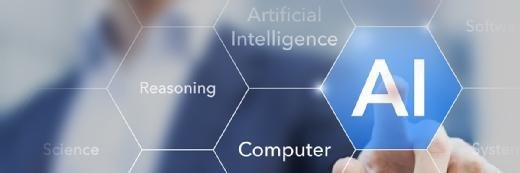
NicoElNino - Fotolia
AI helps supply chain analytics make better predictions
IoT sensors carry more data than ever into SCM platforms, making analytics simultaneously more complicated and effective. Machine learning is the indispensable helper.
What if you could apply machine learning and other types of AI to the terabytes of transactional and sensor data being collected from the supply chain? The result could be a much more autonomous and effective form of supply chain analytics and, ultimately, a more responsive supply chain.
In fact, there is a lot of interest in using AI and machine learning to enhance supply chain analytics, according to David Simchi-Levi, professor of engineering systems at MIT.
Much of the focus in the supply chain is driven by the ability to integrate predictive and prescriptive analytics to, in essence, blend AI and optimization technologies.
Specifically, organizations are using AI in two areas of the supply chain: to enhance predictive analytics to understand behaviors and for prescriptive analytics, where optimization technologies take input from machine learning and try to help decision-makers make better decisions, Simchi-Levi said.
For example, a fashion retailer announces it's going to increase revenue significantly in the next few years. The challenge is forecasting for improving sales and improving supply chains, he said. The retailer realizes it's not going to be able to do it by adding people. The question AI can help answer is, "Can I grow my business without significantly increasing the number of people we have?"
In supply chain management, the opportunity comes from the ability to use machine learning to improve customer prediction and demand prediction. Better demand prediction has a big impact on the supply chain, beyond just understanding customer demand, Simchi-Levi said.
"What's important in machine learning is the fact that not only can I come up with a forecast, I can also report what the level of confidence is that's associated with the forecast," he said. "I may tell you that the forecast is that the next quarter we will sell 50 articles of this product, but I can also tell you what the level of confidence is in this. Given these two pieces of information, it tells you that you need to redesign your supply chain strategy."
Supply chain analytics for better demand planning
Organizations could also adopt machine learning to improve demand planning technology by enhancing the accuracy of the predictions or identifying patterns that are hard to find, according to a November 2018 report titled, "A Supply Chain Analytics Leader's Due Diligence Checklist for AI Projects," written by Gartner analyst Noha Tohamy.
"Natural language might be embedded within a purchasing tool to provide purchasing managers with additional insights on suppliers and buying contracts in a more consumable manner," Tohamy wrote in the report.
Applying AI to the increasing volume of data from IoT sensors is another promising trend in supply chain analytics.
AI and IoT can enable companies to monitor and manage supply chain processes, said Terence Toland, an associate with the global business policy council at Chicago-based A.T. Kearney, in an email.
"Per our 2017 State of Logistics Report, many third-party logistics providers use embedded sensors to track shipments and improve forecasting accuracy," Toland said. "Similarly, air freight uses smart sensors that provide real-time location updates, which prove useful for time-sensitive shipments."
Sensor-enabled assets can provide real-time insight along the supply chain all the way to the end user, he said. Sensor data can also be used to create digital twins, where a software model is made of a physical object. The applications of these digital twins include predictive maintenance and improved product development.
"Given the rapid proliferation of IoT devices, we anticipate that such applications will become more widespread," Toland said.
Combining AI with IoT technology can also improve efficiency, while reducing maintenance and downtime. IoT and data analytics help improve asset performance by gathering data and converting it to useful information in real time, Toland said, citing another A.T. Kearney report.
"Integrating IoT with AI and data analytics will improve production efficiency by shortening production lead times and cycles," he said. "Companies can use advanced data analytics and AI to produce continuously, helping reduce overall costs and increasing productivity."
Organizations can also use AI to identify areas of improvement and forecast demand and inventory levels in a number of ways, according to Toland. AI-powered robots, for example, will be able to adjust production to shifting consumer demand.
A.T. Kearney has recently noted growing cases of companies using AI-powered predictions to provide decision-makers with data from point-of-sale systems that identify the precise SKU volumes being sold through specific stores, he said. These capabilities enable companies to base decisions on more accurate forecasts.
"AI and digitization will play an ever more important role in improving supply chain analytics," Toland said, quoting figures from his company's survey of global executives. Half of respondents identified AI and machine learning as providing the greatest area of opportunity among emerging technologies in the year ahead -- nearly double the previous year's response.
However, these capabilities should be developed alongside a capacity to "sense and pivot" to changing conditions on the ground. There will always be wild cards and shocks to the operating environment that even the best AI and predictive analytics can't anticipate, according to Toland.
"In addition to investment in these digital capabilities, companies must take steps to integrate flexibility and adaptability across their supply chain," he said. "This means developing the capacity to rapidly make adjustments to planning, manufacturing, distribution and logistics as conditions and demand patterns shift."
Applications in logistics, transportation management
The first thing Bill McBeath thinks of when it comes to how AI can improve supply chain analytics is demand forecasting.
"It's an area where we are seeing AI being applied, said McBeath, chief research officer at ChainLink Research, based in Newton, Mass.
Before AI, forecasting experts sought out algorithms they thought were the best to solve each problem, he said. With AI, they can start to take in data more quickly and let the AI algorithms find patterns they're not seeing with traditional algorithms.
Companies are also starting to use AI to get more precise estimated times of arrival, according to McBeath.
"For example, I've got a truck going across the country, and traditionally, this route takes [X days] long. If people are smart, they know that during a certain time of year, it takes longer or it takes less time on a particular day of the week," he explained.
AI can help make route planning more accurate by going through a whole bunch of data and starting to notice such deviations from the average at different times of year, McBeath said.
In addition, AI can help companies use supply chain analytics to prioritize shipments more effectively.
"Let's say you have information about 10,000 shipments per week that are coming into your company, and you want to know which ones are late," McBeath said. "That's hard for a person to do, but easy for an AI machine to do. But you don't care about all of them that are late, so [you can use AI] to filter those, then maybe prioritize them by impact on revenue or impact on key customers."
AI helps companies take huge quantities of data and know what's important to make better decisions. Then, they can observe the outcomes of those decisions, start to figure out which decisions are working and which aren't, and ultimately decide if they should do something differently, McBeath said.