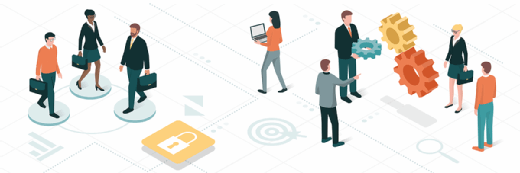
8 considerations for buying versus building AI
Business leaders should consider their employees' technical expertise, technology budgets and regulatory needs, among other factors, when deciding to build or buy AI.
Many companies across a variety of industries understand the benefits AI can bring to their organization. For example, using AI, enterprises can increase efficiency, gain better insights into customers and their buying behaviors, improve their customer service and enable predictive maintenance of equipment and machinery, among other benefits.
However, not all organizations have the resources or funds to create custom AI products for every need.
For businesses, deciding whether to buy off-the-shelf AI or build their own requires careful thought. Businesses should consider numerous factors, including their available talent, their timeframe, the cost of building versus buying AI, vendor lock-in factors and the business use cases they want to solve with AI.
While there are many advantages to using off-the-shelf AI software, there are times when building software may make more sense due to domain expertise in niche markets, regulatory issues or creating core AI tools that provide key differentiators from peers and competitors.
To make the best decision for their organization, implementers and leaders should understand the pros and cons of building AI versus buying AI.
Open source vs. proprietary software
First, business leaders need to understand available AI platforms and their benefits and disadvantages.
Open source software and AI tools such as Python, TensorFlow or scikit-learn make it easier and cheaper for companies to build AI from scratch.
Open source software is usually well supported by a community of passionate developers using and improving on these tools. Open source software also lets companies build AI-specific tools that they can house on premises, which is important for building applications that require on-site software. Building in-house also offers greater flexibility and gives the creator ownership over the intellectual property and full control over the data sets used to create models.
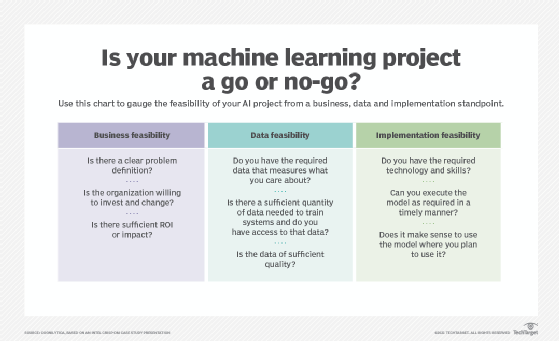
Yet, not all organizations have the in-house know-how needed to build their tools. That's when commercial AI platforms become useful.
Vendors that build AI tools into their platforms already generally have access to a vast pool of very well-organized training data. Additionally, established vendors of enterprise tools benefit from extreme economies of scale. As a result, they can afford to hire very specialized talent to develop and improve their AI models. Many vendors also offer free or low-cost training on their platforms to keep employees updated on all the latest offerings.
Factors to consider when evaluating building versus buying AI systems
When weighing the factors of building versus buying AI software tools, the enterprise should include stakeholders and users of the software to get their input in the decision-making process to ensure it gets used and adopted. This way, developers aren't guessing which features and functions are important, as the end user is giving them direct feedback. It's also important to understand that it's not an all-or-nothing answer. Sometimes it may make more sense to build while other times it makes more sense to buy. Below are eight considerations to take into account when deciding to build or buy AI functionality.
- Availability of talent. One of the most important factors businesses need to consider is the availability of skilled talent. Do you have more expertise around open source software or deeper bench strength on commercial vendor products?
- Timeframe to end solution. Knowing when you need to have a product ready to go is also an important factor to consider. Do you have the time to wait months or years until a tool is ready to use? If so, then building in-house might be a good option. If you need functionality ready to go tomorrow, buying will be your best bet. When scoping out your project, make sure to set clear objectives and requirements to ensure everyone is on the same page.
- Integration with existing software. It's crucial to understand if the vendors you currently work with can integrate with the AI tools you are considering. If a vendor offers an AI tool you don't currently work with, make sure to understand your organization's procurement process and allow sufficient time for the process and for contracting itself. This may add considerable time to your project, and you need to make sure you can support the time delay.
- Vendor lock-in. It's important to question potential AI vendors about data and model ownership to avoid vendor lock-in. If their answers aren't to your liking, it might make more sense to build a tool. In addition, since AI technologies are changing so rapidly, locking in with one vendor may actually become a disadvantage for your organization if that vendor doesn't keep up at the pace you need.
- Total cost of ownership. The discussion around building versus buying AI should always address total cost. While using open source tools may seem cost-effective, build-it-yourself AI can cost millions of dollars once you factor in compute resources and employees needed to create the functionality and the associated ongoing maintenance costs.
- The complexity of the problem. If you have a common problem that numerous vendors have already solved, then creating a tool from scratch probably isn't your best use of resources. Tasks such as sorting different types of documents, natural language processing and chatbot software, or general computer vision related products have all been built and well-tested at this point and it usually makes the most sense to buy instead of build here.
- Regulatory considerations. Heavily regulated industries, such as healthcare, finance and government, might want to consider building their own AI tools, to ensure their tools adhere to their strict guidelines and regulations.
- Cloud vs. on premises. As a follow on to the above consideration, for industries that are heavily regulated such as healthcare, finance or government, there are regulations in place that require organizations to keep sensitive data on premises. In these cases, it might make the most sense to build in house. However, for the majority of companies, cloud-based prebuilt software will offer a more affordable option.
Cost-effectiveness and long-term maintenance
Make sure to do your research and know what software already exists. Understand the strengths and limitations of your team. Consider the long-term maintenance of supporting your own AI tools and make sure the overall costs make sense from a technology and people perspective.
Will this product be a key differentiator in your success against your competitors? If so, then the costs associated with maintenance make sense. Will the functionality become available by a tool you already pay for, or worse, is it already available? If so, the costs to build and then maintain this software don't make sense. By weighing the various factors and considerations when deciding to buy AI tools rather than build, you'll make an informed decision that's right for your organization.