How AI is transforming the talent acquisition process
Machine learning, natural language processing and RPA can source candidates faster, match their skills better and vet them with less bias. An HR technology expert explains how.
It's old news that locating, hiring and retaining top talent is HR's No. 1 challenge. However, the vast changes stemming from the pandemic's impact on remote work and many people's reassessment of their personal life choices, not to mention the "great resignation," have exacerbated these perennial challenges. Systemic issues such as the dearth of child and family care with its outsized effect on the employment of women, the ongoing toll of COVID variants on workforce participation and productivity, the continual stress on healthcare professionals, and the unknown long-term effects of COVID and monkeypox present further complications.
All is not bleak for HR teams facing employee volatility, though, as advancements in AI have increasingly been applied to talent acquisition and other parts of HR. While talent acquisition vendors tentatively poked their toes into AI pre-pandemic, they made use of the time to create "smart" deep-learning platforms that underlie their cloud talent cloud applications. Today, AI is ubiquitous in products for sourcing, hiring and managing employees.
But what has changed and why does it matter?
What is artificial intelligence?
First, AI is not a product, a "thing" that an HRIS professional would go procure. It is, rather, an underlying platform or infrastructure on which specific products such as a recruitment system are built.
Implemented in software, an AI platform consists of a variety of technologies, including machine learning, natural language processing (NLP), predictive analytics and robotic process automation (see the sidebars for deeper dives into the last three). Collectively described as an "engine," AI can support functions across the enterprise, creating efficiencies in supply chain management, customer relationships, product production and delivery, and business planning, to name a few. Now, AI is increasingly making inroads in HR processes and employee management -- specifically in easing issues in sourcing, hiring, onboarding, learning and the overall employee lifecycle.
This article is part of
Ultimate guide to recruitment and talent acquisition
An AI engine analyzes data -- ordinarily an immense amount of it -- then decides or recommends what should happen next to complete a task. Some products use multiple engines to improve reliability. The data could be in resumes, employee profiles, patient records, images like MRIs and X-rays, production schedules, employee promotion rates -- basically anything.
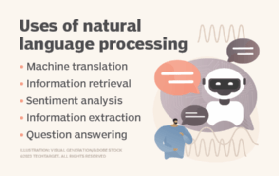
For example, iCIMS, a provider of talent management software, uses an ensemble AI methodology that learns from employer and job-seeker activity over more than 4,000 customers and hundreds of millions of data points across job openings, applications and hires.
The benefits are twofold: Not only can AI-powered systems deliver expected information faster and more efficiently, they can provide insight into areas that otherwise may not be obvious. The ability of the application to use machine learning to continually "learn" and adapt is a fundamental aspect of any AI program.
What is natural language processing?
Natural language processing is a key capability of AI. Consider the vast differences and nuances in written and oral language, and the varied ways humans ask questions and supply answers -- then multiply that across languages and dialects. Now add the computational power of today's machines that learn to understand text and spoken words and respond like people do.
A ready example is Amazon Alexa. Consider the following questions:
- What is the temperature today?
- How hot is it outside?
- What's the temp?
All are variations of the same question that the AI is likely to respond to with the same correct answer: "It is 86 degrees Fahrenheit outside now."
Machines are getting smarter about nuances and better at assessing a speaker's intent or sentiment. Understanding subtleties of sarcasm or irony may be beyond what's necessary in evaluating resumes or applications, but it may have a place in video interviews.
How can AI be used in talent acquisition?
Here are 10 areas in which AI can benefit the talent acquisition process.
1. Job descriptions
Recruiters can't be expected to know everything about the nuances of job requisition language, especially new recruiters or recruiters for highly technical positions. What are the keywords in a given field that attract the most qualified responses? Which words unintentionally signal gender preference? This is where AI comes into play. Competitive talent acquisition products for several years have deployed intelligence that reviews linguistic choices and suggests alternatives. For example, if a recruiter is writing a req for a welder, and in their experience, all welders were male, the language may be unintentionally biased toward male applicants. The AI engine shouldn't have the same bias. As in all cases, it is necessary to apply a human eye to the results because if the AI data set consists only of male welders, the engine, looking at features that tend to surround welding skill, might conclude erroneously that other male traits are essential to being a proficient welder.
