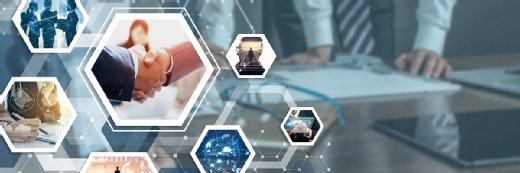
metamorworks - stock.adobe.com
Custom generative AI models an emerging path for enterprises
Custom enterprise generative AI promises security and performance benefits, but successfully developing models requires overcoming data, infrastructure and skills challenges.
Consumer-facing pre-built generative AI models such as ChatGPT have attracted mass attention, but customized models could ultimately prove more valuable in practice for organizations.
Most companies' generative AI initiatives are in the early stages. Only 4% of organizations currently have generative AI in mature enterprise-wide production, according to recent research from TechTarget's Enterprise Strategy Group (ESG). The majority (54%) remain in early deployment, pilot or planning stages.
As businesses increasingly explore generative AI, many are recognizing the value of aligning models to their specific data and use cases. The same ESG survey revealed a preference for customization, with 56% of respondents planning to train their own custom generative AI models rather than solely relying on one-size-fits-all tools such as ChatGPT.
But successfully developing custom enterprise generative AI entails major challenges in areas from data management to security to systems integration. To address generative AI's risks and limitations while availing themselves of the benefits of custom models, businesses will need to take a targeted approach to deploying this emerging technology.
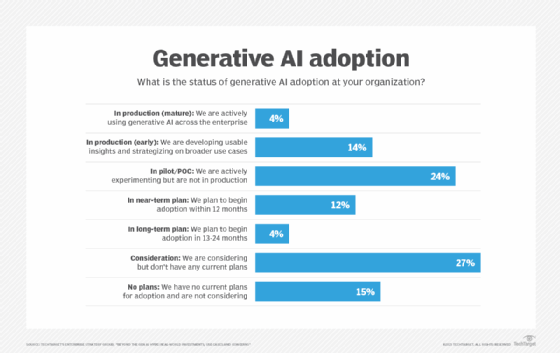
Benefits of custom enterprise generative AI models
There are several compelling reasons to develop a custom enterprise model:
- Stronger privacy and security.
- Better performance through fine-tuning on proprietary internal data.
- Easier integration due to the ability to tailor AI tools to the idiosyncrasies of a company's workflows.
In particular, generative AI's privacy and security risks are a major concern for enterprises, with one IT/cloud manager in the financial, banking and insurance sector describing existing generative AI tools as "too much of a security risk" in response to ESG's survey.
"Many of the available AI technologies are no cost or are being enabled by existing vendors -- without the organization having a chance to proactively review the risks of the tech in light of data privacy, security, compliance, confidentiality and IP considerations," said another respondent, a business vice president in the telecommunications sector.
Fine-tuned proprietary models offer security-conscious organizations better oversight when it comes to the internal data used to train models. With in-house models, organizations maintain control over sensitive data, rather than sharing access with third parties.
Models fitted to company-specific tasks and data are also likely to produce more relevant outputs and fewer hallucinations. This could assuage some organizations' worries about achieving accurate, fair and representative output using third-party models.
"There are concerns about the accuracy and completeness of the AI reports," a consultant in the healthcare and health services sector said in response to ESG's survey. "How can we confirm and verify the sources of data? Plus, there are issues with algorithms containing bias."
Training a model on a targeted data set -- here, information about an organization and its industry -- in a process known as fine-tuning can yield more accurate results for related tasks. And AI tools tailor-made to address specific business problems and workflows could increase efficiency and reduce integration problems. Together, this means that custom models are likely to require less extensive oversight while producing outputs better matched to business needs.
Challenges of custom enterprise generative AI models
However, adopting generative AI isn't without its challenges. Respondents to ESG's survey cited a wide range of obstacles to generative AI implementation -- custom or otherwise -- led by a lack of employee expertise and skills (39%), ethical and legal considerations such as bias and fairness (32%), and concerns around data quality (31%).
Creating effective custom generative AI is an especially complex undertaking that will require overcoming three major obstacles: insufficient good-quality data, the difficulty of integrating models into legacy systems, and a lack of AI and machine learning (ML) talent.
Without sufficiently high-quality, extensive and well-integrated data, training an accurate proprietary model is likely to be difficult or impossible. Transforming messy corporate data into a usable training corpus is a process that requires substantial effort, involving constructing pipelines to ingest and prepare proprietary data to be meticulously labeled and fed into models.
This process of cleaning and labeling enterprise data is resource-intensive in itself, but constructing and maintaining model pipelines that can adapt to changes over time also requires significant ML expertise that remains scarce among current IT employees. A quarter of respondents cited technical complexity as a barrier to generative AI implementation in their organizations, and the limited supply of qualified ML and data science professionals compounds these technical challenges.
Any organization pursuing proprietary generative AI will need internal ML experts to refine data management practices and build training pipelines for custom models. ML operations, or MLOps, skills are also required after deployment for tasks such as monitoring model performance, addressing data deficiencies and bugs, and handling integration issues. But with corporations competing fiercely for a comparatively small pool of ML talent, hiring these team members might pose an obstacle in itself.
The path to effective custom generative AI
Enterprise generative AI remains in its early days. To avoid costly mistakes, organizations will need to cultivate patience and realism along with long-term vision.
Building a custom generative AI model is a complex and costly proposition that won't be the right choice for every business. When evaluating whether an in-house model development initiative is worthwhile, enterprises should weigh the potential benefits of generative AI against the resources required.
"We are still in a rather early stage to adopt AI," a business manager in the transportation industry said in response to ESG's survey. "While many people are talking about AI and are aware of the potential of AI, people ... are reluctant to adopt [or] invest effort to train AI."
Respondents expected their organizations would need to invest in a wide range of areas to support generative AI initiatives, including training and employee skills (47%); information management (44%); and data privacy, compliance and risk (37%).
But for enterprises with the need and resources to invest in ML infrastructure and talent, building custom generative AI can provide a competitive edge. Respondents expected a wide range of benefits from using generative AI in their organizations, including improving or automating processes and workflows (53%), supporting data analytics and business intelligence (52%), and increasing employee productivity (51%).
Extensive planning will be essential for success in generative AI initiatives. As a first step, take the time to carefully identify isolated areas where a custom generative AI tool could have especially high payoff. Next, build prototypes and conduct extensive piloting before expanding to a widespread deployment.
In ESG's survey, top enterprise generative AI use cases included data insights, chatbots, employee productivity and tasks, and content creation. One business executive in the computer services industry, for example, reported that their organization used generative AI to create content ranging from social media marketing to technical e-books to presentation slide decks.
Overall, the key is to start small and focused, with narrowly targeted models whose scope can be gradually expanded after proving value. Don't expect to build a sprawling internal ChatGPT; fine-tuning models on internal data sets for specific tasks is faster, less resource-intensive and more likely to demonstrate short-term returns.