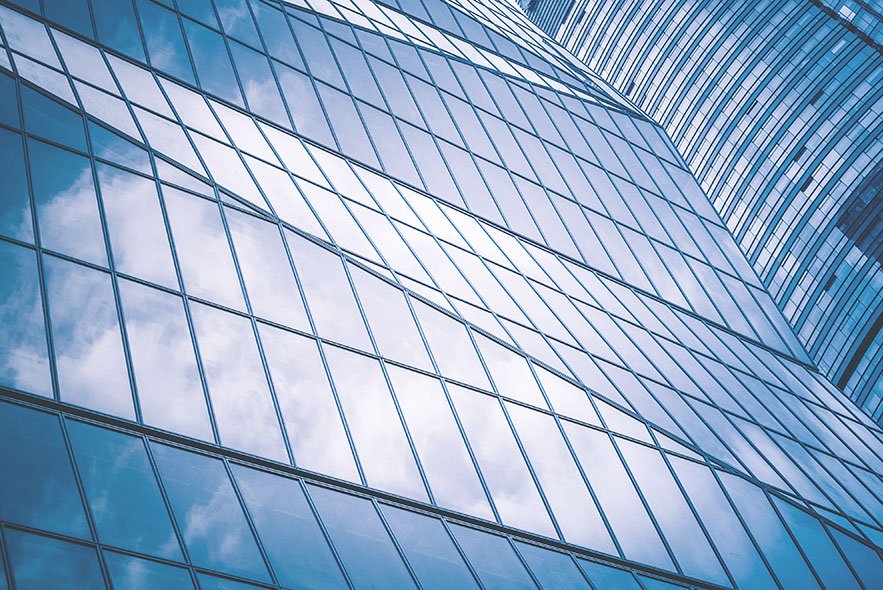
What is a convolutional neural network (CNN)?
A convolutional neural network (CNN) is a category of machine learning model. Specifically, it is a type of deep learning algorithm that is well suited to analyzing visual data. CNNs are commonly used to process image and video tasks. And, because CNNs are so effective at identifying objects, they are frequently used for computer vision tasks, such as image recognition and object recognition, with common use cases including self-driving cars, facial recognition and medical image analysis.
Older forms of neural networks often needed to process visual data in a gradual, piece-by-piece manner -- using segmented or lower-resolution input images. A CNN's comprehensive approach to image recognition enables it to outperform a traditional neural network on a range of image-related tasks and, to a lesser extent, speech and audio processing.
CNN architecture is inspired by the connectivity patterns of the human brain -- in particular, the visual cortex, which plays an essential role in perceiving and processing visual stimuli. The artificial neurons in a CNN are arranged to efficiently interpret visual information, enabling these models to process entire images.
CNNs also use principles from linear algebra, particularly convolution operations, to extract features and identify patterns within images. Although CNNs are predominantly used to process images, they can also be adapted to work with audio and other signal data.
This article is part of
What is enterprise AI? A complete guide for businesses
How do convolutional neural networks work?
CNNs have a series of layers, each of which detects different features of an input image. Depending on the complexity of its intended purpose, a CNN can contain dozens, hundreds and, on rarer occasions, even thousands of layers, each building on the outputs of previous layers to recognize detailed patterns.
The process starts by sliding a filter designed to detect certain features over the input image, a process known as convolution operation -- hence the name convolutional neural network. The result of this process is a feature map that highlights the presence of the detected features in the image. This feature map then serves as an input for the next layer, enabling a CNN to gradually build a hierarchical representation of the image.
Initial filters usually detect basic features, such as lines or simple textures. Subsequent layers' filters are more complex, combining the basic features identified earlier on to recognize more complex patterns. For example, after an initial layer detects the presence of edges, a deeper layer could use that information to start identifying shapes.
Between these layers, the network takes steps to reduce the spatial dimensions -- height and width -- of the feature maps to improve efficiency and accuracy. In the final layers of a CNN, the model makes a final decision -- for example, classifying an object in an image -- based on the output from the previous layers.
Unpacking the architecture of a CNN
A CNN typically consists of several layers, which can be broadly categorized into three groups: convolutional layers, pooling layers and fully connected layers. As data passes through these layers, the complexity of the CNN increases, which lets the CNN successively identify larger portions of an image, as well as more abstract features.
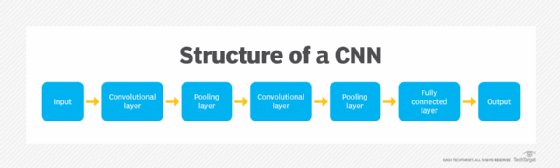
Convolutional layer
The convolutional layer is the fundamental portion of a CNN and is where the majority of computations happen. This layer uses a filter or kernel -- a small matrix of weights -- to move across the receptive field of an input image to detect the presence of specific features.
