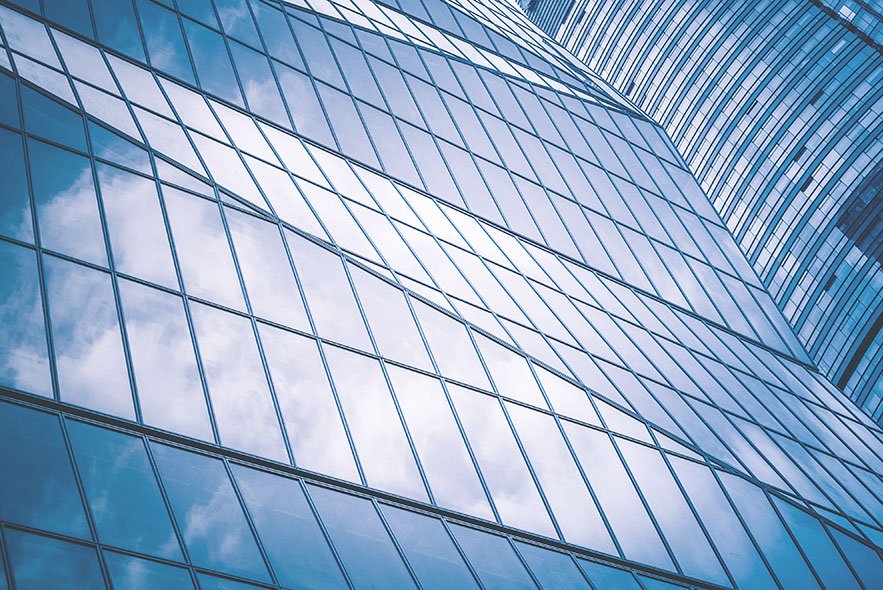
AI winter
What is AI winter?
AI winter is a quiet period for artificial intelligence (AI) research and development. Over the years, funding for AI initiatives has gone through a number of active and inactive cycles. The label winter is used to describe dormant periods when customer interest in AI declines. Use of the season winter to describe the resulting downturn emphasizes the idea that the quiet period will be a temporary state, followed again by growth and renewed interest.
History and timeline of AI winters
The trajectory of AI has been marked by several winters since its inception in 1955 in a formal proposal made by computer scientist and AI researcher Marvin Minksy and several others. Between 1956 and 1974, the U.S. Defense Advanced Research Projects Agency (DARPA) funded AI research with few requirements for developing functional projects. A large amount of hype was spurred during the mid-50s by the following collection of AI projects:
- a machine translation experiment that generated crude word-to-word Russian to English translation;
- a program that could play checkers; and
- a neural network that consisted of perceptrons, which were crude replications of the human brain's neurons.
After the initial hype generated by these AI projects, a quiet decade followed where interest and support gradually tapered off. In 1969, Minsky and another AI researcher, Seymour Papert, published a book called Perceptrons, which pointed out the flaws and limitations of neural networks. This publication influenced DARPA to withdraw its previous funding of AI projects.
In 1973, an evaluation of academic research in the field of AI called the "Lighthill Report" was published. It was highly critical of research in the field up to that point, stating that AI research had essentially failed to live up to the grandiose objectives it laid out. This report caused the U.K. to cease funding for AI. This ushered in the first AI winter, which took place between 1974-1980, after a nearly 20-year period of significant interest during what some have called AI's Golden Era. Interest in AI wouldn't be revived until years later with the advent of expert systems, which used if-then, rule-based reasoning. This would eventually end with another AI winter from the late 1980s to mid-90s.
This article is part of
What is enterprise AI? A complete guide for businesses
We're currently in one of the longest periods of sustained interest in AI in history. Today's distributed systems dwarf the computing power of the past and there are vast troves of training data on which AI systems can cut their teeth. These are distinct advantages that AI developers lacked in the past and are two of the primary drivers behind today's AI advances. But it's still an open question how far the technology can go. Many doubt AI's ability to pass the Turing Test and prove its ability to create systems that imitate human intelligence and behavior.
