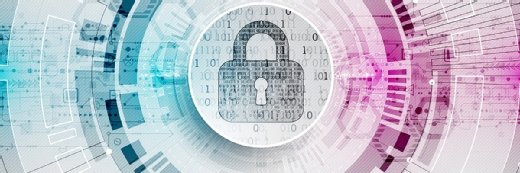
Alex - stock.adobe.com
What is data risk management? Key risks and best practices
Data risk management identifies, assesses and mitigates threats to organizational data, safeguarding sensitive information from unauthorized access.
A specific form of risk management, data risk management typically applies to data privacy, security, acceptable use policies, legislative directives or regulatory compliance. The intent is to protect sensitive business data from unauthorized access and prevent changes that compromise data integrity.
Effective data risk management practices ensure data availability through adequate storage, safeguard against loss, such as deletion and stop malicious use.
What is data risk management?
Data risk management is the set of processes and workflows used to identify, assess, mitigate and monitor risks specifically related to an organization's data assets.
Properly administered, data risk management combines several important elements, including:
- Data identification and classification. An organization must clearly understand the types, amounts, locations and sensitivity of its data assets. This understanding underpins risk management because unknown risks are unmanageable.
- Risk assessments. Each data type's risk assessment carefully evaluates the risks to privacy, security, availability and compliance. For example, a customer database includes sensitive, mission-critical information, while public corporate marketing materials pose less risk to the business.
- Data governance. Data, as a business asset, requires the creation and enforcement of data governance rules—rules that outline the proper use, handling and storage of each data type. Different data classifications often have specific rules that not only provide company-wide guidance for the data but also a foundation for regulatory compliance.
- Mitigation strategies. After scrutinizing each data type's risks, business and technology leaders set risk mitigation policies in motion to address operational concerns. For example, the critical database warrants investment in technologies to ensure redundancy and security protections, such as identity and access management (IAM). This database also uses data protection technologies and provides real-time logging and alerting access. Well-documented mitigation strategies further demonstrate regulatory compliance.
- Monitoring and reporting tools. Data risk management often employs software tools to monitor data access – what was retrieved, when and by whom – and create reviewable logs. Improper access triggers real-time alerts and preventive security measures.
Why is data risk management important?
Data is critical to any modern business. By considering data as an asset, similar to a building or computer, the idea of attaching risk to it becomes sensible. Data can be lost, inaccessible, stolen or damaged. Just as a business requires a serviceable structure or suitable IT infrastructure, it also needs access to critical, often sensitive, data.
Data risk management recognizes business data as an asset requiring protection and ensuring organizational stability. Its numerous benefits include:
- Operational continuity. Data risk management supports business continuity. Required data is complete, accurate and available on demand, courtesy of resilient storage techniques such as RAID and software tools that monitor security and safeguard data integrity.
- Maintaining security. Data risk management drives policies, procedures and techniques that secure business data against theft or loss. Sensitive data access typically requires suitable authentication and authorization, and access is monitored and recorded to prevent malicious activities.
- Ensuring compliance. Modern businesses face an array of regulations. Some protect individuals and their confidential data. Others support successful ongoing business operations. Most regulations impose fines and legal penalties for non-compliance. Data risk management works to achieve compliance.
- Enhancing the business. These outcomes cultivate the trust of customers, business partners, regulators and other stakeholders. Moreover, a strong risk management posture speeds response to breaches or data loss, mitigating impact.
Key types of data risks
Data risks typically fall into three broad categories: theft, misuse and technical failure. Each risk exposes a business to reputational damage, financial loss and legal penalties. Each also presents a unique challenge.
Theft
Data theft includes any action that enables unexpected or unapproved access to sensitive data, including:
- Hacking. A hacker gains unauthorized access to an organization's security to view, steal or alter data, often using methods such as phishing.
- Malicious software. Malware penetrates an organization's security, installs and runs on a system to facilitate unauthorized access, perhaps a keystroke logger to capture user credentials.
- Human error. Data is exposed following employee action or inaction. For example, a misconfigured IAM platform, storage subsystem or network gear enables unauthorized access.
Misuse
Data misuse occurs when data is used or shared in unintended ways, including:
- Data leakage. Protected data is incorrectly shared with unauthorized parties. For example, an employee sends a file to a vendor, unaware of the enclosed data's sensitivity.
- Improper intent. Sensitive data is accessed for purposes inconsistent with normal job functions. For example, an employee may look at a friend's health records even though the employee isn't a healthcare professional or involved in care.
- Third-party access. Organizations commonly provide data access to third parties such as data processing vendors. Once data is shared – even for intended purposes – its theft, loss or misuse has the same consequences for the originating business. Modern data sharing agreements often require third parties to demonstrate the same level of protection.
Technical failures
The complex technical infrastructure handling this data often involves an array of hardware devices and software components. All interconnect through detailed configurations. Failure, even vulnerability, in any aspect poses a risk to data security and accessibility. Common risks include:
- Poor configurations. Elements are not properly configured to interoperate, creating vulnerabilities or limiting reliable access to data. For example, leaving a network device with a default administrative password lets an attacker access the network.
