internet of things (IoT)
What is the internet of things (IoT)?
The internet of things, or IoT, is a network of interrelated devices that connect and exchange data with other IoT devices and the cloud. IoT devices are typically embedded with technology such as sensors and software and can include mechanical and digital machines and consumer objects.
These devices encompass everything from everyday household items to complex industrial tools. Increasingly, organizations in a variety of industries are using IoT to operate more efficiently, deliver enhanced customer service, improve decision-making and increase the value of the business.
With IoT, data is transferable over a network without requiring human-to-human or human-to-computer interactions.
A thing in the internet of things can be a person with a heart monitor implant, a farm animal with a biochip transponder, an automobile that has built-in sensors to alert the driver when tire pressure is low, or any other natural or man-made object that can be assigned an Internet Protocol address and can transfer data over a network.
How does IoT work?
IoT systems function by gathering data from sensors embedded in IoT devices, which is then transmitted through an IoT gateway for analysis by an application or back-end system.
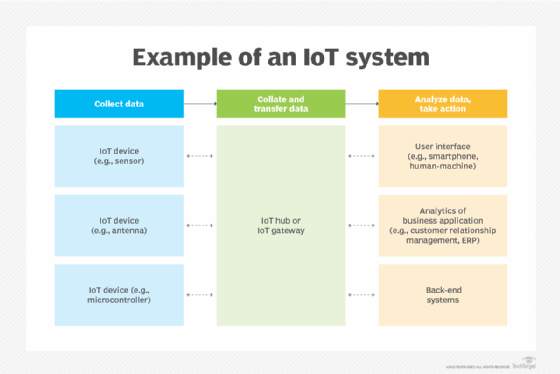
The following four elements are incorporated into an IoT ecosystem for it to function:
Sensors or devices
An IoT ecosystem consists of web-enabled smart devices that use embedded systems, such as processors, sensors and communication hardware to collect, send and act on data acquired from their environments.
Connectivity
IoT devices can communicate with one another through a network over the internet. These devices share sensor data by connecting to an IoT gateway, which acts as a central hub where IoT devices can send data. Before the data is shared, it can also be sent to an edge device where it is analyzed locally.
Data analysis
Only the relevant data is used to identify patterns, offer recommendations and identify potential issues before they escalate. Analyzing data locally reduces the volume of data sent to the cloud, which minimizes bandwidth consumption.
Sometimes, these devices communicate with other related devices and act on the information they get from one another. The devices do most of the work without human intervention, although people can interact with the devices. For example, they can set them up, give them instructions or access the data. The connectivity, networking and communication protocols used with these web-enabled devices largely depend on the specific IoT applications deployed.
IoT can also use artificial intelligence and machine learning to make data collection processes easier and more dynamic.
Graphical user interface
A graphical user interface (UI) is typically used to manage IoT devices. For example, a website or a mobile app can be used as an UI to manage, control and register smart devices.
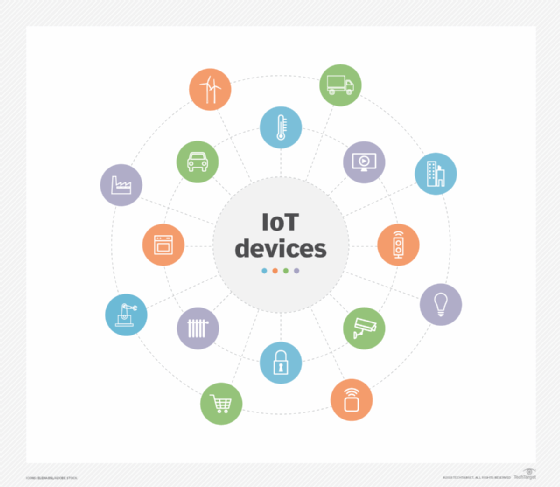
Why is IoT important?
IoT helps people live and work smarter. Consumers, for example, can use IoT-embedded devices -- such as cars, smartwatches or thermostats -- to improve their lives. For example, when a person arrives home, their car could communicate with the garage to open the door; their thermostat could adjust to a preset temperature; and their lighting could be set to a lower intensity and color.
In addition to offering smart devices to automate homes, IoT is essential to business. It provides organizations with a real-time look into how their systems work, delivering insights into everything from the performance of machines to supply chain and logistics operations.
