The future of generative AI: How will it impact the enterprise?
Learn how generative AI will affect organizations in terms of capabilities, enterprise workflows and ethics, and how the technology will shape enterprise use cases.
Generative AI is a foundational technology that is already having an effect across enterprises. In the early days, the technology focused on creating fake humans and automating image creation processes. However, the advent of ChatGPT demonstrated that LLMs often exceeded the capabilities of previous natural language processing approaches that were slowly being adopted across the enterprise.
This enthusiasm was further buoyed by consumers' massive uptake of ChatGPT, which has experienced the fastest adoption of any application to date. But the power of these LLMs isn't limited to fancy chatbots that are better at sounding like real humans. The industry as a whole is starting to acknowledge that LLMs can also serve as universal translators across different domains to generate code, understand complex data sets and simplify user experiences across roles and for customers.
"Generative AI capabilities, such as prompt engineering and adaptive responses, are what set it apart from previous AI models we have seen," said Bharath Thota, partner in the advanced analytics practice at global strategy and management consulting firm Kearney. "With ChatGPT being introduced to the general public, we have seen a shift in the way that people now understand and interact with AI."
That said, the new technology comes with various challenges, such as a propensity to hallucinate, requiring guardrails and vetting by domain experts. It also introduces new ethical challenges around the use of responsible AI. Left unchecked, generative AI could also exacerbate existing issues with bias, privacy, security and public opinion.
Here are some of the different ways generative AI will change the enterprise in terms of capabilities, enterprise workflows, use cases and ethics.
Future of generative AI capabilities
Generative AI will enable the following enterprise capabilities.
The rise of self-teaching models
One of the attractions of LLMs was that they could discover patterns on vast unlabeled data sets on their own, at least as a starting point. The industry is waking up to the requirements for vetting and refining data or fine-tuning models for best results. Rex Chekal, principal product designer at software development consultancy TXI, expects innovations in smaller self-teaching models that compete with large data-hungry models, like GPT-4. One early example is Orca from Microsoft, which imitates the reasoning processes of larger models using progressive learning and teaching assistance to overcome capacity gaps. "For CIOs, using [LLMs] will be like hiring an all-star employee who continuously improves and is transparent about how they work," Chekal said.
Accessibility
Innovations in LLMs make it easier to customize information and experiences for a wide range of employees. "Generative AI has enabled all employees -- and not just technology and IT ones -- to access data and technology in an unprecedented way," said Benjamin Rehberg, managing director, senior partner, and global lead of tech and digital offerings at Boston Consulting Group (BCG). As a result, using AI tools without code or little code is increasingly becoming the new reality.
General to specialized models
The first wave of generative AI unleashed new models that were proficient across many tasks but suffered significant problems in particular domains. As generative AI moves into specific industries and fields, it will drive the development of models fine-tuned for particular purposes, predicted Anil Vijayan, partner at Everest Group. For example, banking, insurance and HR models will have a better ability to speak the languages of these narrower fields.
Applications built on foundational generative AI models
Vijayan also expects to see a proliferation of apps built on top of LLMs or conditioned LLMs to solve specific needs. Early examples include web navigation concierges and code development assistants. Early growth will start in B2C contexts. B2B and business-to-enterprise applications will see a spurt once risks are mitigated.
Rise of open source LLMs
The first crop of LLMs from OpenAI and others were relatively proprietary. More open models, such as Meta AI's Llama 2, provide viable alternatives that increase transparency, customization and cost-effectiveness. This trend should continue, according to Samuel Hamway, research analyst at Nucleus Research. For CIOs, this will mean more control over data and AI operations, but it will also require increased expertise in model management, maintenance, governance and hardware infrastructure.
LLM plugin ecosystems for augmented capabilities
Generative AI vendors, like OpenAI, are starting to support plugins that can augment the core capabilities of LLMs to become more task-specific. "This task grounding makes LLMs more capable for targeted tasks and useful across different verticals," Hamway said. These plugin ecosystems also simplify the integration of AI into existing workflows and streamline the deployment of AI-based offerings.
