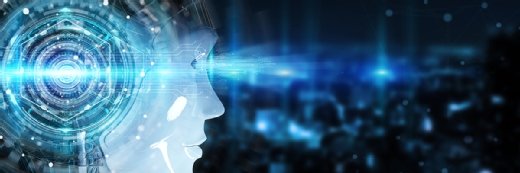
sdecoret - stock.adobe.com
Shadow AI poses new generation of threats to enterprise IT
AI is all the rage -- and so is shadow AI. Learn how unsanctioned use of generative AI tools can open organizations up to significant risks and what to do about it.
Large language model AI is the new black, at least for now.
But, even if the IT industry has hit the peak of AI hype -- and it is not clear that it yet has -- the world is still a long way from understanding how to use this newly powerful AI category responsibly, safely, effectively and efficiently. And unsanctioned AI, also known as shadow AI, poses even more challenges.
What is shadow AI?
Shadow AI is just like every other stripe of shadow IT -- unsanctioned technology that corporate employees deploy ad hoc and use in ways unknown to or hidden from an organization's central IT and risk management functions.
Users turn to shadow IT for any number of reasons, such as the following:
- They have use cases that existing, sanctioned apps fail to address.
- They consider the central IT department unresponsive or incapable of handling an emerging technology.
- Their organization has budgetary restraints that preclude it from adopting sanctioned, enterprise-grade versions of emerging technologies.
- They deem technological and tooling constraints on an approved project too restrictive.
Shadow AI risks
Many corporate users are undoubtedly experimenting with generative AI (GenAI) apps, such as ChatGPT and Google Bard, to see how these tools might help them do their jobs more efficiently and effectively. The impulse is understandable, but shadow AI -- as with any sanctioned large language model (LLM) AI project -- presents specific cybersecurity and business risks, including the following.
Functional risks
Functional risks stem from an AI tool's ability to function properly. For example, model drift is a functional risk. It occurs when the AI model falls out of alignment with the problem space it was trained to address, rendering it useless and potentially misleading. Model drift might happen because of changes in the technical environment or outdated training data.
Operational risks
Operational risks endanger the company's ability to do business. Operational risks come in many forms. For example, a shadow AI tool could give bad advice to the business because it is suffering from model drift, was inadequately trained or is hallucinating -- i.e., generating false information. Following bad advice from GenAI can result in wasted investments -- for example, if the business expands unwisely -- and higher opportunity costs -- for example, if it fails to invest where it should.
Use of an LLM -- especially an unsanctioned one that was developed and trained outside the enterprise's data management policy framework -- could also expose sensitive company and customer data. For example, a chatbot might ingest information included in a user's prompt; use it as training data; make it available to platform operators; and make it available to other users when answering their prompts. If the AI platform were to suffer a cyberattack, the data could also fall into cybercriminals' hands.
Imagine the following problematic shadow AI scenarios:
- A healthcare provider uses a GenAI chatbot to summarize a patient's appointment, exposing medical information and violating HIPAA.
- An executive uses AI to create talking points for an internal presentation, exposing confidential business strategies.
- A lawyer uses a chatbot to organize case notes, exposing details subject to attorney-client privilege.
- A business analyst uploads a large, multifield spreadsheet to a GenAI app to generate a new report, exposing customer credit card numbers.
Sharing this kind of sensitive information with an LLM could put the organization's intellectual property and business strategies at risk, empowering competitors and eroding competitive advantages. In the case of personally identifiable information, it could result in serious data privacy and compliance violations, damage to customer trust and reputational fallout.
Legal risks
Legal risks follow functional and operational risks if shadow AI exposes the company to lawsuits or fines. Say the model advises leadership on business strategy. But the information is incorrect, and the company wastes a huge amount of money doing the wrong thing. Shareholders might sue.
Lawsuits might also materialize if the shadow tool provides customers with bad advice generated by model drift or poisoned training data or if the model uses copyright-protected data for self-training.
And, of course, violations of data privacy regulations could result in hefty legal penalties.
As the legal strictures around AI development continue to evolve, regulatory fines and criminal sanctions may become of growing concern.
Resource risks
Finally, shadow AI usage opens the door to wasteful or duplicative spending among shadow projects or between shadow and sanctioned ones. In some cases, shadow AI users may also waste money by failing to take advantage of negotiated rates for similar, sanctioned technology.
Consider, too, the opportunity cost stemming from shadow projects that ultimately fail because they do not follow company policies or good practices -- that time and money could have been put toward other projects.
And, for shadow projects that do get brought into the portfolio and cease to be shadow, expect transition costs. Staff and employees who used the shadow tool will likely have to be retrained to understand the tool set in its new context with new parameters. And the organization will also face migration costs associated with shifting other users to the sanctioned tool.
How to manage the risks of shadow AI
IT and security teams have few methods at their disposal to preemptively find and rein in shadow AI, even when they have authority to do so.
The resolution lies at the leadership level, with the CIO and CISO working with the CEO, CFO and head of risk management. The CEO has to lend the highest level of support to the process; the CFO needs to sniff out spending on AI applications, platforms and tools that is not visible to IT.
The goal isn't to enlist IT and security teams in crackdowns on the unsanctioned use of AI or even necessarily to force shadow AI users onto preferred technical platforms. Instead, the focus has to be on visibility, risk management and strategic decision-making.
Firstly, leadership needs to know how much is being spent on AI -- sanctioned and otherwise.
Secondly, groups previously working outside the ambit of institutional risk controls must be brought into the fold. Their projects have to comply with the enterprise's risk management requirements, if not its technical choices.
The following steps can further help organizations manage shadow AI risks:
- Classify data. Data classification is a cornerstone of data security and good information stewardship generally, and its importance extends to secure GenAI use. For example, an organization might choose to allow the use of consumer-grade chatbots but only for projects involving publicly available information. Sensitive data, on the other hand, might be restricted to on-premises AI deployments or secure, enterprise-grade apps that are trained to abide by internal data security policies.
- Create an AI acceptable use policy. An AI acceptable use policy can clearly communicate that improper AI usage can hurt the organization, as well as how to align AI usage with data security policies and other risk mitigation strategies. Then, if and when shadow AI surfaces, decision-makers can compare the tools' use against the policy to quickly identify risk exposure and necessary next steps.
- Educate and train employees. AI policies are useless if employees aren't aware of them or don't understand them. With this in mind, prioritize educating employees on safe and secure GenAI usage, either as a part of ongoing cybersecurity awareness training or as a standalone initiative. Training should also communicate the risks of AI use, with particular emphasis on data protection and compliance requirements.
The future of shadow AI
Security and risk leaders should not expect shadow AI to go away any time soon -- especially given the still-expanding set of options available for SaaS tools and for on-premises development. As new-generation LLMs become more numerous and diverse -- both in costs and resource requirements -- there is every reason to expect shadow AI projects will multiply as well.
John Burke is CTO and principal research analyst with Nemertes Research. With nearly two decades of technology experience, he has worked at all levels of IT, including end-user support specialist, programmer, system administrator, database specialist, network administrator, network architect and systems architect. His focus areas include AI, cloud, networking, infrastructure, automation and cybersecurity.
Alissa Irei is senior site editor of TechTarget Security.