What is face detection and how does it work?
Face detection, also called facial detection, is a computer technology that identifies human faces in digital images and video. It clearly distinguishes those faces from other objects and establishes their boundaries. Face detection is used in a variety of fields, including security, biometrics, law enforcement, entertainment and social media. For example, face detection is often used for surveillance and tracking people in real time.
Face detection plays a vital role in face tracking, face analysis and facial recognition:
- Face tracking. A face tracking system extends face detection by making it possible to detect and track more detailed features in human faces that appear in images, videos or real-time camera feeds.
- Face analysis. Face analysis begins with face detection to locate faces in an image or video and then analyzes facial features to determine characteristics such as age, gender and emotions.
- Facial recognition. A facial recognition system relies on face detection to identify faces within an image or video so a faceprint can be created and compared to stored faceprints.
Today's face detection systems incorporate many advanced technologies, including artificial intelligence (AI) technologies, such as machine learning (ML), deep learning and artificial neural networks.
Face detection vs. face recognition
The terms face detection and face recognition are often used interchangeably, but they represent two different approaches to facial identification. Face detection is concerned primarily with detecting and locating faces. Facial recognition takes this a step further by identifying individuals based on their facial features.
Facial recognition software starts by using face detection to distinguish a face from other objects. Once a face is identified, the system generates a faceprint of that image, based on biometric indicators that provide a unique map of the face. The faceprint is then compared to a database containing faceprints of other individuals to see whether there is a match.
Facial recognition is one of the most significant applications of face detection. Facial recognition is used to unlock mobile devices and apps and to support other forms of biometric verification. The banking, retail and transportation industries use facial recognition routinely as part of their security strategies to discourage criminal behavior, protect sensitive resources and identify potential suspects if an incident occurs.
How face detection works
Face detection software typically uses AI and ML algorithms, along with statistical analysis and image processing, to find human faces within larger images and distinguish them from nonface objects, such as landscapes, buildings or other human body parts. Before face detection begins, the analyzed media might be preprocessed to improve its quality and remove objects that could interfere with detection.
Face detection algorithms usually start by searching for human eyes, one of the easiest features to detect. They then try to detect other facial landmarks, such as eyebrows, mouth, nose, nostrils and irises. Once the algorithm concludes that it has found a facial region, it performs additional tests to confirm that it has detected a face.
To ensure a high degree of accuracy, the algorithms are trained on large data sets that incorporate hundreds of thousands of positive and negative images. The training improves the algorithms' ability to determine whether there are faces in an image and exactly where their boundaries are.
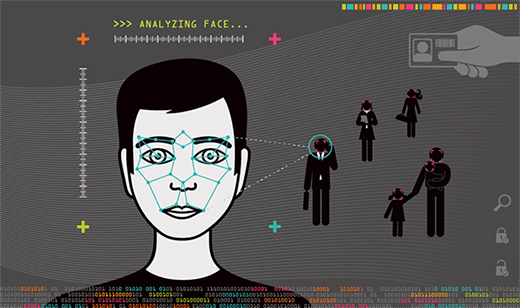
Common approaches to face detection
Face detection software uses different methods to detect faces in an image. The following approaches are some of the more common ones used for face detection:
- Knowledge- or rule-based. These approaches describe a face based on a set of rules, although creating well-defined, knowledge-based rules can be a challenge.
- Feature-based or feature-invariant. These methods use features such as a person's eyes or nose to detect a face, but the process can be negatively affected by noise and light.
- Template matching. This method is based on comparing images with previously stored standard face patterns or features and correlating the two to detect a face. However, this approach struggles to address variations in pose, scale and shape.
- Appearance-based. This method uses statistical analysis and ML to find the relevant characteristics of face images. The appearance-based method can struggle with changes in lighting and orientation.
Face detection also uses other techniques to identify faces in images or videos. For example, it might use one of more of the following approaches to help with the process:
- Background removal. If an image has a plain, monochrome background or a predefined, static one, removing the background might reveal the face boundaries.
- Skin color. In color images, skin color can sometimes be used to find faces, although this approach might not work with all complexions.
- Motion. Using motion to find faces is another option. In real-time video streaming, a face is almost always moving, so users of this method must calculate the moving area. One drawback of this approach is the risk of confusion with other objects moving in the background.
A combination of these strategies can help provide a more comprehensive face detection solution.
Face detection technologies
Face detection software has evolved over time, incorporating advanced technologies to achieve more accurate results and better performance.
One of the earliest efforts was based on the Viola-Jones algorithm, which trains a model to understand what is and isn't a face. Although the framework is still popular for recognizing faces in real-time applications, it has problems identifying faces that are covered or not properly oriented.
As AI technologies have improved, so too have face detection capabilities. Many of these systems now use machine learning and deep learning when searching for faces. They also incorporate convolutional neural networks (CNNs), a type of deep learning algorithm that analyzes visual data, using linear algebra to identify patterns and features within an image.
