Choosing between a rule-based vs. machine learning system
Deciding between a rule-based vs. machine learning system comes down to complexity and organizational needs. Compare the advantages, drawbacks and use cases for each AI approach.
When implementing AI systems, choosing between a rule-based vs. machine learning architecture is critical to an application's usability, compatibility and efficiency.
Getting output from a rule-based AI system can be simple and nearly immediate, but machine learning systems can handle more complex tasks with greater adaptability. Enterprises should understand the core differences between rule-based and machine learning systems, including their benefits and limitations, before taking advantage of either.
What is a rule-based system?
Rule-based systems are a basic type of AI model that uses a set of prewritten rules to make decisions and solve problems. Developers create rules based on human expert knowledge, which then enable the system to process input data and produce a result.
To build a rule-based system, a developer first creates a list of rules and facts for the system. An inference engine then measures the information given against these rules. Here, human knowledge is encoded as rules in the form of if-then statements. The system follows the rules set and only performs the programmed functions.
This article is part of
What is machine learning? Guide, definition and examples
For example, a rule-based algorithm or platform could measure a bank customer's personal and financial information against a programmed set of levels. If the numbers match, the bank grants the applicant a home loan.
Advantages and downsides of rule-based systems
Organizations often use rule-based systems because of the following benefits:
- Accuracy. Rule-based systems operate by cause and effect, and only within their rule set. Thus, the system's rules act as guardrails to ensure precision and accuracy.
- Ease of use. Rule-based systems require only small amounts of simple data to perform tasks and repetitive processes. This makes them easy for developers to create, use and debug.
- Speed. With the proper training, rule-based systems can make informed decisions quickly and efficiently, as there is no room for interpretation or growth. Their limited parameters ensure that responses come as fast as possible.
But because of their simplistic nature, rule-based systems often fall short in the following ways:
- Limited scope. Rule-based systems are exact and do not have learning capabilities, which limits them to working only within the confines of their original programming. Including too many rules can slow down a system and introduce complexity.
- Immutability. By nature, rule-based systems do not change and are unscalable. Altering existing rules or incorporating new ones can introduce time-consuming and expensive complications.
- Restricted intelligence. A rule-based system is only as good as the rules set by its developers and cannot make decisions independent of those rules. This means the system can only act based on its explicit programming and will reflect any flaws or oversights in the original rule set.
What is a machine learning system?
Machine learning is a type of AI that enables applications to predict outcomes without being explicitly programmed to do so. Machine learning algorithms independently detect and analyze data patterns and modify their behavior accordingly to produce new output.
To create a machine learning system, developers select an appropriate AI model and present it with a large data set. The chosen algorithm analyzes the data set and determines relationships within that data.
The logic within machine learning systems is embedded in the algorithm, not coded by a human. As the name suggests, a machine learning model autonomously "learns" from its training data, creating a direct relationship between data inferences and future data outputs.
Advantages and downsides of machine learning systems
Machine learning systems provide the following benefits to organizations:
- Adaptability. Machine learning systems adjust without human intervention as new data is presented to make informed decisions and predictions through continuous learning. This makes them well suited to addressing dynamic problems in fast-changing environments.
- Self-learning. Machine learning systems adapt in response to the patterns and relationships in their input data, enabling models to define their own rules that take into account a wide range of variables. This capability makes machine learning a good choice for tackling complex processes that include multiple factors, rules and patterns that change over time.
- Scalability. As projects and environments grow, machine learning systems can scale and adapt accordingly with new data, infrastructure resources and algorithms. For example, an organization could upgrade a simple classifier to a neural network by expanding its training data set and underlying infrastructure.
Though machine learning systems can tackle many issues, they also come with the following downsides:
- Dependence on quality of training data. Because data quality directly affects model quality, machine learning systems must be thoroughly trained on historical and relevant data to make well-informed decisions and predictions. However, building a sufficiently large and relevant data set can be a resource-intensive and time-consuming process.
- Complexity. Although machine learning systems can handle larger, more complex tasks and environments than rule-based systems, they also require greater technical expertise from teams to develop effective applications. Tasks such as writing machine learning algorithms, identifying useful data and monitoring models in production warrant a data science team and require properly trained employees.
- Limited adaptability. Machine learning systems can adjust and grow, but they cannot adapt to situations or problems outside the realm they are trained for. These systems lack the human intuition to come to conclusions outside their range of knowledge.
Choosing between rule-based vs. machine learning systems
Rule-based AI and machine learning differ in both the type of logic they use to operate and the level of human intervention they require. For rule-based systems, the system's decision logic is instilled at the beginning, with little flexibility after deployment. Machine learning systems, on the other hand, can learn continuously without human direction.
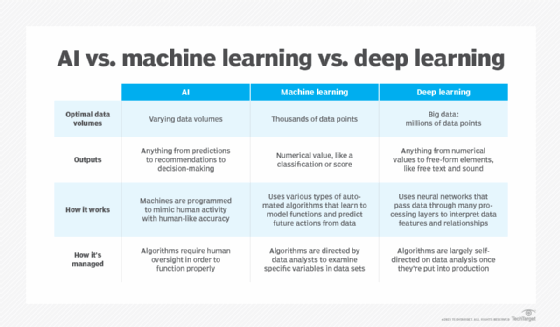
Given these differences in learning methods, the question of whether to use a rule-based or machine learning system comes down to organizational needs. Different types of AI can take different types of actions and produce different analyses or insights.
