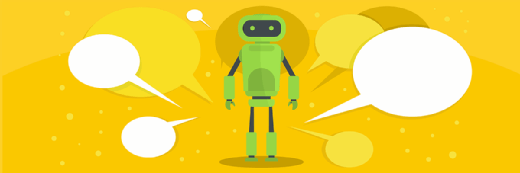
Building trustworthy AI is key for enterprises
Organizations need to focus on transparency in models, ethical procedures and responsible AI in order to best comply with guidelines for developing trustworthy AI systems.
In April 2019, the European Union released a set of guidelines for developing trustworthy AI systems. However, enterprises are just starting to realize ROI with AI applications, and the movement to make these systems ethical and responsible is still nascent.
The core of these guidelines requires applications that use artificial intelligence capabilities be "lawful, ethical and robust." Setting these guidelines in place within your organization requires significant time but has become an important part of AI adoption. Without consumer trust and proper guidelines, AI applications cannot reach their potential in the enterprise.
Real-world AI and the need for trust
One of the challenges with the pursuit of AI is the mismatch between the science fiction concept of artificial intelligence and the real-world, practical applications of AI. In movies and science fiction novels, AI systems are portrayed as super-intelligent machines that have cognitive capabilities equal to or greater than that of humans.
However, the reality is that much of what organizations are implementing today for artificial intelligence are narrow applications of AI. This is in clear contrast to artificial general intelligence (AGI). The limit of our current AI abilities lets organizations implement specific cognitive abilities in narrow domains, such as image recognition, conversational systems, predictive analytics as well as pattern and anomaly detection.
In each of these domains, we are asking machines to perform a specific set of tasks that would otherwise require the judgment, insight or innate cognitive ability of humans. Yet, even in these narrow applications of AI, we have reason to be concerned. Algorithms are put into a position where they make an impact on someone's life, job and health. Organizational application of artificial intelligence places the algorithm in a position of responsibility, and this comes with risk and a need for trust.
Transparency, ethics and responsibility
Wrapped up in this idea of trustworthiness are the concepts of transparency, ethics and responsible AI. The first concept is that of transparency. Humans need to have visibility into how the AI comes to its decisions as well as what data it uses. Without visibility, it's impossible to understand and dissect the reasons behind AI decisions if something goes wrong. Transparency gives people the opportunity to improve their systems by having visibility into how they fail or where they make mistakes. Transparency is more than just a nice-to-have, feel-good feature; it's necessary for overall system viability.
Separate from the issue of transparency is the issue of ethics. Even if we know how the system is working, it is important to know that the actions are ethical. Companies are using algorithmic decision-making that has been shown to be prone to bias. These biases can then become entrenched in the systems if there isn't sufficient human oversight. Applications such as facial recognition have run into challenges with accuracy and the tendency for organizations to put too much emphasis on what is a probabilistic match. The question here is not about the system's functionality or transparency, but rather is about the way in which the AI is being used.
Related to the issue of ethics is the concept of responsible AI. Even if the systems are transparent and we're operating them ethically, it is important for organizations to ensure that any outcomes are handled responsibly. If these systems hold important decisions in the balance, then oversight by employees is key. While these systems might seem ethical when taken at face value, they need aspects of responsibility to make them trustworthy.
What is considered trustworthy AI?
The core of the trustworthy AI recommendations that the European Union released in 2019 can be split into three parts. Trustworthy AI systems are:
- Lawful. Those that respect all laws and regulations.
- Ethical. Those that respect principles and values.
- Robust. These AI applications take both a technical and a social environment perspective into consideration with regards to system behavior.
To implement these three core parts, the EU trustworthy AI recommendations list seven requirements for the AI system to be considered trustworthy. These requirements apply to all those who are involved in planning, developing and managing the AI systems. This is a long list that includes developers, data scientists, project managers, line-of-business owners and even the users of the applications. The core requirements are:
- Focus on human agency and oversight. AI systems need to support human objectives, enable people to flourish, support human agency and fundamental rights and support overall goals of a healthy society.
- Technical robustness and safety. AI systems should "do no harm" and even prevent harm from occurring. They must be developed to perform reliably, have safe failover mechanisms, minimize intentional as well as unintentional harm and prevent damage to people or systems.
- Privacy and data governance. AI systems should maintain people's data privacy as well as the privacy of the models and supporting systems.
- Transparency. AI systems should be able to explain their decision-making as well as provide visibility into all elements of the system.
- Diversity, nondiscrimination and fairness. As part of the focus on human agency and rights, AI systems must support society's goals of inclusion and diversity, minimize aspects of bias and treat humans with equity.
- Societal and environmental well-being. In general, the AI applications shouldn't cause societal or environmental unrest, make people feel like they're losing control of their lives or jobs or work to destabilize the world.
- Accountability. At the end of the day, someone needs to be in charge. The systems might be working in an autonomous fashion, but humans should be the supervisors of the machine. There needs to be an established path for responsibility and accountability for the behavior and operation of the AI system through the system's lifecycle.
Building AI systems we can trust: a human-centered approach
Many of the goals outlined above can seem lofty from the perspective of enterprise adopters. To make these recommendations more practical, those looking to build AI systems in a trustworthy manner should look at each of the above objectives and ask how those overall goals fit their specific needs.
Some ways in which enterprises can put the EU trustworthy AI recommendations into practice include:
- Maintain data privacy and security. Look across the AI system lifecycle and make sure that portions that interact with data, metadata and models are secured and maintain data privacy as required.
- Reduce the bias of data sets to train AI models. Examine training data sets for sources of potential bias and make sure that communities are represented in a fair and equitable way.
- Provide transparency into AI and data usage. Organizations should let AI system users know how their data is being used to train or power AI systems and provide visibility into aspects of data selection, usage and even the business model that the AI system supports. To the extent that the AI system might be invisible to the user, responsible AI usage suggests you should let your users know they are interacting with an AI-based system.
- Keep the human in the loop. Even when AI systems are operating in an autonomous fashion, there should always be a person keeping an eye on system performance. There should be an appointed human system owner or group of people who are responsible. Users should also know who to reach out to when the AI systems are exhibiting problematic behaviors.
- Limit the impact of AI systems on critical decision-making. If the AI system is being used for critical life-or-death or high-impact decisions, there should always be an identified failover process or human oversight to make sure that no harm is done.
Applying the above guidelines gives users more confidence in the AI system and allows the AI to deliver the expected value without any fear of irresponsible behavior or outcomes.
At the end of the day, the concept of trustworthiness is all about humans putting their confidence in machine-based systems. Trust is hard-won and it is vitally important for those looking to put AI into real-world use to pay close attention to these issues of trustworthiness and responsibility. As AI increasingly becomes a part of our daily lives, that trustworthiness will make the difference between AI systems that are relied upon and those that are shuttered due to legitimate concerns or individual fears.