IoT enables machines to complete tedious tasks without human intervention. Companies can automate processes, reduce labor costs, cut down on waste and improve service delivery. IoT helps make it less expensive to manufacture and deliver goods and offers transparency into customer transactions.
IoT continues to advance as more businesses realize the potential of connected devices to keep them competitive.
What are the benefits of IoT to organizations
IoT offers several benefits to organizations. It encourages companies to rethink how they approach their businesses and gives them the tools to improve their business strategies.
Some benefits of IoT are industry-specific while others are applicable across multiple industries. Generally, industrial internet of things (IIoT) is most abundant in manufacturing, transportation and utility organizations that use sensors and other IoT devices; however, it also has use cases for organizations within the agriculture, infrastructure and home automation industries, leading some organizations toward digital transformation.
Examples of consumer and enterprise IoT applications
Common example of IoT applications include the following:
- Agriculture. IoT can benefit farmers by making their jobs easier. For example, sensors can collect data on rainfall, humidity, temperature and soil content and IoT can help automate farming techniques. Additionally, IoT devices can be used to oversee the health of livestock, monitor equipment and streamline supply chain management.
- Construction. IoT can help monitor operations surrounding infrastructure. Sensors, for example, can monitor events or changes within structural buildings, bridges and other infrastructure that could potentially compromise safety. This provides benefits such as improved incident management and response, reduced operations costs and improved service quality.
- Home automation. A home automation business can use IoT to monitor and manipulate mechanical and electrical systems in a building. Homeowners can also remotely control and automate their home environment by using IoT devices, including smart thermostats, lighting systems, security cameras and voice assistants such as Alexa and Siri for increased comfort and energy efficiency.
- Smart buildings and cities. Smart cities can help citizens reduce waste and energy consumption. They can reduce energy costs using sensors that detect how many occupants are in a room and turning the air conditioner on if sensors detect a conference room is full or lowering the heat if everyone in the office has gone home.
- Urban consumption systems. IoT technologies can also be used to monitor and manage urban consumption such as traffic lights, parking meters, waste management systems and public transportation networks.
- Healthcare monitoring. IoT devices such as remote patient monitoring systems, smart medical devices and medication trackers let healthcare providers monitor patients' health status, manage chronic conditions and provide timely interventions. IoT gives providers the ability to monitor patients more closely by analyzing the generated data. Hospitals also often use IoT systems to complete tasks such as inventory management for both pharmaceuticals and medical instruments.
- Retail. IoT sensors and beacons in retail stores can track customer movement, analyze shopping patterns, manage inventory levels and personalize marketing messages. This enhances the shopping experience for customers and optimizes store operations.
- Transportation. IoT devices help the transportation industry by monitoring vehicle performance, optimizing routes and tracking shipments. For example, the fuel efficiency of connected cars can be monitored to reduce fuel costs and improve sustainability. IoT devices can also monitor the condition of cargo so it reaches its destination in optimal condition.
- Wearable devices. Wearable devices with sensors and software can collect and analyze user data, sending messages to other technologies about the users to make their lives easier and more comfortable. Wearable devices are also used for public safety -- for example, by improving first responders' response times during emergencies by providing optimized routes to a location or by tracking construction workers' or firefighters' vital signs at life-threatening sites.
- Energy management. IoT-enabled smart grids, smart meters and energy management systems let utility companies and consumers monitor and optimize energy usage, manage demand-response programs and integrate renewable energy sources more efficiently. For example, the data collected by the IoT devices and sensors helps identify patterns, peak usage times and areas of inefficiency.
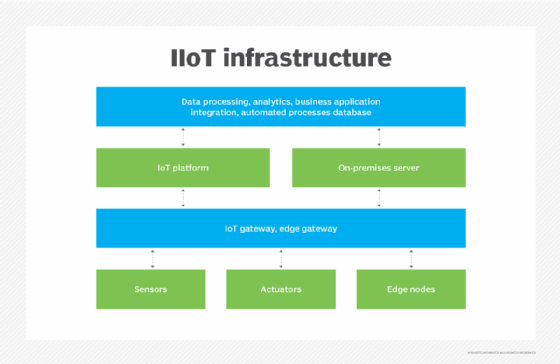
What are the pros and cons of IoT?