One CNN-based approach to face detection is region-based CNN (R-CNN). In this model, the CNN algorithm localizes and classifies objects in images and then generates proposals on a framework. The proposals focus on areas, or regions, in an image that are similar to other areas, such as the pixelated region of an eye. If the eye region matches other regions of the eye, the algorithm knows it has found a match. More recent approaches to facial recognition include Fast R-CNN and Faster R-CNN.
One of the challenges with R-CNN facial recognition and its derivatives is their tendency to become so complex that they overfit, which means they match regions of noise in the training data and not the intended patterns of facial features. Another issue with CNN-based approaches is that they tend to experience bottlenecks, in large part because the software makes two passes over the CNN: one for generating region proposals and one for detecting the object of each proposal.
The single-shot detector (SSD) method helps to address this issue by requiring only one pass over the network to detect objects within the image. As a result, the SSD method is faster than R-CNN. However, the SSD method has difficulty detecting small faces or faces farther away from the camera.
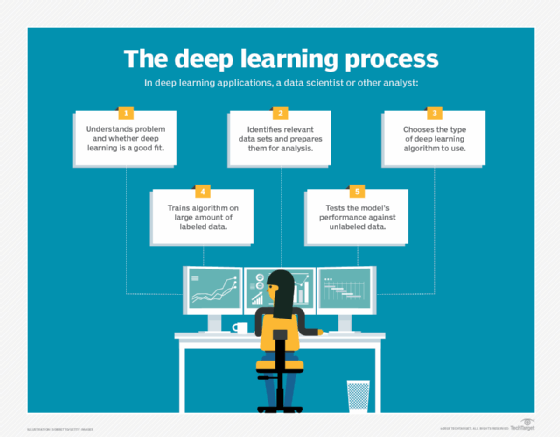
Uses of face detection
As already noted, face detection is a fundamental component of face recognition, which goes beyond simply finding and locating faces. Facial recognition generates faceprints that are compared to a faceprint database in hopes of determining who they are. However, face detection can also be used in a variety of other ways.
Entertainment
Face detection is used in movies, video games and virtual reality. For example, facial motion capture works in conjunction with face detection to electronically convert a human's facial movements into a digital database through the use of cameras and laser scanners. The database can then be used to produce realistic computer animation for movies, games or avatars.
Mobile devices
Smartphones and tablets often use face detection for their cameras' autofocus features when taking pictures and recording videos. Many mobile devices also use face detection in place of passcodes or passwords. For instance, an Apple iPhone can use face detection to unlock a phone for an approved user.
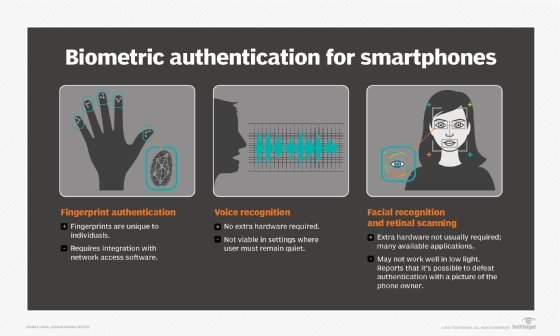
Security
Face detection is used in closed-circuit television security cameras to detect people who enter restricted spaces or to count how many people have entered an area. Face detection might also be used to draw language inferences from visual cues by performing a type of lip reading. Furthermore, it can help computers determine who is speaking and what they're saying, which can aid security applications. Face detection can also be used to determine which parts of an image to blur to ensure privacy.
Marketing
Face detection also has marketing applications. For example, it can be used to display specific advertisements when a particular face is recognized or to detect emotions when customers react to products or services.
Emotional inference
Another application for face detection is as part of a software implementation of emotional inference, which can help people with autism understand the feelings of people around them. The program reads the emotions on a human face using advanced image processing.
Biometric identification
Similar to how face detection is used with smartphones, it can be used in e-commerce and online banking to verify identities based on facial features. It can also be used to control access to physical facilities.
Social media
Social media apps use face detection to determine the identities of people in photos and to suggest how to tag them. Social media was one of the first mainstream uses of face detection.
Healthcare
Face recognition can facilitate patient check-ins and checkouts, maintain security, grant access control to restricted areas and evaluate a patient's emotional state. There is also a growing interest in using face detection to diagnose rare diseases.
Advantages of face detection
Face detection is a key element in facial imaging applications, such as facial recognition and face analysis. Face detection offers numerous advantages, including the following:
- Improved security. Face detection improves surveillance efforts and helps track down criminals and terrorists. Personal security is also enhanced when users can use their faces in place of passwords because there aren't any passwords or IDs for hackers to steal or change.
- Easy integration. Face detection and facial recognition technology are easy to integrate, and most of these applications are compatible with the majority of cybersecurity software.
- Automated identification. In the past, identification was performed manually by an individual, which was inefficient and frequently inaccurate. Face detection enables the identification process to be automated, saving time and increasing accuracy.