The process begins by sliding the kernel over the image's width and height, eventually sweeping across the entire image over multiple iterations. At each position, a dot product is calculated between the kernel's weights and the pixel values of the image under the kernel. This transforms the input image into a set of feature maps or convolved features, each of which represents the presence and intensity of a certain feature at various points in the image.
CNNs often include multiple stacked convolutional layers. Through this layered architecture, the CNN progressively interprets the visual information contained in the raw image data. In the earlier layers, the CNN identifies basic features, such as edges, textures or colors. Deeper layers receive input from the feature maps of previous layers, enabling them to detect more complex patterns, objects and scenes.
Pooling layer
The pooling layer of a CNN is a critical component that follows the convolutional layer. Similar to the convolutional layer, the pooling layer's operations involve a sweeping process across the input image, but its function is otherwise different.
The pooling layer aims to reduce the dimensionality of the input data, while retaining critical information, thus improving the network's overall efficiency. This is typically achieved through downsampling, which is the number of data points in the input.
For CNNs, this typically means reducing the number of pixels used to represent the image. The most common form of pooling is max pooling, which retains the maximum value within a certain window -- i.e., the kernel size -- while discarding other values. Another common technique, known as average pooling, takes a similar approach but uses the average value instead of the maximum.
Downsampling significantly reduces the overall number of parameters and computations. In addition to improving efficiency, this strengthens the model's generalization ability. Less complex models with higher-level features are typically less prone to overfitting -- an occurrence where a model learns noise and overly specific details in its training data, negatively affecting its ability to generalize to new, unseen information.
Reducing the spatial size of the representation does have a potential downside, namely the loss of some information. However, learning only the most prominent features of the input data is usually sufficient for tasks such as object detection and image classification.
Fully connected layer
The fully connected layer plays a critical role in the final stages of a CNN, where it is responsible for classifying images based on the features extracted in the previous layers. The term fully connected means that each neuron in one layer is connected to each neuron in the subsequent layer.
The fully connected layer integrates the various features extracted in the previous convolutional and pooling layers and maps them to specific classes or outcomes. Each input from the previous layer connects to each activation unit in the fully connected layer, enabling the CNN to simultaneously consider all features when making a final classification decision.
Not all layers in a CNN are fully connected. Because fully connected layers have many parameters, applying this approach throughout the entire network creates unnecessary density, increases the risk of overfitting and makes the network expensive to train in terms of memory and compute. Limiting the number of fully connected layers balances computational efficiency and generalization ability with the capability to learn complex patterns.
Additional layers
The convolutional, pooling and fully connected layers are all considered to be the core layers of a CNN. There are, however, additional layers that a CNN might have:
- The activation layer is a commonly added and equally important layer in a CNN. The activation layer enables nonlinearity -- meaning the network can learn more complex (nonlinear) patterns. This is crucial for solving complex tasks. This layer often comes after the convolutional or fully connected layers. Common activation functions include the ReLU, Sigmoid, Softmax and Tanh functions.
- The dropout layer is another added layer. The goal of the dropout layer is to reduce overfitting by dropping neurons from the neural network during training. This reduces the size of the model and helps prevent overfitting.
CNNs vs. traditional neural networks
A more traditional form of neural networks, known as multilayer perceptrons, consists entirely of fully connected layers. These neural networks, while versatile, are not optimized for spatial data, like images. This can create a number of problems when using them to handle larger, more complex input data.
For a smaller image with fewer color channels, a traditional neural network might produce satisfactory results. But, as image size and complexity increase, so does the amount of computational resources required. Another major issue is the tendency to overfit, as fully connected architectures do not automatically prioritize the most relevant features and are more likely to learn noise and other irrelevant information.
CNNs differ from traditional neural networks in a few key ways. Importantly, in a CNN, not every node in a layer is connected to each node in the next layer. Because their convolutional layers have fewer parameters compared with the fully connected layers of a traditional neural network, CNNs perform more efficiently on image processing tasks.
CNNs use a technique known as parameter sharing that makes them much more efficient at handling image data. In the convolutional layers, the same filter -- with fixed weights -- is used to scan the entire image, drastically reducing the number of parameters compared to a fully connected layer of a traditional neural network. The pooling layers further reduce the dimensionality of the data to improve a CNN's overall efficiency and generalizability.