Some of the advantages of IoT devices include the following:
- Easy accessibility. IoT provides easy access to information from anywhere at any time on any device. For example, IoT enhances the accessibility of information by providing real-time data and insights, intuitive interfaces and proactive alerts.
- Improves communication. IoT improves communication between connected electronic devices. It achieves this by enabling efficient data exchange, extending network reach, conserving energy and prioritizing critical communications. For example, if a motion sensor in a smart home ecosystem detects activity at the front door, it triggers a communication alert with the smart lighting system to turn on the outdoor lights.
- Saves time and money. IoT enables the transfer of data packets over a connected network, which can save time and money. Predictive maintenance in industrial settings is another good example of this. IoT sensors installed on machinery continuously monitor parameters such as temperature, vibration and operating conditions in real-time. Data gathered from these sensors is analyzed using machine learning algorithms to detect patterns that show potential flaws or degradation in performance which helps in saving both time and money.
- Optimizes supply chain. IoT data can be used to optimize supply chain and inventory management processes, enabling manufacturers to reduce costs and enhance customer satisfaction. By tracking goods and materials in real-time, manufacturers can keep track of low stock, reduce excess inventory and streamline logistics operations.
- Improves efficiency. IoT analyzes data at the edge, reducing the amount of data that needs to be sent to the cloud. Edge computing enables physical devices to communicate more efficiently by processing data locally and exchanging only relevant information with other devices or cloud services.
- Provides automation. IoT automates tasks to improve the quality of a business's services and reduces the need for human intervention. For example, in agriculture, IoT-enabled irrigation systems can automatically adjust watering schedules based on soil moisture levels, weather forecasts and crop requirements.
- Improves customer experience. IoT enables the development of personalized products and services tailored to individual preferences and needs. Smart home devices, wearable technology and personalized recommendations in retail are examples of how IoT enhances the customer experience.
- Provides flexibility. IoT options can be scaled according to changing needs of a business. Whether it's adding new devices, expanding operations or integrating with existing systems, IoT provides the flexibility to scale and evolve with business requirements.
- Enables better business decisions. IoT generates vast amounts of data that can be analyzed to gain valuable insights into operations, consumer behavior and market trends. By harnessing and analyzing big data, businesses can make data-driven decisions, optimize processes and identify new revenue opportunities.
- Offers environmental sustainability. IoT enables efficient use of resources and reduces the negative environmental effects through initiatives such as smart energy management, waste reduction and sustainable agriculture practices. By optimizing resource utilization and minimizing waste, IoT contributes to environmental sustainability.
Along with its various advantages, IoT comes with some potential drawbacks including the following:
- Security concerns. IoT increases the attack surface as the number of connected devices grows. As more information is shared between devices, the potential for a hacker to steal confidential information increases.
- Complex management. Device management becomes more challenging as the number of IoT devices increases. Organizations might eventually have to deal with a massive number of IoT devices, and collecting and managing the data from all those devices could be difficult.
- Corruption of connected devices. IoT has the potential to corrupt other devices connected to the internet if there's a bug in the system.
- Compatibility issues. IoT increases compatibility issues between devices, as there's no international standard of compatibility for IoT, which causes platform fragmentation. Platform fragmentation refers to the proliferation of diverse and incompatible IoT platforms, protocols and standards, which can hinder interoperability and integration between different devices and systems. For example, many IoT vendors develop proprietary platforms and protocols that are tailored to their specific products and ecosystems. This results in a lack of standardization and interoperability, as devices from different manufacturers use incompatible technologies.
- Job displacements. Due to decreased human intervention in various tasks, IoT can result in job displacement for low-skilled workers. For example, automated inventory tasks and the use of ATMs have reduced the need for manual labor, leading to job losses and job insecurity for those currently employed in such roles.
- Regulatory and legal hurdles. With the proliferation of IoT devices, legal hurdles are also increasing. Businesses must adhere to diverse data protection, privacy and cybersecurity regulations, which can differ from one country to another.
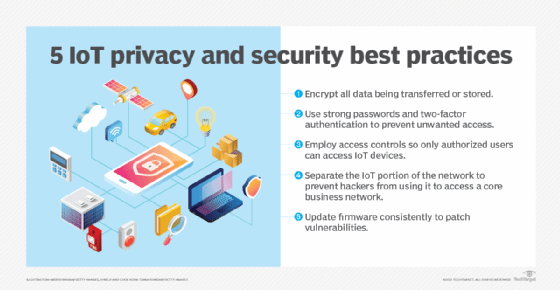
IoT standards and frameworks
Notable organizations involved in the development of IoT standards include the following:
- International Electrotechnical Commission.
