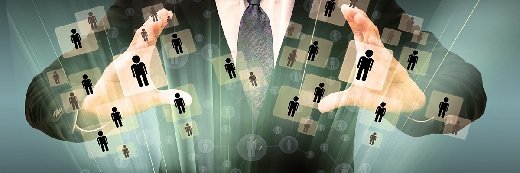
sommai - Fotolia
The peaks and pitfalls of hyper-personalization marketing
As consumers begin to revolt against unlimited personal data collection and usage, the longevity of hyper-personalized communication may be cut short.
Enterprises, government agencies and organizations are applying AI to develop more focused and detailed profiles of individuals other companies and more. Some of these deeper profiles help improve marketing and advertising conversion rates, while other profiles are being used to provide more optimized healthcare, finance, insurance or retail.
The application of AI to build these constantly learning profiles is referred to as hyper-personalization, a method by which systems collect data from a variety of sources to build a unique profile of an individual. This approach is opposed to the traditional "bucketing" approach where individuals are grouped into categories based on some shared characteristic, such as age, income or location. While bucketing approaches can work, they are very generalized and rarely applicable to the needs of individual users. If you can focus on one individual using profile data gathered specifically for that individual, you can serve and target them much better.
While hyper-personalization marketing offers significant benefits for individual optimization, it can also pose significant concern for those who are worried about the increasing amount of information that organizations are collecting.
Positive use cases
Amazon and other e-retailers pioneered the use of personalized profiles to improve product recommendations and tailored search results. Users who might be interested in camping tents are also offered other kinds of camping equipment based not only on their own buying behaviors, but also based on the patterns learned about customers like them. While there have been rules-based and database-centric methods for doing personalization by using product categories, the sheer quantity of new products being added to the catalogs of companies like Amazon or Walmart encouraged these companies to move away from human-centric marketing. Instead, businesses are using machine learning-based approaches that can not only do a more accurate job of identifying products that are similar, but can also uncover hidden patterns of purchasing behavior. Any online retailer looking to offer such a similarly wide array of constantly changing products has learned the power of AI-based systems to do such tasks more reliably, in a scalable manner and cost-effectively.
Hyper-personalization is also being used with potent effect in marketing and advertising. Rather than segmenting audiences based on general categories, online advertisers are tapping into the power of machine learning-enhanced profiles to create an ideal scenario with the right product, timing, channel of communication, offer, content and targeted consumers. Companies and websites use intelligent algorithms to tailor ads for potential customers by not only rehashing advertisements on products or services they've already shown interest in but by presenting new versions of content to optimize conversions. Rather than using more human-based, static "A/B testing" approaches, the use of machine learning-driven hyper-personalization allows an almost infinite number of combinations of content, channel, audience, and offer to learn individual patterns and present the right combination to a specific user.
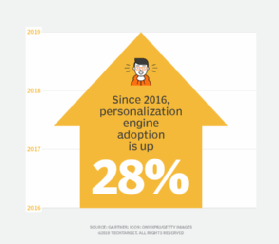
AI systems are also being used to tailor content for other industries. Netflix uses machine learning-based hyper-personalization to optimize and present custom content to each individual user. The system finds patterns in customer viewing and selection behavior, pairing this data with millions of other people's data sets in order to create unique groupings that take the common preferences of these groups and suggest them to all members. Instead of broad groupings of movies such as horror or comedy, this hyper-personalization allows Netflix to create niche categories such as "Victorian romance thriller musicals" or "science fiction movies of the 1960s with B-level celebrities". By focusing on the specifics of what each customer wants, Netflix can encourage more usage of its vast library of content, thus retaining customers as ongoing subscribers and increasing their satisfaction.
These associations between individual buying habits and those of others that are similar are known as affinities. Starbucks' emailing algorithm is one prominent example of using affinities to make a huge number of hyper-personalization marketing emails using data gleaned from customer's past purchases. Rather than crafting only a few dozen emails on a monthly basis with offers for the broad Starbucks audience, Starbucks is using their "digital flywheel" with AI-enabled capabilities to generate over 400,000 personalized weekly emails featuring different promotions and offers. Promotions are no longer just based on location or self-selected interests, but rather on detailed and individual customer behavior.
Hyper-personalization is also being increasingly applied to important areas of concern like our health and wealth. Personalized medicine is using genetic data as well as patient behavior data to recommend medical treatments and augment healthcare with solutions that are more tailored for each individual patient's lifestyle and wellbeing. Likewise, personalized finance tools claim to help people better manage their finances by evaluating their individual purchasing, investing and saving behavior and recommending optimized allocations of assets and expenditures to maximize savings goals and spending budgets. In this way, AI acts as a personal assistant helping people in their daily lives by learning patterns and recommending solutions.
When do we cross the line?
Given that AI systems are keeping track of what we buy, how we shop, our finances, our health and even the content we consume, are we crossing into territory where AI systems and the companies that run them know too much about us? The ever-encroaching amount of data tracking can certainly be overwhelming. Trust in companies and corporations is dubious at best, and consumers are starting to push back -- some through 'de-googling' themselves, deleting social media accounts or cutting the cord to big service providers.
However, disconnecting from the increasingly connected and profiled world does not seem like a realistic possibility. Enterprise interests are deeply rooted in hyper-personalization marketing. As consumers, the gains received from companies offering products that are relevant to us, content that we're interested in reading and watching, healthcare and finance solutions that are better suited for our specific circumstances and needs come at the price of someone having to store some data about us somewhere. The challenge comes from making sure companies don't store more information than is necessary, and disclosing when and how that information is stored, shared and used. As data usage and privacy continue to come to the forefront, these topics will likely be addressed.