2. Job postings
CareerBuilder claims that its AI helps recruiters create job postings five times faster. The platform provides a candidate appeal score to help recruiters gauge how effective a post will be in attracting applicants, increasing applications by 175%, according to the company. A veteran in the hiring industry, CareerBuilder says its AI learns from data from more than 2.3 million job postings, 680 million unique profiles, 310 million resumes, 10 million job titles and 1.3 billion skills.
Recent AI tools can predict the salary range from a job posting that doesn't include salary data, potentially giving applicants more interview ammunition than recruiters intended.
3. Candidate sourcing
Starting with the wording of requirements in the job description, the AI engine can expand the search beyond the exact wording in the job post to related industry-specific language and terms. Unlike a simple word-matching algorithm, AI-enabled sourcing provides a semantic search by intent or meaning.
In addition, through the deep learning that permeates AI systems, the concept of skills adjacency is addressed. If a candidate knows how to do a certain thing, the software can infer the prerequisites of that skill or knowledge and determine the likelihood of the person having a similar but different skill, based on analysis of millions of data points. Candidate sourcing is thereby facilitated with a wider, more inclusive pool of candidates that humans may miss, and over time can answer such questions as the following:
- What are the best sources of high-performing candidates who will stay on the job for more than two years?
- What are the best sources of women who have been promoted into management positions?
- What sources are yielding healthcare workers who can fill the position on day one?
When sourcing candidates or, later, evaluating them, AI has the ability to infer skills or interests from, for example, prior jobs held, volunteer positions or education. Because the program is always learning, it retains associations it can then apply in different recruitment scenarios. This can increase the possible positions within an organization for which the candidate or a current employee may be suited, even if they did not actually apply for them.
What is predictive analytics?
Predictive analytics uses predictive modeling to analyze patterns in data and create forecasts. Because AI engines can be fed big data -- millions and millions of data points -- they can report patterns that humans might take years to perceive. They can generate models to provide insights for business decisions and predict anomalies, for example, which is useful in product quality control and demand forecasting. Devoid of emotion, the engine can expose patterns and correlations that humans may miss or prefer to overlook. Prior history is only one input -- few in business want to recreate the past -- but combined with current data on trends and outcomes, AI-based predictive analytics can provide deep, relevant insights on which to base decisions and actions.
4. Applicant screening
In candidate screening, as in sourcing, the kinds of data from which a machine learning program can learn rules and patterns may be varied and include past or current employee resumes; competency or skill models that are tied to the specific industry or task; incoming resumes or records of employee success or longevity based on skills, education or even location. It may also include art or design portfolios, physical objects or electronic samples such as videos and games. External data sources such as national or international salary ranges, U.S. Bureau of Labor Statistics job classifications, etc., can also be fed into the "brain" that is AI. Machine learning can then help provide answers to questions such as "What candidate traits correlate with long-term success in this position?" Some firms use AI-powered games or video games to ascertain the fit between the candidate's personality or working preferences and the position.
Because candidates often want to apply to a company rather than a specific job, AI can recommend positions that are relevant to the skill set, education or soft skills of each candidate. It is also useful for recommending new positions or opportunities for current employees based on their skills, interests and aspirations.
This is a great advantage to both applicants and hiring companies. For example, a college graduate who might want to work for Microsoft can submit their resume and the AI-based talent acquisition tools can recommend the jobs for which they may be a likely candidate. In another scenario, if a non-degreed applicant is a gamer that a recruiter might reject out of hand (or perhaps never see their application at all), AI can look at non-traditional skills such as gaming and social media as applicable to a particular position. It is a huge time-saver for recruiters, hiring managers and candidates alike.