- Institute of Electrical and Electronics Engineers (IEEE).
- Industrial Internet Consortium.
- Open Connectivity Foundation.
- Thread Group.
- Connectivity Standards Alliance.
Some examples of IoT standards include the following:
- IPv6 over Low-Power Wireless Personal Area Networks (6LoWPAN) is an open standard defined by the Internet Engineering Task Force (IETF). This standard lets any low-power radio communicate with the internet, including 804.15.4, Bluetooth Low Energy and Z-Wave for home automation. In addition to home automation, this standard is also used in industrial monitoring and agriculture.
- Zigbee is a low-power, low-data rate wireless network used mainly in home and industrial settings. ZigBee is based on the IEEE 802.15.4 standard. The ZigBee Alliance created Dotdot, the universal language for IoT that enables smart objects to work securely on any network and understand each other.
- Data Distribution Service (DDS) was developed by the Object Management Group and is an IIoT standard for real-time, scalable and high-performance machine-to-machine (M2M) communication.
IoT standards often use specific protocols for device communication. A chosen protocol dictates how IoT device data is transmitted and received. Some example IoT protocols include the following:
- Constrained Application Protocol. CoAP is a protocol designed by the IETF that specifies how low-power, compute-constrained devices can operate in IoT.
- Advanced Message Queuing Protocol. The AMQP is an open source published standard for asynchronous messaging by wire. AMQP enables encrypted and interoperable messaging between organizations and applications. The protocol is used in client-server messaging and in IoT device management.
- Long-Range Wide Area Network (LoRaWAN). This protocol for WANs is designed to support huge IoT networks, such as smart cities, with millions of low-power devices.
- MQ Telemetry Transport. MQTT is a lightweight protocol used for remote control and remote monitoring applications. It's suitable for devices with limited resources.
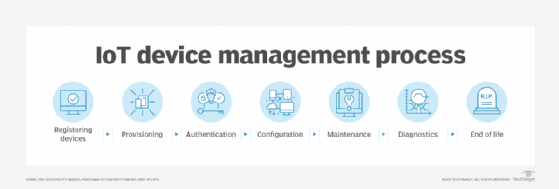
IoT frameworks include the following:
- Amazon Web Services (AWS) IoT is a cloud computing platform for IoT released by Amazon. This framework is designed to enable smart devices to easily connect and securely interact with the AWS cloud and other connected devices.
- Arm Mbed IoT is an open source platform to develop apps for IoT based on Arm microcontrollers. The goal of this IoT platform is to provide a scalable, connected and secure environment for IoT devices by integrating Mbed tools and services.
- Microsoft Azure IoT Suite platform is a set of services that let users interact with and receive data from their IoT devices, as well as perform various operations over data -- such as multidimensional analysis, transformation and aggregation -- and visualize those operations in a way that's suitable for business.
IoT security and privacy issues
IoT connects billions of devices to the internet and involves the use of billions of data points, all of which must be secured. Due to its expanded attack surface, IoT security and IoT privacy are cited as major concerns.
One of the most notorious IoT attacks happened in 2016. The Mirai botnet infiltrated domain name server provider Dyn, resulting in major system outages for an extended period of time. Attackers gained access to the network by exploiting poorly secured IoT devices. This is one of the largest distributed denial-of-service attacks ever seen and Mirai is still being developed today.
Because IoT devices are closely connected, a hacker can exploit one vulnerability to manipulate all the data, rendering it unusable. Manufacturers that don't update their devices regularly -- or at all -- leave them vulnerable to cybercriminals. Additionally, connected devices often ask users to input their personal information, including name, age, address, phone number and even social media accounts -- information that's invaluable to hackers.
Hackers aren't the only threat to IoT; privacy is another major concern. For example, companies that make and distribute consumer IoT devices could use those devices to obtain and sell user personal data. To ensure the safe and responsible use of IoT devices, organizations must provide education and awareness about security systems and best practices.
What technologies have made IoT possible?
Many technological advancements have accelerated IoT. A few key advancements include the following:
- Sensors and actuators. Environmental changes such as temperature, humidity, light, motion or pressure is detected by sensors, while actuators cause physical changes such as opening a valve or turning on a motor.