Improved search
A new crop of enterprise search tools uses LLMs to enhance access to relevant data. One key innovation will be improvements in vector databases that stage data that has been transformed into an intermediate format more accessible to LLMs. Hamway said enterprise search initiatives will be required to consider data retrieval mechanisms as a core competency to make data more actionable and insights timelier. CIOs should also consider how a unified data architecture could improve integration with LLM-powered search capabilities.
Future of generative AI in the enterprise
Here are nine specific ways generative AI will affect the enterprise:
- Upskilling. The rush to take advantage of generative AI will likely increase the pace of upskilling efforts. "AI upskilling paves the way for building an enterprise that fully understands how to use generative AI and the benefits the technology brings to the company," said Scott Likens, global AI and innovation technology leader at global consultancy PwC. He expects to see far more companies investing in training and upskilling for current employees in the future, especially in generative AI usage.
- Hybrid architectures. Likens also observed that generative AI is changing at a rate he has rarely seen. The increased pace of innovation in open source software and platforms from big tech companies means enterprises need to rethink how they test and scale these technologies. This also increases the use of hybrid architectures that are changing more rapidly than other digital transformations he has seen. These new approaches can shorten the time to value, but it's also important to design flexibility into new offerings.
- Changing the nature of expertise. Generative AI is automating an increasing number of tasks to boost productivity. Everest Group's Vijayan expects this to also change the nature of expertise. Enterprises will need to rethink their talent agenda, workforce planning, and learning and development programs. The bar of valuable human expertise will move from mastering things such as programming language syntax to higher-level understanding. Enterprises will need to rebuild their skills taxonomies and reassess how they plan for talent.
- Renewed focus on enterprise data strategy. The biggest gains of generative AI will require going beyond the low-hanging fruit of generating text, images and other media. Vijayan envisions a future where generative AI creates appropriate business or IT workflows, creates complex documents from scratch and generates marketing collateral specific to a company. These new use cases will require seamless access to enterprise data, regardless of the approach. "The enterprise data journey is not new, but GenAI will require a renewed focus and perhaps more investments to get there quicker," he said.
- Automation of job tasks reshaping jobs. Prior waves of automation built on robotic process automation and business process automation focused on automating whole processes. Generative AI could help spin up automation more quickly but might only be sufficient for some tasks now performed by humans as part of a larger process. Certain tasks will become fully automated, BCG's Rehberg said, while more complex work will require a human element. "We recognize that all revolutionary developments have not led to less jobs, just changes in the effectiveness and efficiencies of employees," he said. "It would be a mistake to believe that there will be less jobs available moving forward, rather some jobs will just be reshaped and evolve."
- Generative AI embedded natively into existing enterprise apps. Rajesh Kumar R, CIO at IT consultancy LTIMindtree, predicts generative AI will transform from standalone assistants, like ChatGPT, to something natively weaved into productivity applications, such as email, spreadsheets, content authoring tools, presentation tools and other core enterprise systems, such as ERP, CRM, HR management and recruitment systems. Microsoft's Copilot provides an early example of how this might unfold across different applications.
- Competition, disruption and lowered barriers to entry. Generative AI will enable more automation to help organizations do more with fewer resources. "As costs go down, fundamentally new business models become more feasible in multiple domains," Vijayan said. This is similar to how digital banks quickly started taking business from brick-and-mortar ones. It could be particularly disruptive in stock media, customer service, entertainment and other industries.
- The niche future of generative AI. Enterprises will likely see the best results in customizing LLMs for their own particular industry and use case. There won't be one general tool, such as a chatbot, used across industries, predicted Mona Chadha, director of category management at AWS. Rather, each organization will develop a generative AI offering that can deliver business outcomes catered to its base. "These tools will include highly domain-specific LLMs for consumers to use with their own contextual data," she said.
- Cost. The current crop of generative AI services is relatively more expensive than traditional search and natural language processing (NLP) techniques. However, Vijayan has already seen price drops by at least an order of magnitude and expects these to go down further as infrastructure, hosting, training and inference become more efficient and economies of scale further facilitate lowering costs.
Future of generative AI use cases
Here are some of the ways generative AI will shape various use cases within enterprises.