5. Skill assessment
Deep learning generally implies the use of vast neural networks, such as those Eightfold.ai uses: billions of profiles and global data points and over 1 million unique skills to provide data-driven insights about skills. With the goal of understanding an applicant's potential, Eightfold.ai helps companies identify the prevalence of capabilities on a global scale so they can find talent in particular locations. The software assesses a candidate's validated skills, likely adjacent skills, those that remain to be validated and missing skills. It's particularly important for reskilling for the AI to identify capability gaps in the workforce and find people with the best potential to fill them.
6. Bias mitigation
Many vendors employ AI in talent acquisition applications to alleviate bias in hiring and further diversity instead of perpetuating homogeneity in race, ethnicity, gender, gender preference, color, etc. An unbiased application can help eliminate bias arising from other characteristics that lead to discrimination, such as regional accents, perceived social standing and parental status. An AI tool is not going to be overly impressed with a beautiful resume layout or underwhelmed if a resume has a typo -- two things that can unconsciously influence human decisions.
Such smart programs evaluate job requisitions, postings and other written communications for gender-laden language. However, not all products purporting to use AI for talent acquisition are the same. If a company only uses its own historical data -- which is by definition limited -- biased output is likely to result. Successful applications that embed AI have access to deep-learning neural networks of external data with which to complement internal data sets.
Even so, recruiters have to be diligent in reviewing AI recommendations, as systemic bias in the past is not alleviated solely by using vast numbers of records. More than a few organizations have realized that the historical preponderance of white males still colors the AI-generated suggestions.
7. Online interviewing
In video interviews, AI can catch subtleties such as gestures and facial expressions that humans might not pay attention to and miss. Conversely, the tool can purposefully overlook mannerisms that the human interviewer may find distracting but which wouldn't impede the candidate's success in the job. Similarly, because AI can analyze huge volumes of data without fatigue, patterns may well emerge that would otherwise be overlooked by busy recruiters and hiring managers. It is this ability to make sense or find correlations in masses of information that makes AI such a valuable tool.
Not to be ignored is the ability of AI tools to look across calendars and manage interview scheduling, often one of the greatest sources of annoyance in the hiring process. Scheduling via AI can be less painful and more rewarding for everyone.
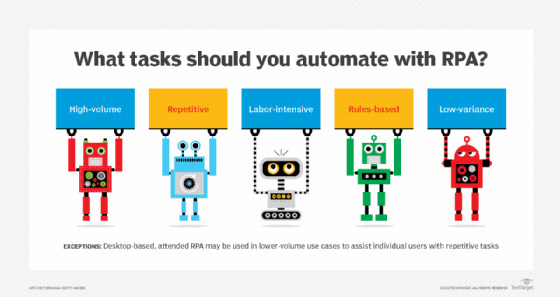
8. Candidate experience
Robotic process automation (RPA) uses bots -- think Amazon Alexa or Apple Siri -- to replicate human actions in handling time-consuming questions and tasks. The increasingly widespread use of these natural language chatbots, some of which can understand and respond to both written and spoken input, is becoming de rigueur in human capital management software. Today's users are accustomed to bots as sources of information and direction and are used to interacting in natural language with bots in online and telephone transactions. Some recent studies show that younger people prefer interacting with a bot than a real person.
Hiring management platforms use chatbots to answer candidates' questions, guide them in timely choices and actions, and keep them informed of their status, while letting them know about new opportunities that might be good fits. In HR, bots are noted for their value in handling the repetitive questions employees tend to ask, such as "What holidays does the company take off? How do I get proof of employment?" As the number of people accustomed to chatbots increases, and as the bots get smarter, chatbot use lessens the number of escalations to humans that are required. In fact, Gartner anticipates that all future customer service will be conducted by bots. The same is likely true of candidate management.