- Connectivity and network protocols. The availability of a host of network protocols for the internet has made it easy to connect sensors to the cloud and to other devices, facilitating efficient data transfer. IoT employs a range of connectivity technologies, including WiFi, Bluetooth, cellular, Zigbee and LoRaWAN.
- Low cost and low power sensor technology. More manufacturers now have access to IoT technology due to the availability of dependable and reasonably priced sensors. These sensors make it possible to gather data from the real world, which is then transferred to and analyzed in the digital domain.
- AI and NLP. Due to the developments in neural networks, IoT devices now feature natural language processing, which makes them appealing and useful for a wide range of uses, such as conversational AI assistants and digital personal assistants.
- Microservices and wireless technologies. IoT has evolved from the convergence of wireless technologies, microelectromechanical systems and microservices. All these advancements have facilitated seamless connectivity and data exchange between devices and the cloud.
What is the history and future outlook of IoT?
Kevin Ashton, co-founder of the Auto-ID Center at the Massachusetts Institute of Technology (MIT), first mentioned the internet of things in a presentation he made in 1999 to Procter & Gamble (P&G). Wanting to bring radio frequency ID to the attention of P&G's senior management, Ashton called his presentation "Internet of Things" to incorporate the cool new trend of 1999: the internet. MIT professor Neil Gershenfeld's book, When Things Start to Think, also appeared in 1999. Although the book didn't use the exact term, it provided a clear vision of where IoT was headed.
IoT has evolved from the convergence of wireless technologies, microelectromechanical systems, microservices and the internet. This convergence helped tear down the silos between operational technology and information technology, enabling unstructured machine-generated data to be analyzed for insights to drive improvements.
Although Ashton was the first to mention IoT, the idea of connected devices has been around since the 1970s, under the monikers embedded internet and pervasive computing.
The first internet appliance, for example, was a Coke machine at Carnegie Mellon University in the early 1980s. Using the web, programmers could check the status of the machine and determine whether there would be a cold drink awaiting them, should they decide to make the trip to the machine.
IoT evolved from M2M communication with machines connecting via a network without human interaction. M2M refers to connecting a device to the cloud, managing it and collecting data.
Taking M2M to the next level, IoT is a sensor network of billions of smart devices that connect people, computer systems and other applications to collect and share data. As its foundation, M2M offers the connectivity that enables IoT.
IoT is also a natural extension of supervisory control and data acquisition (SCADA), a category of software application programs for process control, the gathering of data in real time from remote locations to control equipment and conditions. SCADA systems include hardware and software components. The hardware gathers and feeds data into a desktop computer that has SCADA software installed, where it's then processed and presented in a timely manner. Late-generation SCADA systems developed into first-generation IoT systems.
The concept of the IoT ecosystem, however, didn't come into its own until 2010 when, in part, the government of China said it would make IoT a strategic priority in its five-year plan.
The following are some key milestones and current and future outlooks of IoT:
- Between 2010 and 2019, IoT evolved with broader consumer use. People increasingly used internet-connected devices, such as smartphones and smart TVs, which were all connected to one network and could communicate with each other.
- In 2020, the number of IoT devices continued to grow along with cellular IoT, which worked on 2G, 3G, 4G and 5G, as well as LoRaWAN and long-term evolution (LTE-M ) for machines.
- In 2023, billions of internet-connected devices collected and shared data for consumer and industry use. IoT has been an important aspect in the creation of digital twins -- which is a virtual representation of a real-world entity or process. The physical connections between the entity and its twin are most often IoT sensors, and a well-configured IoT implementation is often a prerequisite for digital twins.
- According to Forbes, in 2024, the IoT healthcare market is predicted to grow to around $150 billion with an expected valuation of $289 billion by 2028. Likewise, IoT in healthcare has expanded its use of wearables and in-home sensors that can remotely monitor a patient's health.
- By 2035, autonomous cars are expected to yield revenue between $300 billion and $400 billion. As IoT advances, there's a move from a single-device model to a modular, microservices approach. Connectivity technologies such as 5G, Wi-Fi 6, LPWAN and satellites are enhancing IoT adoption, while wearable devices such as smartwatches, earbuds and AR/VR headsets are increasingly evolving.
Learn about more current and potential future trends in IoT.