Research
Generative AI will have the greatest impact on jobs that focus on research, particularly those involving the largest sets of data, said Brian Spanswick, chief information security officer and head of IT at data security company Cohesity. This includes research relating to legal questions, scientific research, data governance and code development. This will also increase the emphasis on higher levels of critical thinking in day-to-day work. LLMs will do the heavy research lifting more completely across larger data sets virtually instantly. "Rather than spending the majority of people's time on busy work, the power of the employee will be in making strong decisions based on the data they have, with the knowledge that that data is trustworthy," he said.
Cybersecurity
Generative AI will also streamline many aspects of cybersecurity. Spanswick said one of the biggest challenges in cybersecurity has been understanding where critical data sits, as well as getting insight into how that data moved through the company and how that data enables core business processes. Generative AI will help security teams obtain, analyze, synthesize and act on this data. This will make it easier to understand their attack surface and the level of protection required, as well as assess the security posture of these attack surfaces. This will help prioritize security investments, assess practical cyber-risk and set concrete KPIs for core security controls.
Business intelligence
Generative AI will play a pivotal role in improving the value and effectiveness of business intelligence (BI), predicted Porter Thorndike, principal product manager at software development firm Cloud Software Group. Analytics vendors are starting to explore how they can take advantage of AI capabilities in their platforms by either integrating with existing generative AI services or building their own. Generative AI will also streamline traditional BI workflows that now require close collaboration among developers, data scientists and business analysts. LLMs will help produce the same content, while requiring a less technical skill set. They will also help explain the meaning of content on existing dashboards tuned to different users.
Advancement of AIOps
Enterprises are increasingly turning to AI to improve IT operations management, or AIOps. This is sometimes confused with MLOps, which focuses on enhancing machine learning development workflows. Generative AI will improve the ability to sift through vast quantities of IT-related data to take programmatic actions, predicted Chris Opat, senior vice president of cloud operations at cloud backup and storage service Backblaze. He has started working with Selector AI to ingest various forms of business data to identify and mitigate anomalous behavior faster and more precisely.
Personalized customer experiences
Generative AI is also playing a role in increasing customer engagement through tailored interactions, website experiences, products and services. Kearney's Thota said generative AI could help businesses craft highly personalized content to boost sales through cross-selling and upselling opportunities. It can also reduce churn. He recommended CIOs work closely with marketing and customer relationship teams to gain insight into how generative AI can be integrated with existing customer engagement strategies. This can help guide the processing of collecting and integrating the data needed to build the personalization engine and have the structure to scale the tool as it expands across the organization.
Advanced conversational AI
Generative AI techniques will lead to more sophisticated NLP models to better understand context and generate humanlike text. Thota believes this could transform various aspects of business operations, including customer support, multilingual support, conversational knowledge databases and virtual assistants across multiple functions. He recommended companies prepare by identifying areas where advanced conversational AI can contribute to customer- and employee-facing interactions. They should also start thinking about governance and establishing user guidelines to prevent misuse of conversational AI models.
Automated content creation
Marketing, communication and design teams are using AI-powered tools to streamline content creation processes. This accelerates campaign timelines, optimizes creative resource allocation and bolsters brand consistency, said Dr. Stefan Sigg, chief product officer at Software AG. CIOs will need to explore ways to integrate AI-powered tools into workflows to improve collaboration between AI and humans. It's also important to upskill creative teams to work harmoniously with AI systems, scale AI infrastructure for increased content demands and foster an organizational shift that embraces AI as a creative ally rather than a replacement. "Perhaps, larger enterprises will end up having their own EnterpriseGPT to allow for customized use within the corporation," he said.
Increased efficiency of operations
The expected wide adoption of generative AI should improve the efficiency of operations in many different verticals, said Shipra Sharma, head of AI and analytics at AI consultancy Bristlecone. It can work alongside humans to make their jobs easier, which can translate to time and cost savings. "That's a tangible thing, and all organizations will eventually find a way to use generative AI to help make operations nimbler. But it's still questionable whether it can be used to increase profits or solve highly complex problems without human interaction," Sharma said.