CNNs vs. RNNs
Recurrent neural networks (RNNs) are a type of deep learning algorithm designed to process sequential or time-series data. They are able to recognize data's sequential characteristics and use patterns to predict the next likely scenario. RNNs are commonly used in speech recognition and natural language processing (NLP).
Both RNNs and CNNs are forms of deep learning algorithms. Both have also been important developments in the artificial intelligence (AI) field. And, although they have similar acronyms, they have distinct tasks they excel in. RNNs are well suited for use in NLP, sentiment analysis, language translation, speech recognition and image captioning, where the temporal sequence of data is particularly important. CNNs, in contrast, are primarily specialized for processing spatial data, such as images. They excel at image-related tasks, such as image recognition, object classification and pattern recognition.
They also have different architectures. CNNs use feedforward neural networks that use filters and a variety of layers, while RNNs feed results back into the network.
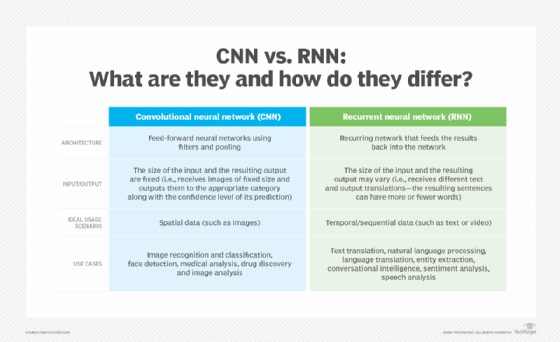
Benefits of using CNNs for deep learning
Deep learning, a subcategory of machine learning, uses multilayered neural networks that offer several benefits over simpler single-layer networks. CNNs, in particular, offer a variety of benefits as a deep learning process:
- Strong in computer vision tasks. CNNs are especially useful for computer vision tasks, such as image recognition and classification, because they are designed to learn the spatial hierarchies of features by capturing essential features in early layers and complex patterns in deeper layers.
- Strong in automatic processes. One of the most significant advantages of CNNs is their ability to perform automatic feature extraction or feature learning. This eliminates the need to extract features manually, historically a labor-intensive and complex process.
- Reusable. CNNs are also well suited for transfer learning, in which a pretrained model is fine-tuned for new tasks. This reusability makes CNNs versatile and efficient, particularly for tasks with limited training data. Building on preexisting networks enables machine learning developers to deploy CNNs in various real-world scenarios, while minimizing computational costs.
- Efficient. As described above, CNNs are more computationally efficient than traditional fully connected neural networks, thanks to their use of parameter sharing. Due to their streamlined architecture, CNNs can be deployed on a wide range of devices, including in mobile devices, such as smartphones, and in edge computing scenarios.
Disadvantages of using CNNs
Difficulties that come with CNNs, however, can include the following:
- Difficult to train. Training a CNN takes up a lot of computational resources and might require extensive tuning.
- Large amount of required training data. CNNs typically require a large amount of labeled data to train to an acceptable level of performance.
- Interpretability. It might become difficult to understand how a CNN arrives at a specific prediction or output.
- Overfitting. Without a dropout layer, a CNN might become prone to overfitting.
Applications of convolutional neural networks
Because processing and interpreting visual data are such common tasks, CNNs have a wide range of real-world applications, from healthcare and automotive to social media and retail.
The most common fields in which CNNs are used include the following:
- Healthcare. In the healthcare sector, CNNs are used to assist in medical diagnostics and imaging. For example, a CNN could analyze medical images, such as X-rays or pathology slides, to detect anomalies indicative of disease, thereby aiding in diagnosis and treatment planning.
- Automotive. The automotive industry uses CNNs in self-driving cars to navigate environments by interpreting camera and sensor data. CNNs are also useful in AI-powered features of nonautonomous vehicles, such as automated cruise control and parking assistance.
- Social media. On social media platforms, CNNs are employed in a range of image analysis tasks. For example, a social media company might use a CNN to suggest people to tag in photographs or to flag potentially offensive images for moderation.
- Retail. E-commerce retailers use CNNs in visual search systems that let users search for products using images rather than text. Online retailers can also use CNNs to improve their recommendation systems by identifying products that visually resemble those a shopper has shown interest in.
- Virtual assistants. Although CNNs are most often used to work with image data, virtual assistants are a good example of applying CNNs to audio processing problems. CNNs can recognize spoken keywords and help interpret users' commands, enhancing a virtual assistant's ability to understand and respond to its user.
CNNs have many varied cases where they can be useful. Learn more about how CNNs and other types of deep learning methods are used in the healthcare space.