Applying machine learning and rule-based systems
The choice between a rule-based vs. machine learning system depends on how strict parameters must be, requirements around efficiency and training costs, and whether a data science team or an algorithm will create the rules.
Machine learning can handle complex and intensive issues in relatively variable environments, whereas rule-based AI systems are easier to interpret and promote precision. In addition, the adaptability and speed of machine learning systems comes at a cost. There is an advanced level of commitment with machine learning, as algorithms require large amounts of data to be effective.
Training on this data can take extended periods of time, so taking a rule-based approach might make more sense for simpler tasks. Rule-based systems work well for scenarios that don't involve highly complicated decisions or massive amounts of complex data.
The bottom line: When machine learning systems are the best option
Machine learning systems are often viewed as unequivocally better than rule-based AI, as they are capable of more complex analysis and can continuously learn from and adapt to changing data. Machine learning capabilities such as predictive analytics, anomaly detection and computer vision can achieve more comprehensive and nuanced results compared with rule-based systems. Consequently, if a business can support the cost and time required to build a machine learning model, doing so will often be the better choice.
However, rule-based systems still have a time and place. For one, they can be helpful for more rudimentary use cases, and they are often more cost-effective compared with machine learning. They also have a much narrower window for error than machine learning systems. Rule-based systems can thus prove more successful in scenarios with clear-cut rules where accuracy and speed are paramount -- for instance, simple medical diagnostics or online grading systems.
Use cases and limitations to consider
Though rule-based systems are typically not complex by nature, adding more rules and mitigating factors can introduce contradictions and overlap. While machine learning systems can account for greater complexity, it can come at the cost of accuracy. Consider, for example, the following real-world use cases and the variety of limitations they can present depending on what type of system is used.
Home loan approval
In the example of average income needed to be approved for a home loan, a rule-based system would have data scientists create a rule, such as requiring a monthly income of three times the estimated monthly mortgage payment. However, it's never that simple in large AI applications: The more modifications necessary, the more complicated rule-based systems can become.
These modifications could include anything from factoring in an applicant's location to accounting for an economic downturn. These new rules significantly increase the amount of time and planning originally anticipated. Although this increase in foundational work enables greater adaptability, it also takes more time. Therefore, mitigating the limiting factors can also decrease ease of use.
Turning to machine learning models makes sense when the modifications in a rule-based system become burdensome. Machine learning excels in constantly changing environments, as it is scalable and mutable.
Creating an algorithm, training it on relevant data sets, and teaching it to identify patterns and relationships requires a greater initial investment. But the resulting model can handle more complexity than a rule-based system.
In a home loan approval system, a machine learning system could use backpropagation for optimization to find the rule for approval as it learns from data. Thus, the model could ultimately arrive at a more nuanced rule that accounts for an applicant's income, credit history and location.
E-commerce chatbots
In the case of e-commerce chatbots, a rule-based system could be sufficient for rudimentary chatbot services. For example, consider a small business that wants to employ a chatbot that can provide preset answers regarding services, hours and products. A rule-based system can be programmed with predetermined answers for customers when specific words are used. Developing such a system would be more cost-effective and less time-consuming than programming a machine learning system.
However, customer questions are often complex, and the rules can change based on external circumstances. For more dynamic chatbots, using a machine learning system could create more complex question-answering, overall enhancing the user experience. However, businesses might need to devote more time and funds to the machine learning system and monitor it for issues of inaccuracy or bias.
Customer personalization and recommendation engines
Many organizations opt to integrate customer personalization into their services. For instance, a company can employ a machine learning system to analyze historic data to personalize a customer's experience in the form of purchase suggestions. Machine learning systems can also fuel recommendation engines. Netflix is a prime example -- its fine-tuned analysis of what users watch on its streaming platform enables the service to create curated recommendations for users.
In contrast, rule-based systems cannot learn from users' actions; instead, rule-based systems only provide recommendations and personalization through narrow if-then programming. For instance, if a viewer on a streaming platform watches the first movie in a trilogy, a rule-based system could be programmed to recommend the second movie in the series to the user upon finishing the first movie.
Banking and fraud protection
If an AI model is attempting to discover new or rare events -- as is the case in fraud protection -- the field from which examples can be harvested will be limited to start. Not having enough labeled data will hinder the model's performance, making banking and fraud protection use cases often best suited for a machine learning system.
For instance, a machine learning system can evaluate a credit card user's past purchasing tendencies to detect potential fraud. A rule-based system, on the other hand, would struggle to identify nuance in purchasing habits. It might automatically suspend a credit card if certain rules are met, such as when an international purchase is made. Instead, a machine learning system would be able to identify that a given U.S. customer often crosses the Canadian border and thus would not flag purchases made in Canada as suspicious behavior.
Editor's note: Joseph M. Carew originally wrote this tip, and Emily Foster and Olivia Wisbey later updated and expanded it.
Olivia Wisbey is associate site editor for TechTarget Enterprise AI. She graduated with Bachelor of Arts degrees in English literature and political science from Colgate University, where she served as a peer writing consultant at the university's Writing and Speaking Center.
Joseph M. Carew was formerly an assistant site editor at TechTarget, and Emily Foster was formerly an associate site editor at TechTarget.