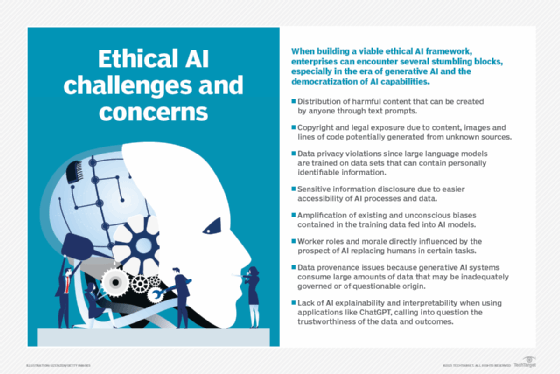
Ethical issues arising from generative AI
The rapid adoption of generative AI will also lead to various new problems and exacerbate existing ones. Important aspects to consider include the following:
- Primary risks. Enterprises must address primary risks around generative AI for broad-based adoption, Everest Group's Vijayan said. Top issues include regulatory, intellectual property, data, privacy and explainability concerns. He expects enterprises and vendors to develop meaningful workarounds and mitigation mechanisms, which seem promising based on current progress.
- Responsible use. PwC's Likens said enterprises must consider security and performance risks with generative AI, such as bias and hallucinations. This will make it imperative for enterprises to plan and execute responsible AI rollouts to employees. Enterprises will increasingly invest in internal AI tools to ensure proprietary data and ideas remain within the enterprise. Adopting responsible AI frameworks and guardrails could also promote responsible and ethical use.
- Shadow AI. Cloud innovations created a variety of low-cost and easy-to-access services that drove the growth of shadow IT outside the purview of IT governance. Abhishek Gupta, founder and principal researcher at Montreal AI Ethics Institute, predicted a similar rise in shadow AI. This refers to the AI systems, solutions and services used or developed within an organization without explicit organizational approval or oversight. It can include anything from using unsanctioned software and apps to developing AI-based solutions in a skunkworks-like fashion. Enterprises should embark on a drive to raise literacy around responsible use of AI systems and promote discussions of new risks, such as hallucinations, bias, and leakage of private and confidential information. "Ultimately, the goal is to make responsible AI the norm rather than the exception," Gupta said.
- Regulatory and copyright concerns. Regulators are still in the early stages of sorting out the impact of AI on existing copyright and intellectual property frameworks. "Content created by a generative AI model resembles human-created work and thus could lead to regulatory and copyright issues," Thota said. Enterprises need to develop plans for ethical usage of content and ensure models avoid plagiarism. CIOs must also be proactive and collaborate with legal teams to develop internal policies and guidelines for using generative AI applications within their firm. It's also important to track emerging trends in copyright laws and regulations within your industry.
- Trustworthy AI. Kumar R predicted generative AI technology providers and governing bodies will focus on making it more trustworthy. This will include more secure technology, data protection guarantees and legal protections. Data labeling and tagging, along with digital watermarks, could also enhance trust. These practices will also improve transparency into how the data is used and increase clarity on topics such as copyright.
- Rise of confidential computing. Training LLMs on vast data sets won't do for many enterprise data sets involving personally identifiable information or confidential data. Sigg expects security and privacy concerns to prompt the evolution of generative AI toward techniques like federated learning and secure multiparty computation that protect sensitive data during the training process. "These advancements allow businesses to collaboratively train models on decentralized data, fostering industry partnerships, while maintaining data security and complying with regulations such as GDPR," he said. This balance between data sharing and privacy protection opens doors to innovative collaborations previously hindered by privacy concerns.
- Interpretive AI. Early generative AI models made it difficult to discern how the technology was used across the organization at scale. AWS' Chadha predicted that innovations in interpretive AI will help CIOs understand the value of their customer base and organization regarding product impact, market adoption and internal utilization.
- Energy use. Generative AI can dramatically impact the consumption of IT resources and, hence, the energy usage of an organization. Chadha said organizations should prioritize use cases that are most valuable to their customers and strip down inference code to optimize cost and time. It might also be helpful to identify ways to make the GPUs at the heart of generative AI training and inferencing tasks more efficient by developing a strategy to consider additional GPU capacity coming online. CIOs might also position their company to run workloads on premises and monitor their cloud provider's effect on sustainability.