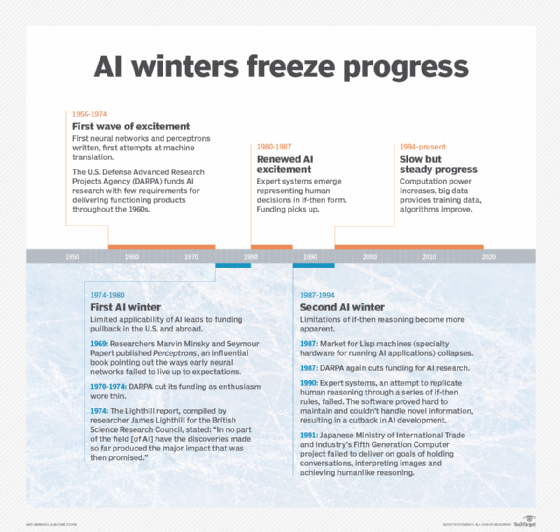
The main causes behind AI winters
Historically, AI winters have occurred because vendor promises have fallen short and AI initiatives have been more complicated to carry out than promised. When AI-washed products fail to deliver a significant return on investment (ROI), buyers become disappointed and direct their attention elsewhere.
AI winters occur when the hype behind AI research and development starts to stagnate. They also happen when the functions of AI stop being commercially viable. The promises generated by new techniques tend to create a large amount of buzz and raise the public's expectations. Businesses and organizations invest a lot of money based on these expectations, and gradually over time, if the new technology fails to deliver on those expectations, they lose interest in AI. If organizations begin to withdraw funding, it's a sign of waning interest and an impending AI winter.
To forestall another AI winter, some vendors have chosen to label software features predictive instead of artificially intelligent.
Will a future AI winter happen?
In the past decade, AI has been on a strong upswing. Some of the main advancements of artificial intelligence that have fueled the hype include deep learning, graphics processing units and big data analytics and processing. Some other real-world landmark areas of innovation include the following:
- facial recognition;
- machine learning algorithms;
- data science and analytics;
- the AI agent AlphaGo for the Chinese boardgame Go;
- advanced language recognition and translation;
- medical diagnoses and imaging; and
- self-driving cars.
Although these advancements have been influential, they also have significant limitations that prevent broad applicability and ubiquitous, cross-contextual use. For example, facial recognition deals with ethical challenges in certain contexts. Also, self-driving cars aren't capable of driving with the sophistication of human drivers and are still prone to accidents due to flaws in object recognition.
AI still has significant obstacles to overcome before becoming an integral, everyday technology. Current applications of artificial intelligence excel at solving certain specific problems and require a lot of data to do so. To achieve artificial general intelligence -- dubbed the Holy Grail of artificial intelligence -- AI will have to improve at solving a wider array of problems with significantly less data. These limitations are the reason that, after several years of hype, advances and implementations, some analysts are predicting another AI winter. Still others remain optimistic, as AI continues to automate business functions in what some have dubbed the Autonomous Revolution.
AI summers
An AI summer represents a point in time when interest and funding for AI is booming and an increase in funding is devoted to the development and application of AI technology. Despite the limitations of AI, many believe the industry is in an AI summer. During AI summers, lofty expectations are set due to technological breakthroughs, promises are made about the future of AI and the market invests in them.
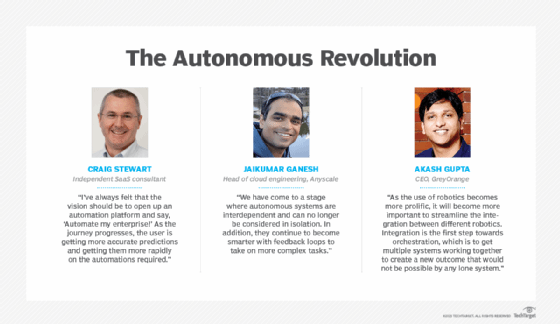
At every point in the hype cycles of optimism and disillusionment that define the public perceptions of AI technology, there still remain a range of challenges. Ethics is a hot-button topic of discussion for AI and the IT industry in general. Consumers and tech industry workers are raising questions about how automated decision-making systems are designed and what decisions they should be allowed to make, both in terms of industry verticals and specific applications within them. This is an issue in the medical industry, for example, where emergent medical data can be used to gather medical information from an individual's seemingly unrelated behavior patterns.
Despite doubts, limitations and pessimism, the enterprise AI industry is here to stay. Learn how to implement, maintain and develop it using this complete guide.