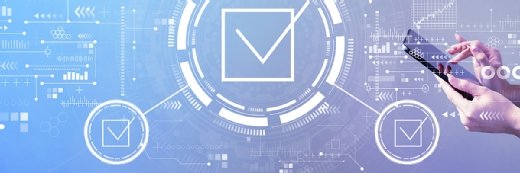
Getty Images/iStockphoto
What are the risks and limitations of generative AI?
As enterprise adoption grows, it's crucial for organizations to build frameworks that address generative AI's limitations and risks, such as model drift, hallucinations and bias.
Generative AI tools are moving into the enterprise technology portfolio at a breakneck pace as organizations race to jump on the bandwagon. But many organizations are ill prepared to cope with the limitations and risks associated with using generative AI.
A Nemertes enterprise AI research study for 2023-24 found that more than 60% of participating organizations were already using AI in some production capacity and nearly 80% were using it in their lines of business. Yet fewer than 36% had a full policy framework guiding their generative AI use.
Understanding the limitations of generative AI tools
Two significant and interlocked generative AI limitations are scope and confabulation.
To be as flexible and effective as possible, developers often build generative AI tools around large language models. This is because larger models with more parameters tend to be more powerful, thanks to their ability to capture more complex relationships and patterns.
Unfortunately, LLMs large enough to provide ChatGPT-style power are also prone to hallucinations -- the phenomenon of a model unpredictably making up information when the user's prompt implies a desire for accurate information. For example, when a lawyer asks a generative AI tool for precedent relevant to a point of law, they do not expect the output to cite fictional cases. But limiting a model's power to the extent that it can no longer provide creative responses makes it less useful overall.
Scale and longevity are also problems for those developing their own AI models instead of using commercially available offerings. Developing a powerful LLM-based AI tool can require millions of dollars' worth of hardware and power.
Once built, such tools require either periodic retraining -- which adds to the resource expense -- or the ability to autonomously learn and self-update. Active learning to adjust the model opens up the risks of the model drifting out of its tuned state into something less useful or downright dangerous, such as becoming irreparably biased or prone to hallucinations.
AI bias can be a subtle problem. Bias embedded in the training inputs, initial training, retraining or active learning can lead to bias in the outputs. These biases can exist in the training data itself, such as text reflecting sexist or racist norms, or in layers of tagging and manipulation of input data that guide a model's learning to reflect the trainer's biases. For example, biases in models trained to evaluate loan applications and resumes have resulted in race- and gender-based discrimination.
Gaps in reasoning are another significant limitation of AI models and can become harder to identify as models begin to produce higher-quality output. For example, a tool designed to create recipes for a grocery store chain generated obviously toxic ingredient combinations. Although most people would be suspicious of a recipe called "bleach-infused rice surprise," some users -- such as children -- might not realize the danger. Likewise, a less obvious toxic ingredient combination could have led to a disastrous rather than amusing outcome.
3 types of potential generative AI risks
Generative AI risks fall into several broad categories: functional, operational and legal.
1. Functional risks
Functional risks threaten the continued utility of an organization's AI tools. Two key functional risks are model drift and data poisoning.
Model drift happens when a model gradually loses alignment with the space in which it was trained to help. To resolve this problem, the model must be retrained on refreshed data, a process that can be costly and time-consuming.
Data poisoning occurs when a bad actor, such as a commercial competitor or a hostile nation-state, corrupts the data stream used to train a model. Adversaries might poison input for a pre-released training cycle or a model that uses production data input to self-modify.
2. Operational risks
Operational risks are those that might hurt a company's ability to function.
In part, the risks associated with following incorrect AI-generated advice or using the output of a poisoned model stem from the misdirection and resulting waste of resources. There is also the issue of wasting resources that could otherwise have been put toward sounder strategies, resulting in missed opportunities.
Another risk is the unwanted disclosure of confidential intellectual property. Through careful prompt engineering, malicious actors could lead generative AI tools to disclose sensitive information. Leaks of this sort can undercut competitive advantages and reveal trade secrets.
3. Legal risks
Legal risks occur when the use of generative AI exposes an organization to civil and criminal actions.
These legal risks can arise from confabulation -- for example, if a consumer is harmed by false information that an organization's AI tool provides. Biases in AI tools evaluating documents such as loan applications and resumes can expose the companies involved to penalties, fines and lawsuits, as well as reputational damage.
Copyright infringement is another risk for those using generative AI tools. An LLM's training data can include copyrighted works, and whether responses that draw on that data are considered copyright infringement is still an open question. In a similar vein, generative AI tools that disclose personally identifiable information could expose organizations to lawsuits, penalties and reputational damage.
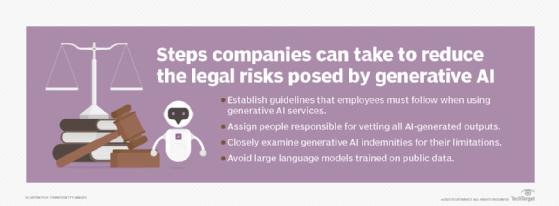
Generative AI risk mitigation policies and best practices
The best first step to mitigate generative AI risks is to develop and adhere to a well-defined machine learning operations lifecycle; that MLOps lifecycle should be embedded in a broader governance framework within the boundaries of which an organization develops and uses AI. Enterprises should involve not just IT teams in creating policies, but also cybersecurity, legal, risk management, and HR leaders and specialists.
Long-term awareness is the best mitigation. Organizations should regularly revisit their AI policy framework and conduct tabletop exercises to stress-test it. By working through scenarios involving potential problems and how to respond to them, organizations can be sure everyone is aware of the potential problems, as well as what AI-related policies exist and why.
John Burke is CTO and principal research analyst with Nemertes Research. With nearly two decades of technology experience, he has worked at all levels of IT, including end-user support specialist, programmer, system administrator, database specialist, network administrator, network architect and systems architect. His focus areas include AI, cloud, networking, infrastructure, automation and cybersecurity.