- Unpatched software. Software's well-known vulnerabilities attract attackers. It's important to patch critical software tools quickly to mitigate known vulnerabilities.
- Weak passwords. Modern computing requires credentials to access data. Many organizations require strong passwords that are changed regularly, avoiding easily determined or publicly known defaults.
- System failures. Hardware faults – from a crashed server to a failed storage device – leave critical data inaccessible. Vital data requires a resilient infrastructure, including redundant servers or storage devices, capable of tolerating some hardware failures. Continued access to data maintains normal business operations until the fault is identified and corrected.
How AI is affecting data risk management
The emergence of machine learning and artificial intelligence (AI) profoundly impacts data risk management. Broadly speaking, AI technologies provide three principal benefits in the modern business world:
- They process enormous amounts of data from varied sources to find patterns and relationships.
- They act autonomously in response to that situational data in context.
- They respond in a fraction of the time needed for human administrators to react.
Therefore, AI is a natural fit for data risk management tasks, enhancing the identification, assessment and mitigation of data risks.
In cybersecurity, for example, AI analyzes and models vast amounts of log and network data to find characteristic signatures of possible intrusion; it then takes real time steps to block and report subsequent incidents to mitigate the possible attack. In another example, AI checks outgoing email, looks for protected data in any attachments and understands how data leaks occur. The AI blocks such attachments, then sends messages to the senders about the importance of data security and data risk management.
However, AI also poses several challenges, including:
- It needs extensive, complex and computationally intensive training, requiring substantial upfront investments of financial and intellectual capital.
- It must be trained and validated to address and eliminate bias, first in its training data, then in its algorithms and decision making.
- It must be able to learn and adapt to changing business needs and risk profiles, requiring ongoing training and continuous monitoring by humans to ensure stable and reliable outcomes.
- It is not 100% accurate. At times, AI makes mistakes or misinterprets behavioral or situational data.
- It does not relieve the business from compliance obligations. For example, if a data breach occurs because of an AI error, the business remains fully responsible for fines, litigation and other penalties.
Ultimately, AI technologies refine and accelerate data risk management techniques, but they're not perfect, nor do they remove humans – or human error – from data risk management tasks. Today, AI is a useful tool to enhance data risk management, but AI systems cannot operate autonomously.
Best practices for data risk management
Data risk management is a complex endeavor with demands that vary widely depending on industry requirements, business size, regulatory obligations and staff skills. While there is no single playbook for proper data risk management, there are some best practices, including:
- Assess risks frequently. Business data constantly grows and changes, making data risks a dynamic threat. Establishing a frequent data inventory and reviewing data assets – types, locations, value and vulnerabilities – are important foundations needed to understand what's at risk and identify the risks and their consequences. Businesses use assessments to design safeguards, raise employee awareness and carry out mitigation strategies.
- Control data access. Zero-trust policies and access controls provide reliable authentication and limit authorizations strictly to data needed to perform the user's job. Common access techniques include role-based access control and attribute-based access control, which grant access to broader user types rather than individuals.
- Monitor data quality and access. Data is altered and corrupted even during legitimate access, so regular data quality monitoring ensures that all data remains complete, consistent and accurate. Also, monitoring and logging data access helps match users to data quality issues, clarifying what data is accessed and by whom.
- Leverage AI. Advanced data risk management platforms, often based on AI technologies, analyze data quality, review access patterns and spot unusual behaviors that potentially threaten data access and security. Advanced platforms alert administrators and take autonomous actions to mitigate potential threats. Other technologies, such as data loss prevention, stop sensitive data from leaving the business using upload or email attachments.
- Employ end-to-end encryption. Tools with low computing overhead, including data encryption, protect data both at rest and in motion. End-to-end encryption is especially beneficial if sensitive data must be shared outside of a business's local area network.
- Educate everyone. Every employee and third-party partner plays a role in recognizing, understanding and managing data risks. Necessary education includes ongoing training and regular audits to reinforce the importance of data risks. These processes also promote the creation of an incident response team to handle data breaches.
Communicating data risk management at your organization
Data risk management requires effective communication across an organization. Keep the following practices in mind when communicating the importance of data risk management:
- Tailor the message. Different stakeholders need different types of messages. C-level executives are primarily concerned with strategic issues – financial, legal and reputational risks. Rank-and-file employees want news about specific policies that affect their everyday jobs. Outside parties typically focus on trust issues attached to risks that require constant monitoring and occasional response.
- Keep the message clear. Regardless of the message, clarity is required. Communication must be well supported with practical terms, examples and procedural steps appropriate for the various stakeholders.
- Develop a risk-aware culture. Use regular communication, as well as ongoing training and education, to foster a corporate culture that empowers individuals to act, prevent data risks if possible and mitigate their impact when they occur.
Stephen J. Bigelow, senior technology editor at TechTarget, has more than 30 years of technical writing experience in the PC and technology industry.