Disadvantages of face detection
Face detection also comes with a number of disadvantages, including the following:
- Massive data storage. The ML technology used in face detection requires a lot of data storage. Supporting that system can require significant overhead and resources.
- Inaccuracy. Face detection provides more accurate results than manual identification processes, but it can also be thrown off by changes in appearance, camera angles, expressions, positions, orientations, skin colors, pixel values, eye glasses, facial hair and differences in camera gain, lighting conditions and image resolution.
- Potential privacy breach. Face detection's ability to help the government track down criminals can offer many benefits, but the same surveillance enables governments to observe private citizens. Strict regulations must be put in place to ensure that the technology is used fairly and in compliance with human privacy rights.
- Discrimination. Experts have raised concerns about face detection's inaccuracy in recognizing people of color, particularly women, and how this can result in falsely connecting people of color with crimes they didn't commit. These worries are part of a broader concern about racial biases in ML algorithms.
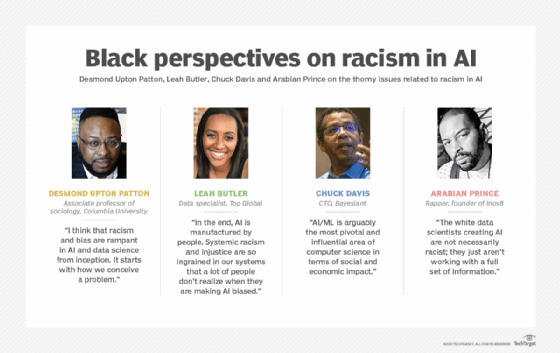
Popular face detection software
Many software products, online services and other tools incorporate face detection, including the following:
- Amazon Rekognition is a cloud-based service that provides customizable computer vision capabilities, including the ability to identify individuals in real-time video streams and pair individual metadata with faces.
- Dlib is a C++ toolkit that contains ML algorithms and other tools for creating complex software in a variety of domains, including security, surveillance and image analysis.
- Google Cloud Vision API provides APIs for accessing advanced vision models, including basic face detection and identification in photos and videos.
- Megvii AI algorithms use deep learning to analyze the visual elements in an image, including face detection in complex and varied environments.
- Microsoft Face API is a cloud-based service that uses algorithms to detect human faces in images to support services such as face detection, face verification and face grouping.
- OpenCV is an open source computer vision library used in academic and commercial applications to support real-time image processing, including object detection and face recognition.
History of face detection
The first computerized face detection experiments were launched in 1964 by American mathematician Woodrow W. Bledsoe. His team at Panoramic Research in Palo Alto, Calif., used a rudimentary scanner to scan people's faces and find matches in an attempt to program computers to recognize faces. The experiment was largely unsuccessful because of the computer's difficulty with poses, lighting and facial expressions.
Major improvements to face detection methodology came in 2001 when computer vision researchers Paul Viola and Michael Jones at Mitsubishi Electric Research Laboratories proposed a framework to detect faces in real time with high accuracy.
The Viola-Jones algorithm trains a model to understand what is and is not a face. Once trained, the model extracts specific features and stores them in a file. Those features can be compared with the stored features at various stages. If the image under study passes through each stage of the feature comparison, then a face has been detected, and operations can proceed.
The Viola-Jones framework is still used to recognize faces in real-time applications, but it has limitations. For example, the framework might not work if a face is covered with a mask or scarf. In addition, if the face isn't properly oriented, the algorithm might not be able to find it. Recent years have brought advances in face detection using deep learning, which outperforms traditional computer vision methods.
The future of face detection
Face detection capabilities are quickly growing due to the use of deep learning and neural networks. Advanced algorithms are driving face recognition systems to more accurate, real-time detections. They're also enabling pairings with other biometric authentications, such as fingerprints and voice recognition, helping to achieve more advanced security.
In some cases, developers and companies have taken a step back from face detection advancements, such as the ability to detect emotion through facial features. They're responding in part to larger concerns about the responsible and ethical use of AI. For instance, Microsoft removed emotional recognition capabilities from its Azure services.
Many experts cite ethical and privacy concerns in arguments against the further development of face detection and AI in general. Most significantly, face detection and facial recognition can be used without a person's consent or awareness. In addition, the risk of false positives continues to be a problem.
Even supporters of AI have urged the industry to slow down or take a temporary halt from developing AI systems, including face detection technology. They cite ethical considerations and concern about unforeseen negative consequences.
Even so, face detection capabilities continue to evolve and improve, including emotion detection. As AI technologies become more sophisticated and powerful, so do the face detection capabilities that rely on the AI technologies. In coming years, face detection will likely become even more widespread across domains and be incorporated into more platforms. It will also improve systems that already rely on face detection, particularly facial recognition.
Despite all this, there is a growing call for stricter controls over how and when face detection and other AI-based technologies should be used, although it's uncertain how successful these efforts will be.
Face detection is a technology at the cutting edge of AI. Learn the key benefits of AI for business.