises strengthened capabilities for business use cases, but how best to plan an implementation strategy remains unclear for many organizations.
With generative AI discussions already occurring in many organizations, ChatGPT Enterprise's launch in late August 2023 attracted widespread interest across industry sectors. However, extending the AI tool's reach into sensitive business settings adds new security, compliance and integration considerations.
To get the most out of generative AI while mitigating risks and challenges, organizations need to develop a comprehensive plan rather than getting caught up in the hype. With the launch of ChatGPT Enterprise, a structured implementation framework remains critical for success -- just as it was for previous game-changing technologies such as SaaS and cloud that are now integral elements of many businesses.
ChatGPT Enterprise overview
ChatGPT Enterprise is the latest addition to OpenAI's lineup, joining the free and Plus editions of ChatGPT. According to OpenAI's website, the company is also planning to launch a ChatGPT Business tier, described as a self-serve tool for smaller teams.
The following are some of the key features differentiating ChatGPT Enterprise from the other editions:
- Enterprise-grade security and privacy. In ChatGPT Enterprise, customer prompts and company data are not used to train OpenAI models. Data is also encrypted at rest (AES 256) and in transit (TLS 1.2+), and the software is certified SOC 2 compliant.
- Management features for enterprise administrators. ChatGPT Enterprise offers an admin console for bulk user management, support for single sign-on and domain verification, and an analytics dashboard for usage insights.
- Improved availability and performance. ChatGPT Enterprise users can run the GPT-4 large language model (LLM) with no usage caps and at higher speeds -- up to twice as fast, according to OpenAI.
- Data analytics and development features. ChatGPT Enterprise offers unlimited access to advanced data analysis, formerly known as Code Interpreter, and free API credits for enterprise developers looking to further customize ChatGPT.
- Longer, more detailed prompts. ChatGPT Enterprise extends ChatGPT's context window to 32,000 tokens, quadrupling the potential length of initial user prompts, files and follow-up questions.
- Reusable templates and workflows. ChatGPT Enterprise promises shareable chat templates for internal collaboration and the ability to build custom workflows, which could help users take advantage of the technology without needing advanced prompt engineering skills.
OpenAI has yet to publicize a ChatGPT Enterprise roadmap. However, the product page hints at possible future developments, including the ability to connect ChatGPT to company data via secure integration with other enterprise applications, more powerful data analysis capabilities, and features for specific job functions such as marketing and customer support.
Implementing ChatGPT Enterprise: A primer
Implementing ChatGPT at the enterprise level introduces security and compliance concerns, as well as the stakeholder and employee questions that come with any introduction of generative AI. Mitigate these issues by using the following implementation framework to roll out ChatGPT Enterprise.
1. Analyze and define the implementation plan
When implementing a generative AI application of the scope that ChatGPT Enterprise promises, an analysis and definition phase is essential. Some critical steps in this phase include the following:
- Analyze and define the organization's use cases for an LLM and map out the existing enterprise architecture.
- Determine any industry compliance standards or security protocols that the implementation must follow, such as HIPAA or Sarbanes-Oxley Act.
- Identify where relevant corporate data resides and how ChatGPT Enterprise will access and consume that data.
- Recruit a pilot team from both the IT and business sides of the organization.
- Engage the cybersecurity team throughout the implementation process to weigh in on all security and compliance questions.
2. Review and negotiate pricing, licensing and contracts
Expect that ChatGPT Enterprise licensing and contracts might continue to evolve, even for organizations that aren't among the first wave of customers implementing the software. Take the extra time to perform a thorough legal review of the licensing and contract terms, especially where it concerns AI and the security of corporate data.
Unfortunately for those wondering how much ChatGPT Enterprise costs, there's no public pricing available for ChatGPT Enterprise yet. Instead, the company refers potential enterprise customers to the sales team; OpenAI's COO has said that the company will work with customers on a pricing plan depending on the business's needs.
This situation, while typical for startup SaaS vendors, requires organizations to bring their own pricing expertise to the table. This could add another challenge, depending on in-house knowledge and skills. It also raises the potential threat of a price increase surprise at renewal time unless pricing is locked in during initial negotiations.
3. Plan to integrate ChatGPT Enterprise
Planning to integrate ChatGPT into existing applications and systems is a critical step. At the time of publication, there's no information as to whether OpenAI will have professional services partners to handle this work.
The top priority should be API integration: determining how ChatGPT Enterprise will communicate with other corporate applications and back-end systems via APIs. It's also essential to define the data ingestion process that will enable ChatGPT Enterprise to securely consume and update necessary data while adhering to compliance standards. The organization must also plan for implementing secure authentication to ensure only authorized users can access ChatGPT Enterprise.
4. Customize the model and train personnel
Training has a dual meaning when implementing an enterprise generative AI solution.
First, expect to spend some time fine-tuning the base LLM on the organization's data to ensure that model output is more domain specific. For example, a niche engineering firm will need to train ChatGPT on the terminology specific to the company's field. This process often involves collecting training data to ensure good performance.
Next, during or shortly after model customization and training, it's essential to train stakeholders and end users on the new generative AI system. Stakeholder training should target managers and executives, with an emphasis on the business realities and value proposition for generative AI, whereas user training should focus on job-related use cases. Plan to create job aids for knowledge transfer to help new and skeptical generative AI users get started, and prepare the service desk team to handle inquiries about ChatGPT Enterprise.
5. Conduct a pilot or proof of concept
Don't get lost in the generative AI hype. As with any new tool, whether from a startup or an established enterprise vendor, there's no getting around the need for a pilot or proof of concept inside the organization with real users.
When planning a ChatGPT Enterprise pilot, consider getting creative with the scope. For example, include marketing, analytics and sales use cases. Tying the pilot to scenarios that can help make sales more efficient should answer the questions of generative AI proponents and naysayers alike with verifiable facts and data.
6. Go live
Teams' work in the previous phases culminates in ChatGPT Enterprise going live in the organization. During this phase, project stakeholders and the service desk will be busy supporting users as the new generative AI tool joins existing workflows. Be sure to have feedback channels in place so that teams can learn what is and isn't working when it comes to generative AI adoption.
7. Engage in continuous learning
Continuous learning takes on new meaning when implementing generative AI in the enterprise. Marketing messages fade as teams cut through the noise by doing the actual work. All signs in the information that OpenAI has released thus far on ChatGPT Enterprise point to more learning being key to implementation success.
Will Kelly is a technology writer, content strategist and marketer. He has written extensively about the cloud, DevOps and enterprise mobility for industry publications and corporate clients and worked on teams introducing DevOps and cloud computing into commercial and public sector enterprises.