Judicious use of bots in talent acquisition is an advantage because bots can respond to a candidate immediately and often in personalized ways. In response to the pervasive reliance on text messaging in recruiting conversations, tools such as HireVue and iCIMS' text engagement tools use NLP and machine learning to enable dual-conversation live chat and initiate intelligent text-based discussions, both of which are easy and convenient for the candidate. These applications understand people's natural inputs and ultimately improve feedback and predictions by learning over time. Their conversational interfaces can improve candidate engagement and personalize responses, and obviously, delivering bot-supplied answers in real time minimizes the need for human intervention. That saves time for the recruiter, the hiring manager and the candidate.
With intelligent bots, candidates often cannot tell they aren't dealing with a human. Today's smart bots, often referred to as digital assistants, can schedule interviews, respond in any language, walk candidates through video or text processes and provide encouragement if they get stuck in the application process. They are useful for career site chat, when a passive job seeker is just browsing a site, by calling attention to jobs of interest. The bot can embed employee video testimonials to further attract the potential applicant's interest.
What is RPA?
The word "robot" usually brings up images of Star Wars characters R2D2, C-3PO or the smooth-voiced Hal of 2001: A Space Odyssey. Today's factories and warehouses are heavily automated with robots used in production, picking and packing, and delivery. My personal favorite robot is a natural-language-aware barista in San Francisco that makes perfect lattes and cappuccinos without human intervention.
More commonly, though, RPA refers to software robots that handle repetitive and rule-based tasks. It generally uses structured data, and humans define the logic and rules. Today, such bots are everywhere on websites from banking to airlines, providing customer and candidate self-service. More sophisticated bots are likely to dominate all service in the future and human intervention or escalation will rarely be needed.
9. Economic advantages
The efficiency and speed of AI-enabled talent acquisition applications have positive cost benefits in that the AI engine never tires or takes a day off. CareerBuilder, for one, reports that its talent acquisition software has reduced the cost per candidate by as much as 50% since the product was rebuilt to employ AI. Chatbots allow recruiters to focus their talents in the bottom of the applicant funnel, a productivity boon. More expedient hiring, including improved time-to-fill and time-to-hire metrics, brings real cost savings to an organization. An empty seat is always an expensive proposition, and delays in responding to applicants is a sure way to lose them.
10. Onboarding
Employees feel most positive about their new position on the day they get the offer letter. Unfortunately, that high level of engagement can decline between offer acceptance and the first day on the job. Here is another major role for AI and bot-initiated communication. First, AI "knows" the forms that are necessary for what jobs in which locations, ensuring that the new hire gets the appropriate ones. Because all this can be done online with digital signatures, the tasks can be completed long before the first day at work. AI can also tell if the forms are complete and correct by spotting deviations in responses about age, Social Security number, addresses and the like and automatically requesting data verification or reentry.
As important, if not more, is the welcoming information for the new candidate. The hiring management program knows what location the person is working at or if they are working from home. Site-map simulations can walk the new hire through the building, showing them where to go on day one, where their office or cube is, where the cafeteria and restrooms are and where to get a badge or other ID. AI can automate requisition of the right materials and ensure that business cards are on the new employee's desk on the first day, a proven engagement technique. Bots with employee videos can introduce the new hire to other team members, all in the first few days after offer acceptance. Bots can answer FAQs of new hires, saving HR time and easing the employee's anxiety. Nevertheless, the welcome from the hiring manager, one would hope, is always real person to real person.
Summary
AI is ubiquitous in the new talent acquisition applications hitting the market. That it will make sourcing, screening and hiring easier and more efficient is clear. AI's applicability in HR and in all of business will be widespread, and organizations will be well served to create governance committees to oversee its use.
Because AI technology is in effect recommending, making choices and predicting, human vigilance remains necessary to ensure relevant data is being fed to the engine and that the output makes sense for the organization. Cathy O'Neil, author and data scientist, makes a compelling point in discussing the use of AI in decision-making: "Algorithms are opinions embedded in code." Those opinions are only good when they relate to the requirements of your business.